Step selection analysis with non-linear and random effects in mgcv
biorxiv(2024)
摘要
Step selection analysis is used to jointly describe animal movement patterns and habitat preferences. Recent work has extended this framework to model inter-individual differences, account for unexplained structure in animals' space use, and capture temporally-varying patterns of movement and habitat selection. In this paper, we formulate step selection functions with penalised smooths (similar to generalised additive models) to unify new and existing extensions, and conveniently implement the models in the popular, open-source mgcv R package. We explore non-linear patterns of movement and habitat selection, and use the equivalence between penalised smoothing splines and random effects to implement individual-level and spatial random effects. This framework can also be used to fit varying-coefficient models to account for temporally or spatially-heterogeneous patterns of selection (e.g., resulting from behavioural variation), or any other non-linear interactions between drivers of the animal's movement decisions. We provide a broad overview of how smooth effects can be applied to increase the flexibility and biological realism of step selection analysis. We provide the necessary technical details to understand several key special cases of smooths and their implementation in mgcv, showcase the ecological relevance using two illustrative examples, and provide R code (available at https://github.com/NJKlappstein/smoothSSF) to facilitate the adoption of these methods.
### Competing Interest Statement
The authors have declared no competing interest.
更多查看译文
AI 理解论文
溯源树
样例
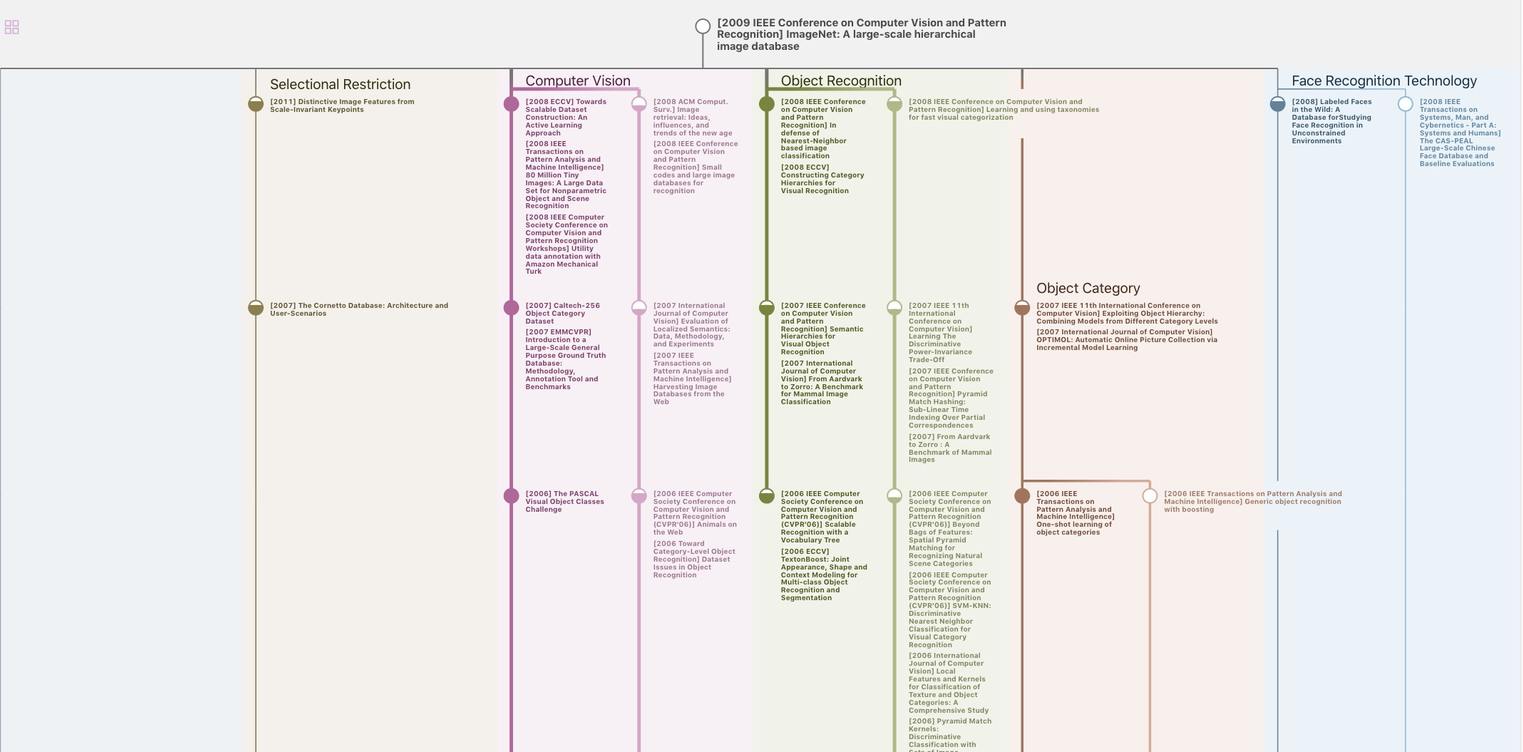
生成溯源树,研究论文发展脉络
Chat Paper
正在生成论文摘要