SIGNRL: A Population-Based Reinforcement Learning Method for Continuous Control.
2023 IEEE Symposium Series on Computational Intelligence (SSCI)(2023)
摘要
In engineering processes that require continuous control, it is common to face significant challenges. Addressing these challenges through explicit modeling can take much work and effort. For this reason, Reinforcement Learning (RL) has gained popularity as a feasible strategy for solving this problem. In this context, various value-based methodologies, policies, or combinations have been employed to obtain an optimal learning policy. However, problems such as convergence to local maxima and high variance in training persist. In addition, computational time and cost increase in complex environments, so more robust RL methodologies are required. This paper proposes a Swarm Intelligence Guided Neural Reinforcement Learning (SIGNRL) algorithm, which uses Particle Swarm Optimization as a multi-agent parameter explorer to find the optimal policy. Numerical results obtained in the OpenAI Gym Cart-Pole environment show that SIGNRL, with its gradient-free learning, exhibits good convergence and lower variance in continuous control tasks.
更多查看译文
关键词
Reinforcement Learning,Artificial Neural Networks,Particle Swarm Optimization,Control Systems
AI 理解论文
溯源树
样例
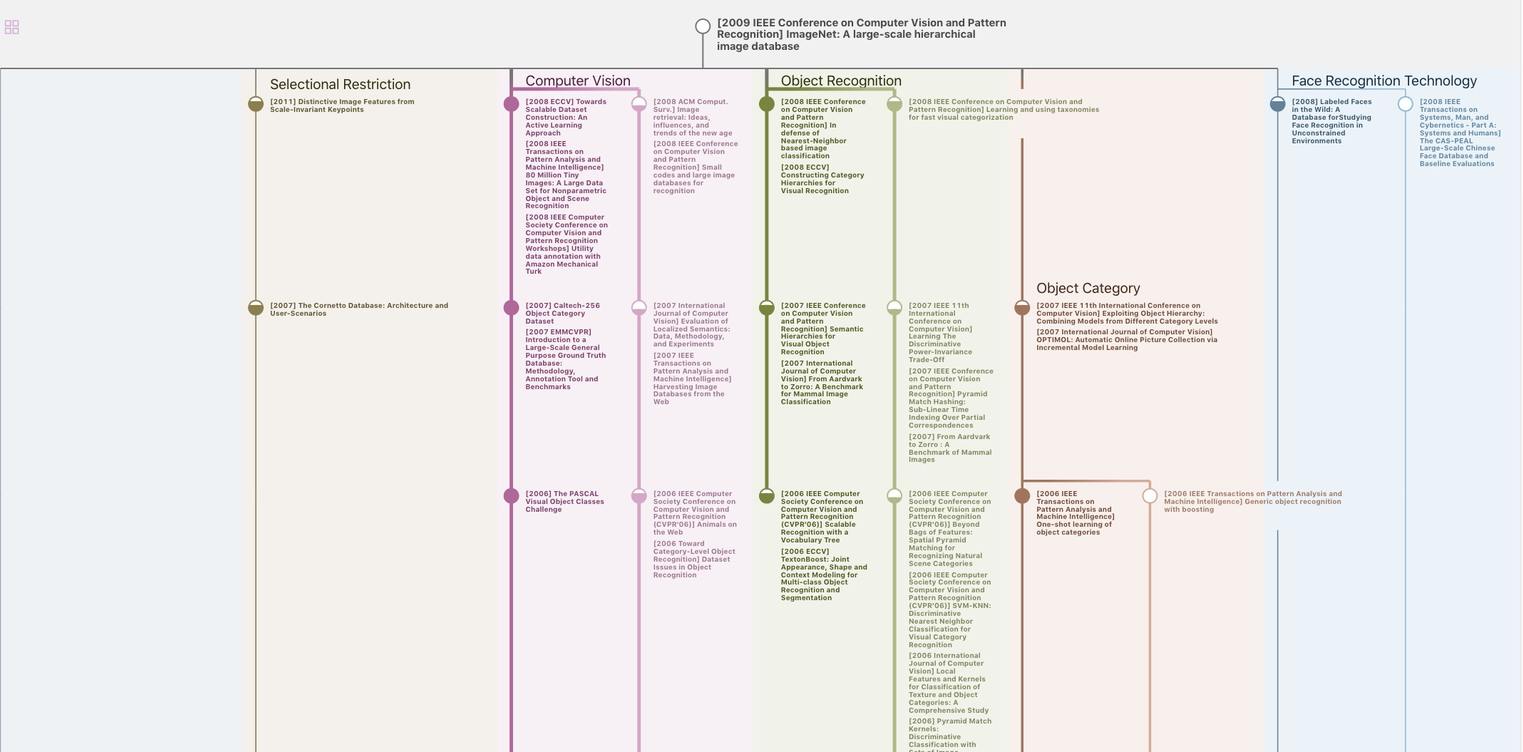
生成溯源树,研究论文发展脉络
Chat Paper
正在生成论文摘要