Time-dependent sequential association rule-based survival analysis: A healthcare application
METHODSX(2024)
摘要
The analysis of event sequences with temporal dependencies holds substantial importance across various domains, including healthcare. This study introduces a novel approach that combines sequential rule mining and survival analysis to uncover significant associations and temporal patterns within event sequences. By integrating these techniques, we address the limitations linked to the loss of temporal information. The methodology extends traditional sequential rule mining by introducing time-dependent confidence functions, providing a comprehensive understanding of relationships between antecedent and consequent events. The incorporation of the KaplanMeier estimator of survival analysis enables the calculation of temporal distributions between events, resulting in time-dependent confidence functions. These confidence functions illuminate the probability of specific event occurrences considering temporal contexts. To present the application of the method, we demonstrated the usage within the healthcare domain. Analyzing the ICD-10 codes and the laboratory events, we successfully identified relevant sequential rules and their time-dependent confidence functions. This empirical validation underscores the potential of methodology to uncover clinically significant associations within intricate medical data. center dot The study presents a unique methodology that integrates sequential rule mining and survival analysis. center dot The methodology extends traditional sequential rule mining by introducing time-dependent confidence functions. center dot The application of the method is demonstrated within the healthcare domain.
更多查看译文
关键词
Sequential rule mining,Survival analysis,Kaplan-Meier estimator,Time-dependent confidence function,Bootstrapping
AI 理解论文
溯源树
样例
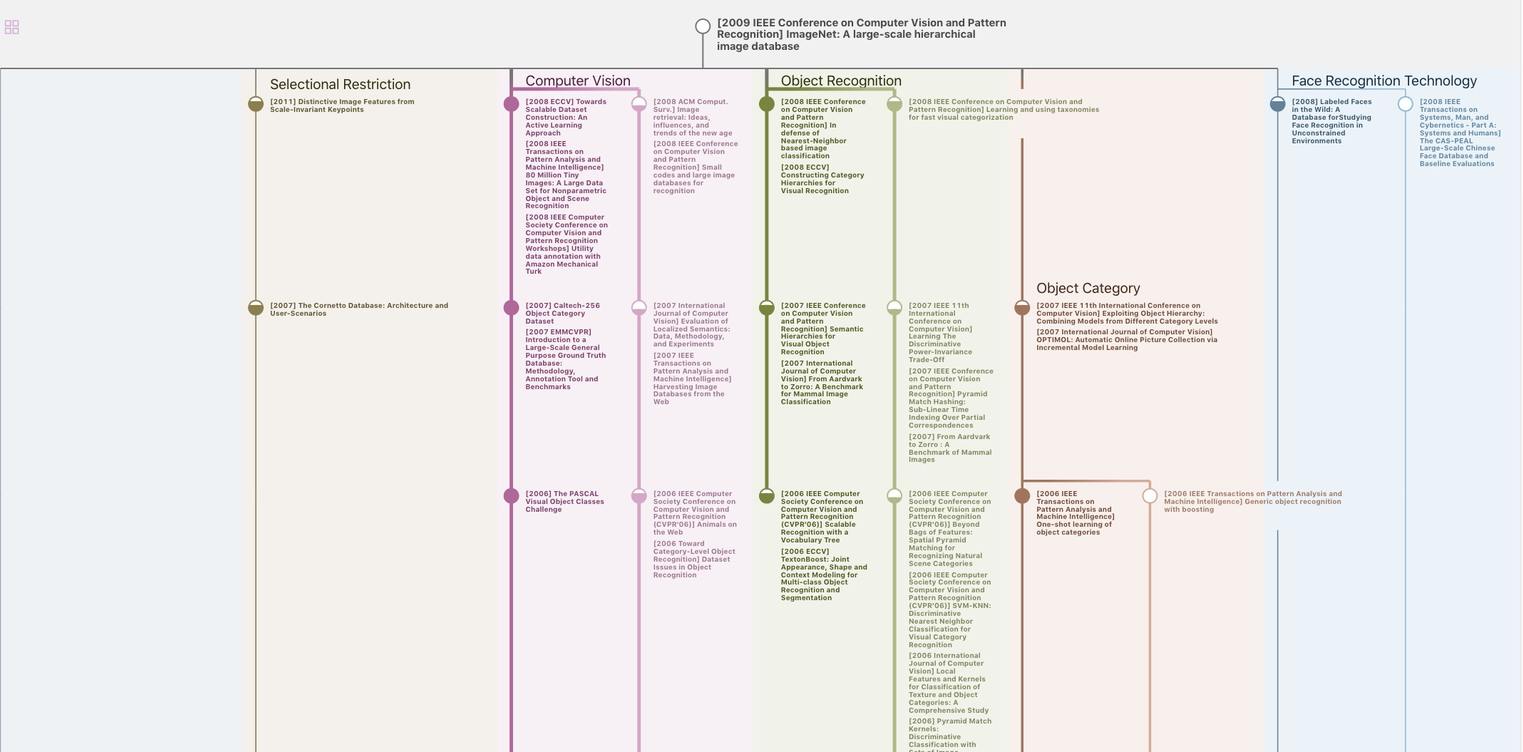
生成溯源树,研究论文发展脉络
Chat Paper
正在生成论文摘要