Human-in-the-Loop Policy Optimization for Preference-Based Multi-Objective Reinforcement Learning
CoRR(2024)
摘要
Multi-objective reinforcement learning (MORL) aims to find a set of
high-performing and diverse policies that address trade-offs between multiple
conflicting objectives. However, in practice, decision makers (DMs) often
deploy only one or a limited number of trade-off policies. Providing too many
diversified trade-off policies to the DM not only significantly increases their
workload but also introduces noise in multi-criterion decision-making. With
this in mind, we propose a human-in-the-loop policy optimization framework for
preference-based MORL that interactively identifies policies of interest. Our
method proactively learns the DM's implicit preference information without
requiring any a priori knowledge, which is often unavailable in real-world
black-box decision scenarios. The learned preference information is used to
progressively guide policy optimization towards policies of interest. We
evaluate our approach against three conventional MORL algorithms that do not
consider preference information and four state-of-the-art preference-based MORL
algorithms on two MORL environments for robot control and smart grid
management. Experimental results fully demonstrate the effectiveness of our
proposed method in comparison to the other peer algorithms.
更多查看译文
AI 理解论文
溯源树
样例
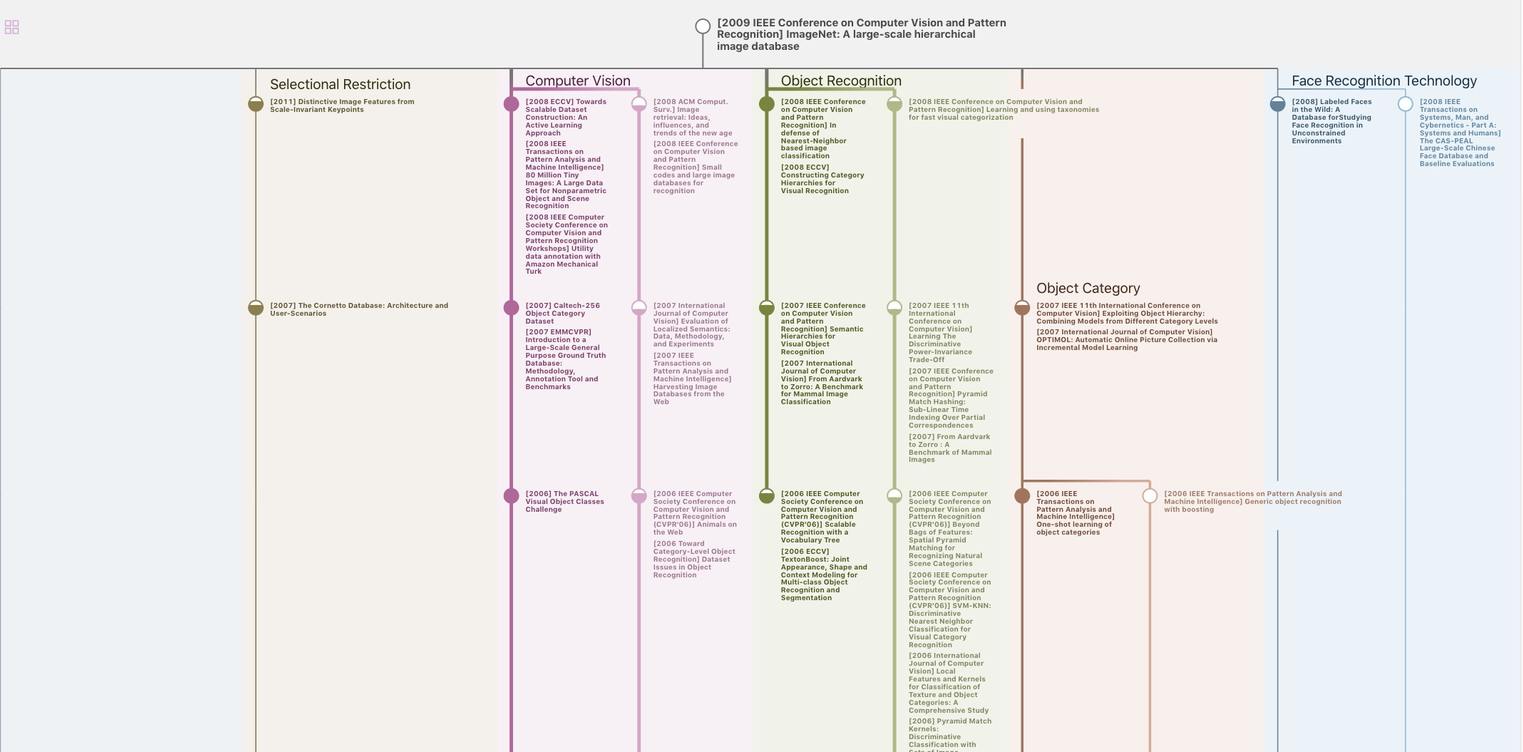
生成溯源树,研究论文发展脉络
Chat Paper
正在生成论文摘要