Interpretable Task-inspired Adaptive Filter Pruning for Neural Networks Under Multiple Constraints
International Journal of Computer Vision(2024)
摘要
Existing methods for filter pruning mostly rely on specific data-driven paradigms but lack the interpretability. Besides, these approaches usually assign layer-wise compression ratios automatically only under given FLOPs by neural architecture search algorithms or just manually, which are short of efficiency. In this paper, we propose a novel interpretable task-inspired adaptive filter pruning method for neural networks to solve the above problems. First, we treat filters as semantic detectors and develop the task-inspired importance criteria by evaluating correlations between input tasks and feature maps, and observing the information flow through filters between adjacent layers. Second, we refer to the human neurobiological mechanism for the better interpretability, where the retained first layer filters act as individual information receivers. Third, inspired by the phenomenon that each filter has a deterministic impact on FLOPs and network parameters, we provide an efficient adaptive compression ratio allocation strategy based on differentiable pruning approximation under multiple budget constraints, as well as considering the performance objective. The proposed method is validated with extensive experiments on the state-of-the-art neural networks, which significantly outperforms all the existing filter pruning methods and achieves the best trade-off between neural network compression and task performance. With ResNet-50 on ImageNet, our approach reduces 75.49% parameters and 70.90% FLOPs, only suffering from 2.31% performance degradation.
更多查看译文
关键词
Interpretable,Task-inspired,Adaptive,Filter pruning,Neural networks compression,Multiple constraints
AI 理解论文
溯源树
样例
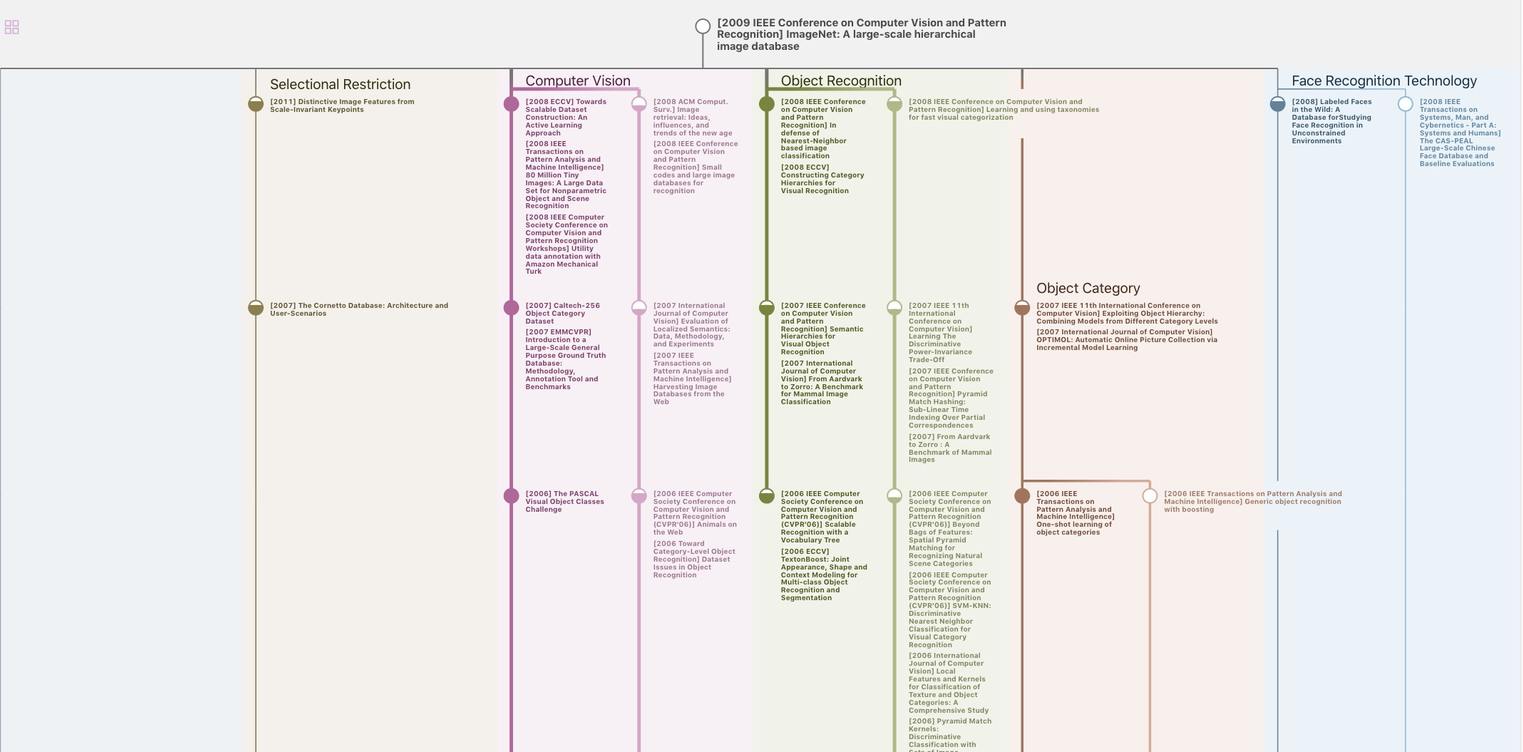
生成溯源树,研究论文发展脉络
Chat Paper
正在生成论文摘要