Affective Computing: A Topic-Based SER Approach on Collaborative Discussions in Academic Setting.
2023 IEEE Frontiers in Education Conference (FIE)(2023)
摘要
One of the biggest concerns in the modern day especially in the educational domain centers on the student's mental health. High rates of anxiety and depression have especially brought the attention of researchers in engineering education to apply affective computing to help with students' academic performance. It is known that a person's emotional states cause physiological and physical changes in the body. Emotions may impact facial expression, tone of speech, blood pressure, pulse, etc. Since visual and auditory signals are two variables that can be measured without the need to attach any physical device to the individuals, they are most studied in this field. Speech in particular has been known as a means that transfers much information about the mental and emotional states of the person. Speech Emotion Recognition (SER) is a growing field that has been applied in several domains including engineering education. Recent advancements in AI, Natural Language Understanding (NLU), and Large Language Models (LLM) have significantly streamlined this line of research. In this work which is a continuation of our prior work, we propose a speech analysis model that extracts both the emotions and topics from verbal discussions in a computer science classroom to understand if the expressed emotions were mostly about the course related topics or not. The goal of this research is to develop a tool that helps educators gain insights into the students' emotional states in teamwork and also understand the context of their conversations. We further analyze if the expressed emotions in the verbal class discussions are mostly about the course content or other subjects outside class setting. To expand the emotion analysis module we added a new layer to our developed pipeline by passing the speech data into the ChatGPT API to generate summarized scripts and extract additional classes of emotion. The preliminary results from this study are promising, indicating the potential value of this research direction and its prospects for further development. Application of this model in the educational domain can greatly benefit both educators and students and allows the instructors to make necessary interventions needed to maximize students' positive experiences in team settings while considering their emotional states.
更多查看译文
关键词
Speech Emotion Recognition (SER),Large Language Models (LLM),NLP,Topic modeling,Affective computing,ChatGPT API,Teamwork,Engineering education
AI 理解论文
溯源树
样例
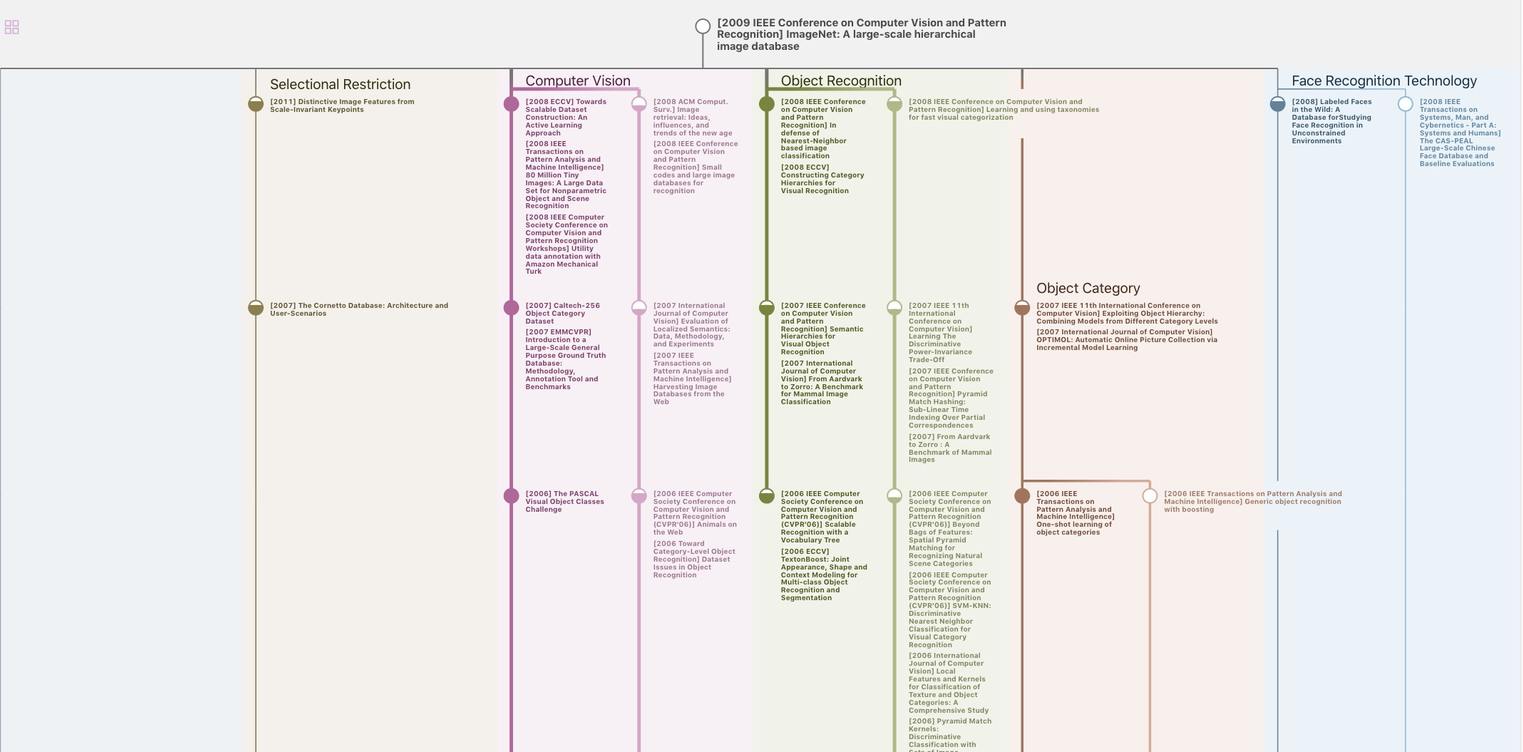
生成溯源树,研究论文发展脉络
Chat Paper
正在生成论文摘要