Residual Relation-Aware Attention Deep Graph-Recurrent Model for Emotion Recognition in Conversation
IEEE ACCESS(2024)
摘要
This work addresses Emotion Recognition in Conversation (ERC), a task with substantial implications for the classification of the underlying emotions in spoken encounters. Our focus is on utilizing a fully connected directed acyclic graph to represent conversations, presenting inter-locutor and intra-locutor ties to capture intricate relationships. Therefore, we propose a novel methodology, Residual Relation-Aware Attention (RRAA) with Positional Encoding, enhancing speaker relations' contexts for improved emotion recognition in conversation. The purpose of this mechanism is to facilitate a thorough comprehension of the connections between speakers, hence enhancing the sophistication and contextual awareness of an emotion recognition framework. We utilized the Gated recurrent units (GRU) to regulate context transmission, ensuring adaptability to changing emotional dynamics. It regulates the transmission of conversation context across all layers of the graph, guaranteeing a flexible and responsive representation of the changing emotional dynamics within the discourse. Evaluations on IEMOCAP, MELD, and EmoryNLP datasets disclose our model's superior performance (F1 scores: 69.1%, 63.82%, 39.85%, respectively), outperforming state-of-the-art approaches. In general, this work enhances speaker interactions by utilizing a fully connected graph, and providing a more concise and efficient ERC framework.
更多查看译文
关键词
Emotion recognition in conversation,residual relation-aware attention,deep graph-recurrent model,fully connected directed acyclic graph,positional encoding
AI 理解论文
溯源树
样例
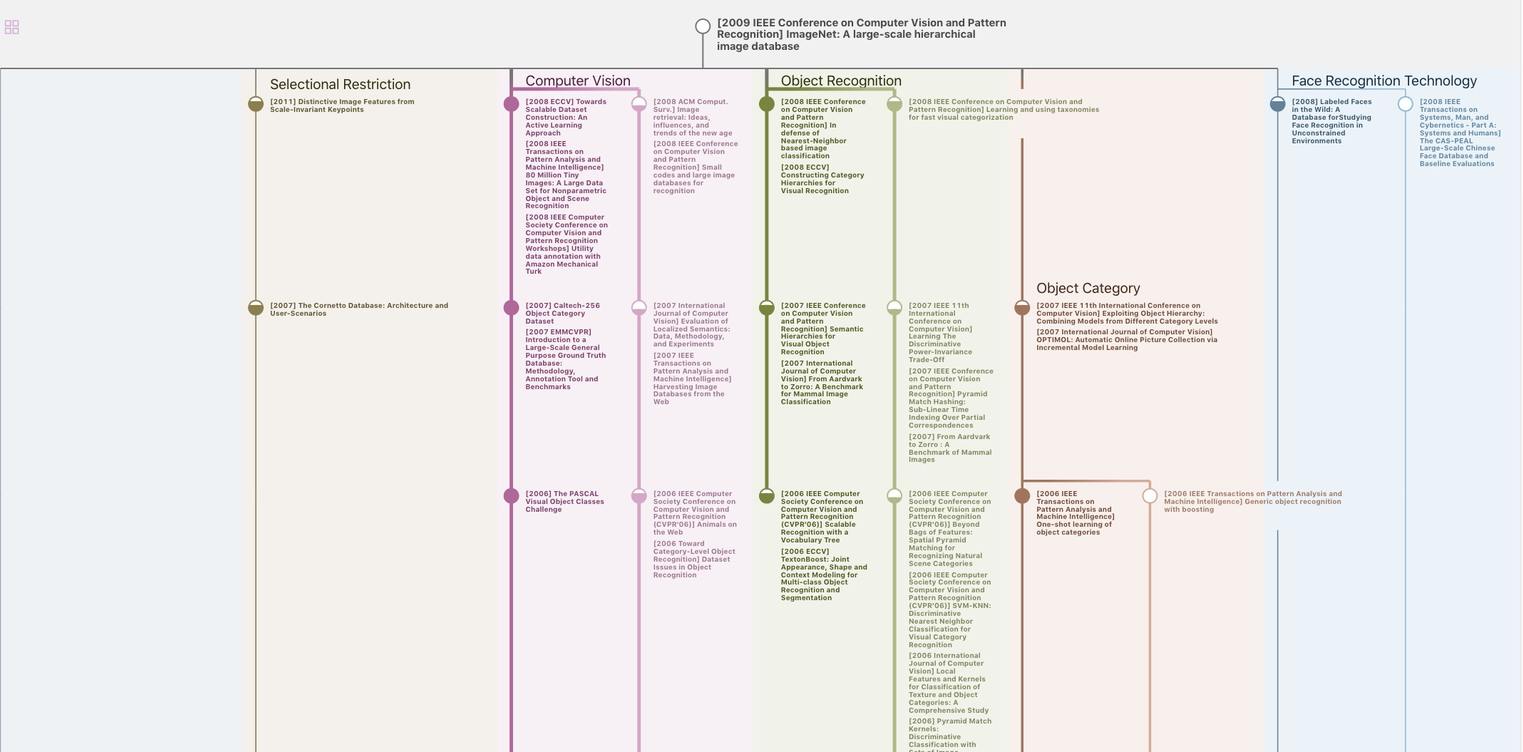
生成溯源树,研究论文发展脉络
Chat Paper
正在生成论文摘要