Efficient Neuroevolution Using Island Repopulation and Simplex Hyperparameter Optimization.
2023 IEEE Symposium Series on Computational Intelligence (SSCI)(2023)
摘要
Recent studies have shown that the performance of evolutionary neural architecture search (i.e., neuroevolution) algorithms can be significantly improved by the use of island based strategies which periodically experience extinction and repopulation events. Further, it has been shown that the simplex hyperparameter optimization (SHO) method can also improve neuroevolution (NE) performance by optimizing neural network training hyperparameters while the NE algorithm also trains and designs neural networks. This work provides an extensive examination of combining island repopulation events with five different island-based variations of SHO. These methods are evaluated for the evolution of recurrent neural networks for the challenging problem of multivariate time series forecasting on two real world datasets. We show with statistical significance that adding repopulation to the SHO variants in almost every case improves performance, and for those that does there is no statistical difference. In addition, we find that one variant in particular, multi-island, random island best genome (MIRIB) performs the best across all experiment types.
更多查看译文
关键词
Neuroevolution,Time Series Forecasting,Hy-perparameter Optimization,Recurrent Neural Networks
AI 理解论文
溯源树
样例
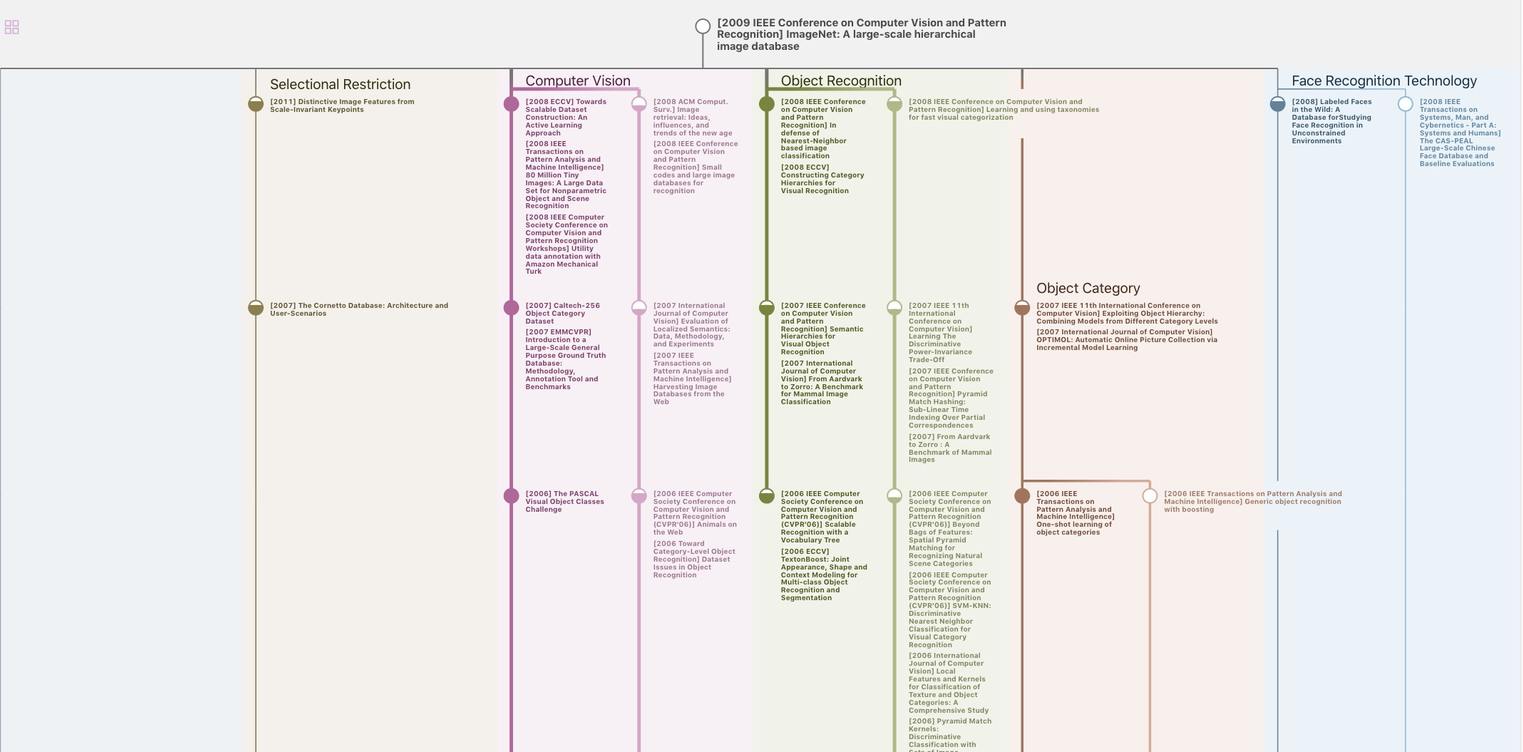
生成溯源树,研究论文发展脉络
Chat Paper
正在生成论文摘要