Improve the efficiency of handcrafted features in image retrieval by adding selected feature generating layers of deep convolutional neural networks
Signal, Image and Video Processing(2024)
摘要
Today, with the rapid growth of communication technology and the development of social networks and smartphones, the amount of data stored by users in the form of images has significantly increased. Therefore, several researchers have focused on the field of image retrieval in the past decade. Image retrieval means retrieving the most similar images to the query sample from a vast image database in terms of content and semantic. So far, various image retrieval methods based on feature engineering or deep features have been proposed. Most methods that use handcrafted features, despite their lower runtime, usually provide lower performance than deep learning-based approaches. In this paper, to increase the efficiency of handcrafted features in image retrieval, their combination with the output of residual blocks in deep neural networks is used in the feature fusion phase. In this paper, the efficiency of feature generation layers in common deep networks such as residual, classical sequential convolution and bottleneck has been analyzed in order to increase the efficiency of image retrieval systems based on handcrafted features. For this purpose, in most popular deep networks, the layers that perform the classification task are removed, and the output of feature generation layers at different depths, with the help of a flattened layer, is converted to feature vectors that can be connected to handcrafted made numerical features in retrieval systems. A variety of popular handcrafted features in the spatial domain, such as color and texture and wavelet in frequency, are employed to generate handcrafted features. This paper presents a novel hybrid feature set based on a combination of classical feature engineering techniques and deep convolutional neural networks suitable for image retrieval. The efficiency of the proposed method is evaluated in terms of precision and recall metrics on the benchmark datasets, such as Corel-1 and -5k. The proposed method provided an accuracy of 96.68 and 94.56% on the Corel-1k and Corel-5k, respectively. The comparative results showed that combining residual block feature maps with handcrafted features compared to bottleneck and sequential classical convolution layers increases the final performance of the image retrieval system. The comparison of the proposed method with state-of-the-art machine learning and deep learning-based techniques shows that the proposed method provides promising results in terms of precision and recall rates.
更多查看译文
关键词
Image retrieval,Feature extraction,Deep learning,Texture analysis,Residual neural network
AI 理解论文
溯源树
样例
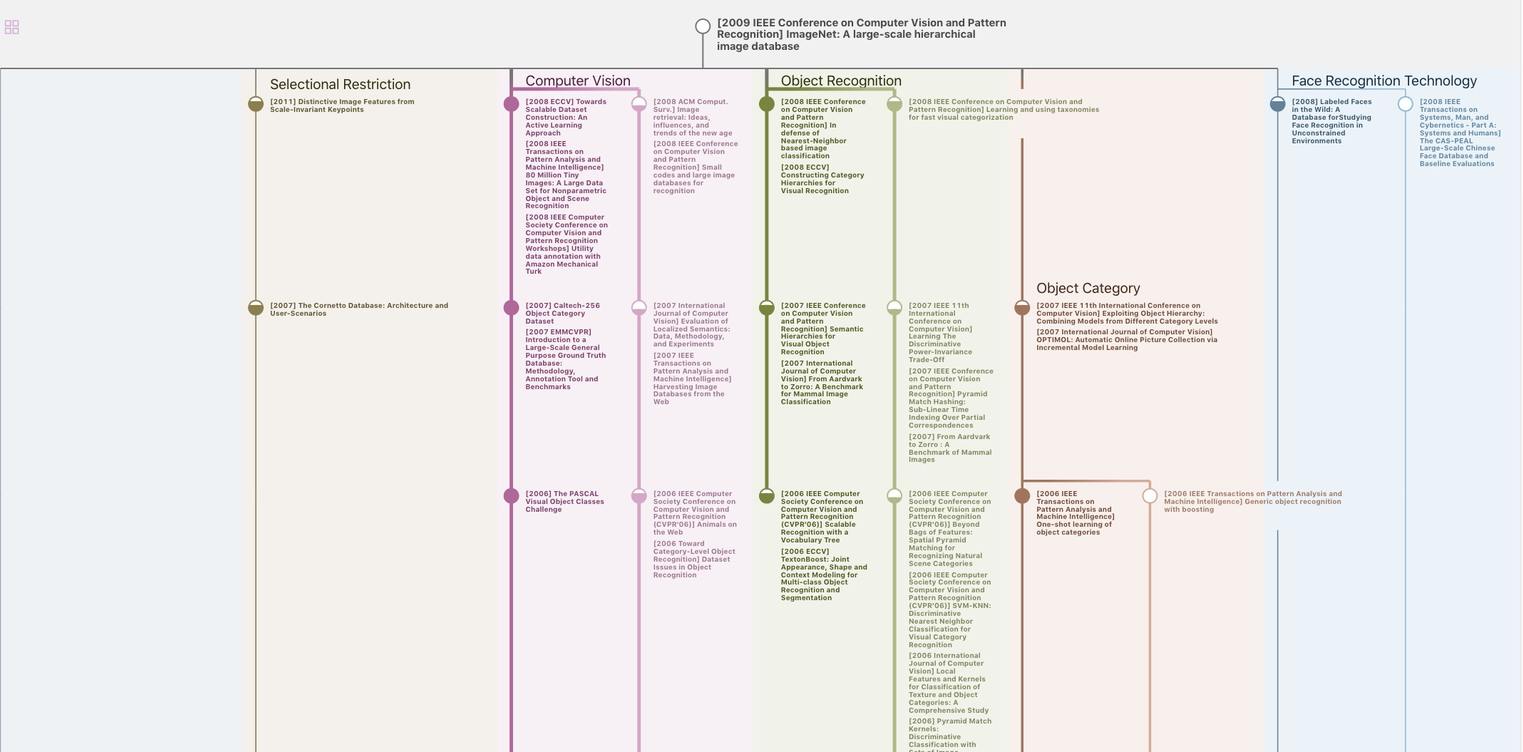
生成溯源树,研究论文发展脉络
Chat Paper
正在生成论文摘要