Multimodal Informative ViT: Information Aggregation and Distribution for Hyperspectral and LiDAR Classification
CoRR(2024)
摘要
In multimodal land cover classification (MLCC), a common challenge is the
redundancy in data distribution, where irrelevant information from multiple
modalities can hinder the effective integration of their unique features. To
tackle this, we introduce the Multimodal Informative Vit (MIVit), a system with
an innovative information aggregate-distributing mechanism. This approach
redefines redundancy levels and integrates performance-aware elements into the
fused representation, facilitating the learning of semantics in both forward
and backward directions. MIVit stands out by significantly reducing redundancy
in the empirical distribution of each modality's separate and fused features.
It employs oriented attention fusion (OAF) for extracting shallow local
features across modalities in horizontal and vertical dimensions, and a
Transformer feature extractor for extracting deep global features through
long-range attention. We also propose an information aggregation constraint
(IAC) based on mutual information, designed to remove redundant information and
preserve complementary information within embedded features. Additionally, the
information distribution flow (IDF) in MIVit enhances performance-awareness by
distributing global classification information across different modalities'
feature maps. This architecture also addresses missing modality challenges with
lightweight independent modality classifiers, reducing the computational load
typically associated with Transformers. Our results show that MIVit's
bidirectional aggregate-distributing mechanism between modalities is highly
effective, achieving an average overall accuracy of 95.56
multimodal datasets. This performance surpasses current state-of-the-art
methods in MLCC. The code for MIVit is accessible at
https://github.com/icey-zhang/MIViT.
更多查看译文
关键词
Hyperspectral image,multimodal fusion,land cover classification,mutual information,self-distillation
AI 理解论文
溯源树
样例
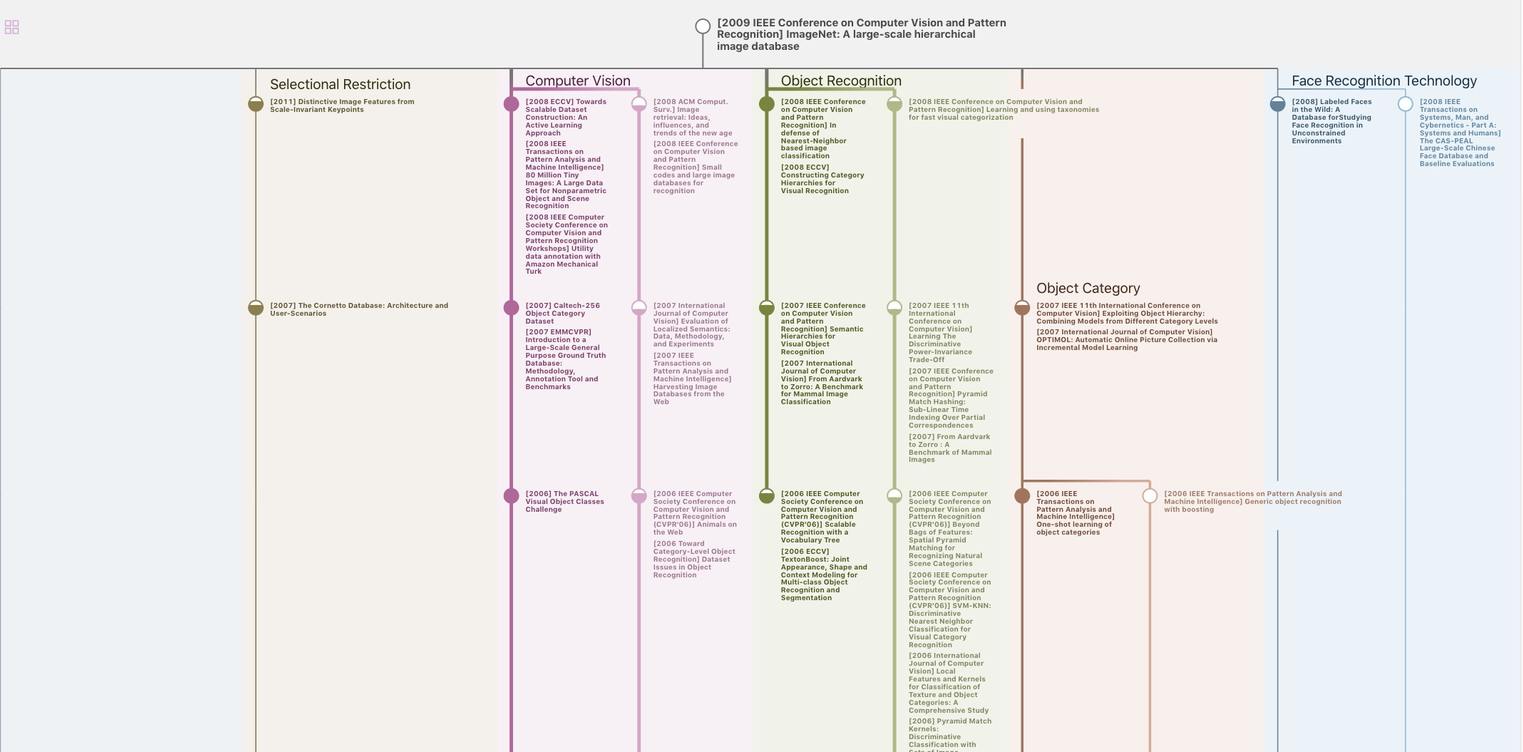
生成溯源树,研究论文发展脉络
Chat Paper
正在生成论文摘要