Clustering-Based Contrastive Learning for Fault Diagnosis With Few Labeled Samples.
IEEE Transactions on Instrumentation and Measurement(2024)
摘要
In recent years, the utilization of deep learning (DL) for fault diagnosis has become more and more prevalent. However, most DL methods rely on a large amount of labeled data to train models, which could lead to their poor generalization ability when applied to different scenarios. Moreover, labels are precious and not easily accessible in practical industrial production environments. To overcome these drawbacks, we propose a clustering-based contrastive learning (CCL) framework. Specifically, data augmentation (DA) is first applied to the raw data by converting them into two related views using a dropout method. Next, we adopt an inter-instance contrasting module based on dynamic clustering to learn the discriminability representation of the neural network model. This module seeks to maximize the similarity of the same sample features while minimizing the similarity between different sample features. Finally, an intra-instance temporal contrast module is designed to learn the intra-instance temporal relationship by undertaking a challenging cross-prediction task to establish robust temporal representations. The effectiveness of our proposed method is demonstrated by the experimental results of three public rotating machinery datasets and one dataset collected from 15 pumps in the Tengzhou factory. In the most difficult task where only 1% of the data are labeled, the proposed CCL can improve the accuracy by a maximum of 23.13% over the supervised learning baseline. This method can significantly improve the diagnostic performance and generalization ability of the model with limited labels.
更多查看译文
关键词
Contrastive learning (CL),fault diagnosis,few labeled data,self-supervised learning (SSL)
AI 理解论文
溯源树
样例
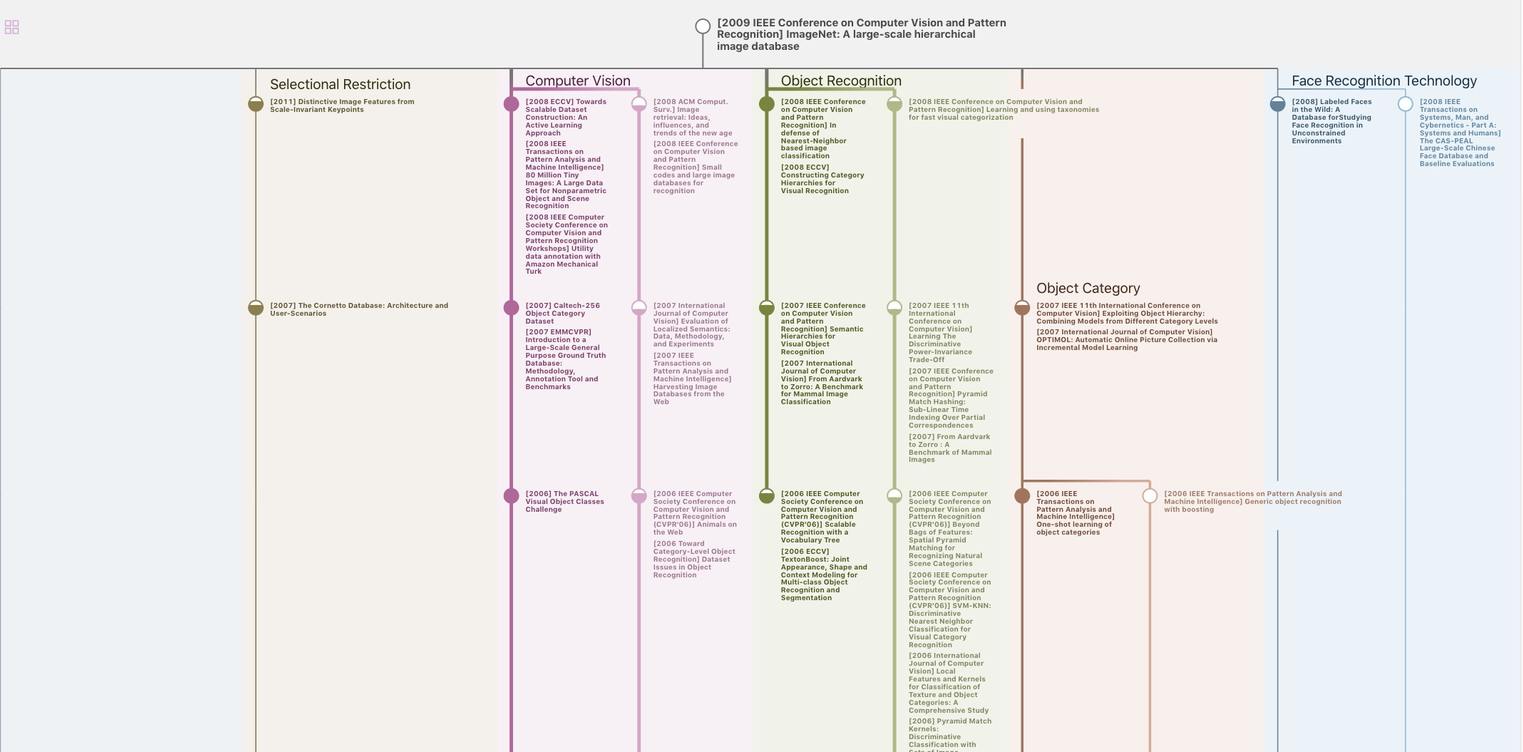
生成溯源树,研究论文发展脉络
Chat Paper
正在生成论文摘要