Models for COVID-19 Data Prediction Based on Improved LSTM-ARIMA Algorithms
IEEE ACCESS(2024)
摘要
The global repercussions of the COVID-19 pandemic on economies and public health worldwide have been profound. This study aims to examine the developmental trends of the COVID-19 pandemic, establish predictive models, and provide insights for effective control measures against potential future disease outbreaks. Considering the coexistence of both linear and nonlinear factors in COVID-19 data, conventional single-machine learning and traditional forecasting models encounter challenges in accurately predicting pandemic trends. To enhance the precision of COVID-19 pandemic predictions by integrating linear and nonlinear factors, this study proposes three combined forecasting models: CNN-LSTM-ARIMA, TCN-LSTM-ARIMA, and SSA-LSTM-ARIMA. These models leverage the strengths of deep learning in capturing nonlinear factors and the capabilities of the traditional ARIMA model in handling linear factors. Initially, LSTM and ARIMA models are used to model and predict the COVID-19 pandemic in Quebec, Canada. Subsequently, CNN models, TCN models, and the Sparrow Search Algorithm are employed to integrate predictions from the LSTM and ARIMA models. Comparative analyses of the three combined models, it was found that the CNN-LSTM-ARIMA model exhibits the highest predictive accuracy, with an MSE of 7048.26, RMSE of 83.95, MAE of 61.18, MAPE of 0.16, and R-2 of 0.95. To validate the applicability and stability of the CNN-LSTM-ARIMA model in predicting COVID-19 pandemics, Italian COVID-19 pandemic data was employed. The three combined forecasting models are established and evaluated using model evaluation metrics. The results affirm that the CNN-LSTM-ARIMA model remains the optimal choice, underscoring its high stability and suitability for COVID-19 pandemic forecasting endeavors.
更多查看译文
关键词
COVID-19,LSTM,ARIMA,CNN-LSTM-ARIMA,TCN-LSTM-ARIMA,SSA-LSTM-ARIMA
AI 理解论文
溯源树
样例
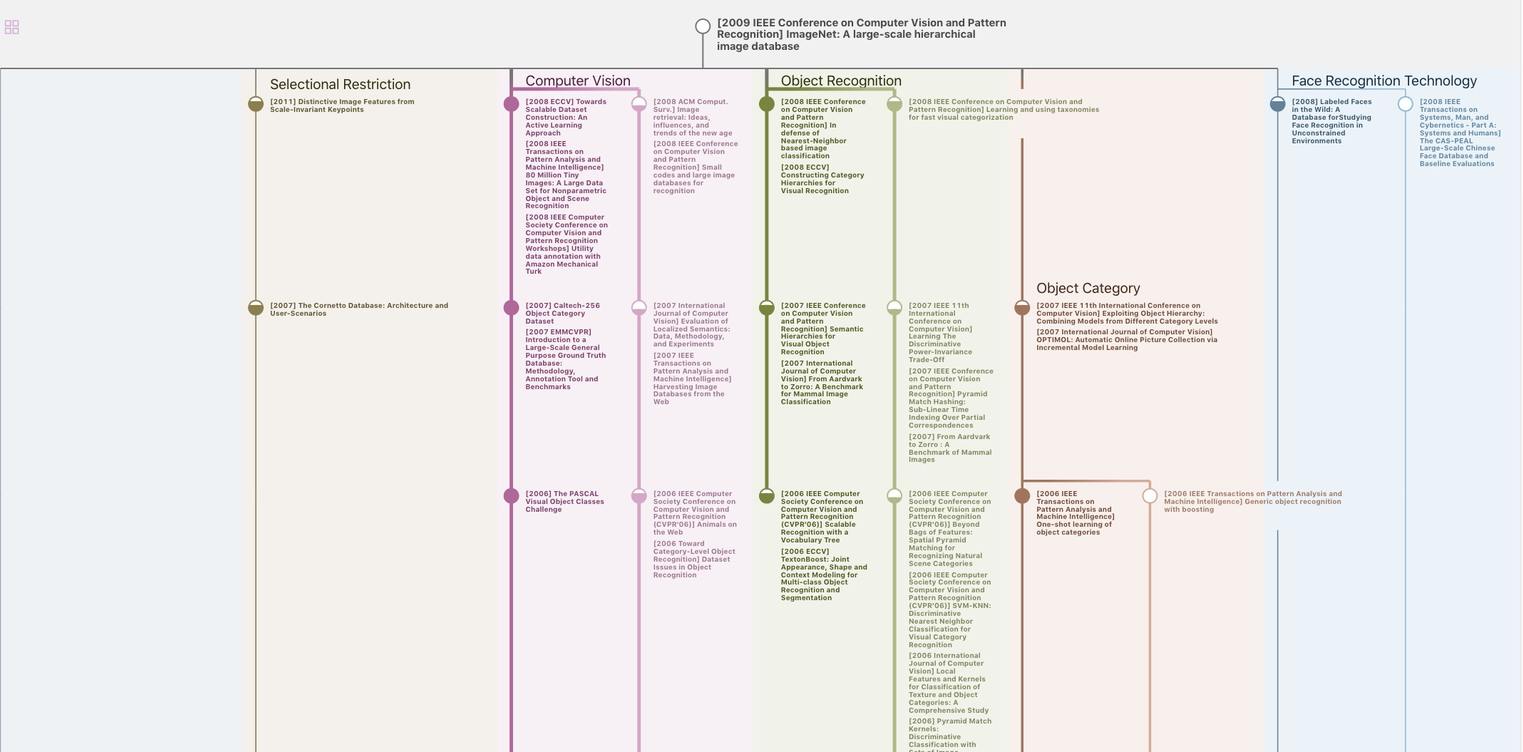
生成溯源树,研究论文发展脉络
Chat Paper
正在生成论文摘要