SecDAN: Prevention of Network Breaches in Defense Area Network Using Machine Learning Techniques
2023 International Conference on Research Methodologies in Knowledge Management, Artificial Intelligence and Telecommunication Engineering (RMKMATE)(2023)
摘要
This abstract summarizes a comprehensive study aimed at improving the safety of Defense Area Networks through the use of advanced machine learning techniques. The suggested model takes a new approach by combining deep learning architectures and anomaly detection methods to detect both established and previously unexpected network intrusions. The study draws on a variety of datasets, including network traffic and security records, and employs thorough data preparation and feature engineering procedures. The accuracy of three important algorithms is rigorously compared Random Forest, and Logistic Regression K-nearest Neighbors. The findings of this study highlight the clear edge of K-nearest Neighbors in terms of overall better performance and technique and analysis. This paper contributes to network security by proposing a comprehensive model tailored to the complexities of Defense Area Networks, hence increasing the resistance against network breaches. In the modern interconnected digital environment, the susceptibility to network breaches is particularly pronounced. These breaches, often referred to as cyberattacks or data breaches, undermine the security of information confidentiality, integrity, and accessibility by unauthorized entry into systems, networks, or private data repositories. Cybercriminals deploy a diverse array of sophisticated techniques, encompassing malicious software, phishing, ransomware, and social manipulation, to infiltrate corporate networks and abscond with sensitive data. The expanding realm of technological advancement correlates with the escalating risk of network breaches, underscoring the imperative for robust cybersecurity strategies, continuous monitoring, and swift incident response.
更多查看译文
关键词
Network Breach Detection,Malicious,Decision Tree,Cyber Attack,Random Forest,Security,Logistic Regression,Techniques,Machine Learning Model
AI 理解论文
溯源树
样例
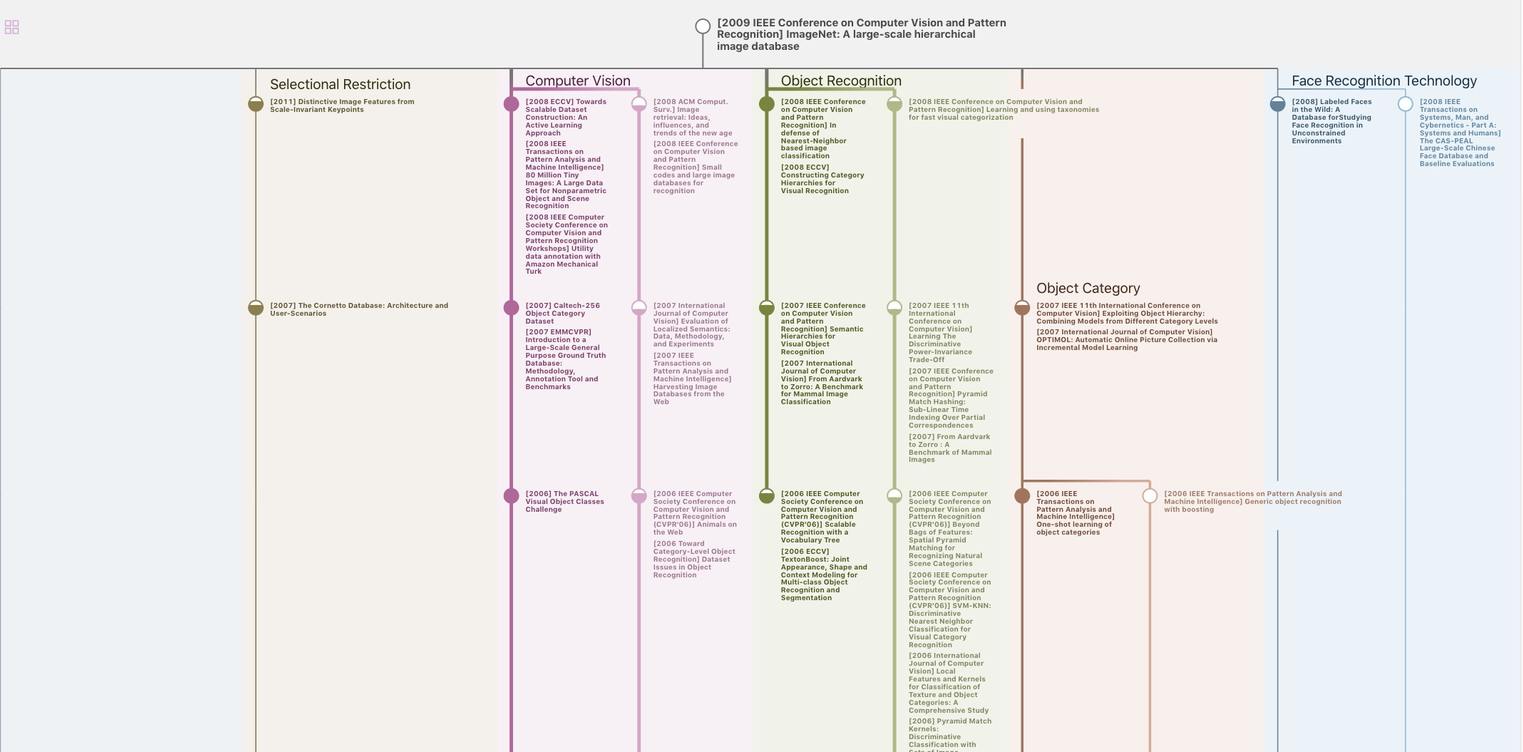
生成溯源树,研究论文发展脉络
Chat Paper
正在生成论文摘要