Nonlinear functional regression by functional deep neural network with kernel embedding
CoRR(2024)
摘要
With the rapid development of deep learning in various fields of science and
technology, such as speech recognition, image classification, and natural
language processing, recently it is also widely applied in the functional data
analysis (FDA) with some empirical success. However, due to the infinite
dimensional input, we need a powerful dimension reduction method for functional
learning tasks, especially for the nonlinear functional regression. In this
paper, based on the idea of smooth kernel integral transformation, we propose a
functional deep neural network with an efficient and fully data-dependent
dimension reduction method. The architecture of our functional net consists of
a kernel embedding step: an integral transformation with a data-dependent
smooth kernel; a projection step: a dimension reduction by projection with
eigenfunction basis based on the embedding kernel; and finally an expressive
deep ReLU neural network for the prediction. The utilization of smooth kernel
embedding enables our functional net to be discretization invariant, efficient,
and robust to noisy observations, capable of utilizing information in both
input functions and responses data, and have a low requirement on the number of
discrete points for an unimpaired generalization performance. We conduct
theoretical analysis including approximation error and generalization error
analysis, and numerical simulations to verify these advantages of our
functional net.
更多查看译文
AI 理解论文
溯源树
样例
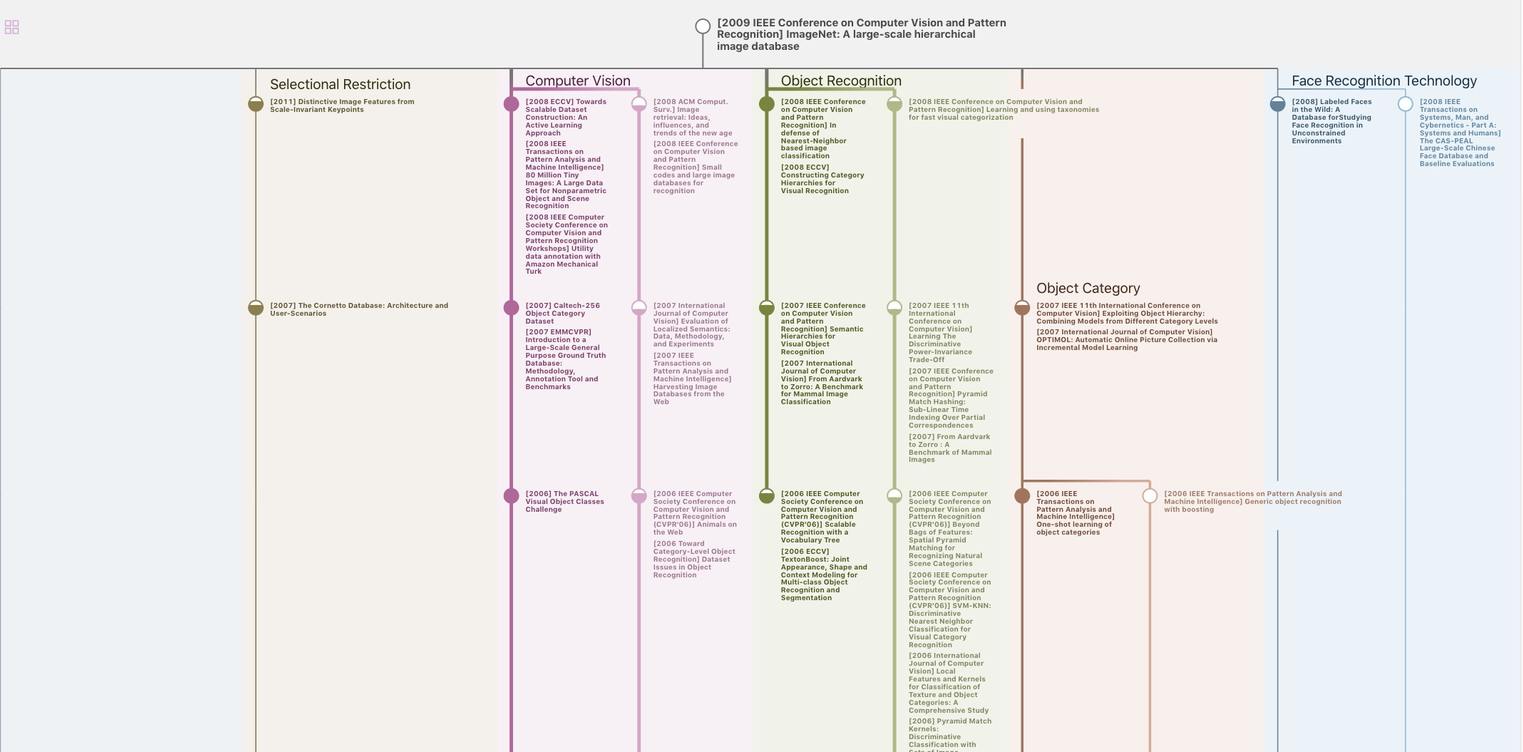
生成溯源树,研究论文发展脉络
Chat Paper
正在生成论文摘要