The neuron as a direct data-driven controller.
Proceedings of the National Academy of Sciences of the United States of America(2024)
摘要
In the quest to model neuronal function amid gaps in physiological data, a promising strategy is to develop a normative theory that interprets neuronal physiology as optimizing a computational objective. This study extends current normative models, which primarily optimize prediction, by conceptualizing neurons as optimal feedback controllers. We posit that neurons, especially those beyond early sensory areas, steer their environment toward a specific desired state through their output. This environment comprises both synaptically interlinked neurons and external motor sensory feedback loops, enabling neurons to evaluate the effectiveness of their control via synaptic feedback. To model neurons as biologically feasible controllers which implicitly identify loop dynamics, infer latent states, and optimize control we utilize the contemporary direct data-driven control (DD-DC) framework. Our DD-DC neuron model explains various neurophysiological phenomena: the shift from potentiation to depression in spike-timing-dependent plasticity with its asymmetry, the duration and adaptive nature of feedforward and feedback neuronal filters, the imprecision in spike generation under constant stimulation, and the characteristic operational variability and noise in the brain. Our model presents a significant departure from the traditional, feedforward, instant-response McCulloch-Pitts-Rosenblatt neuron, offering a modern, biologically informed fundamental unit for constructing neural networks.
更多查看译文
AI 理解论文
溯源树
样例
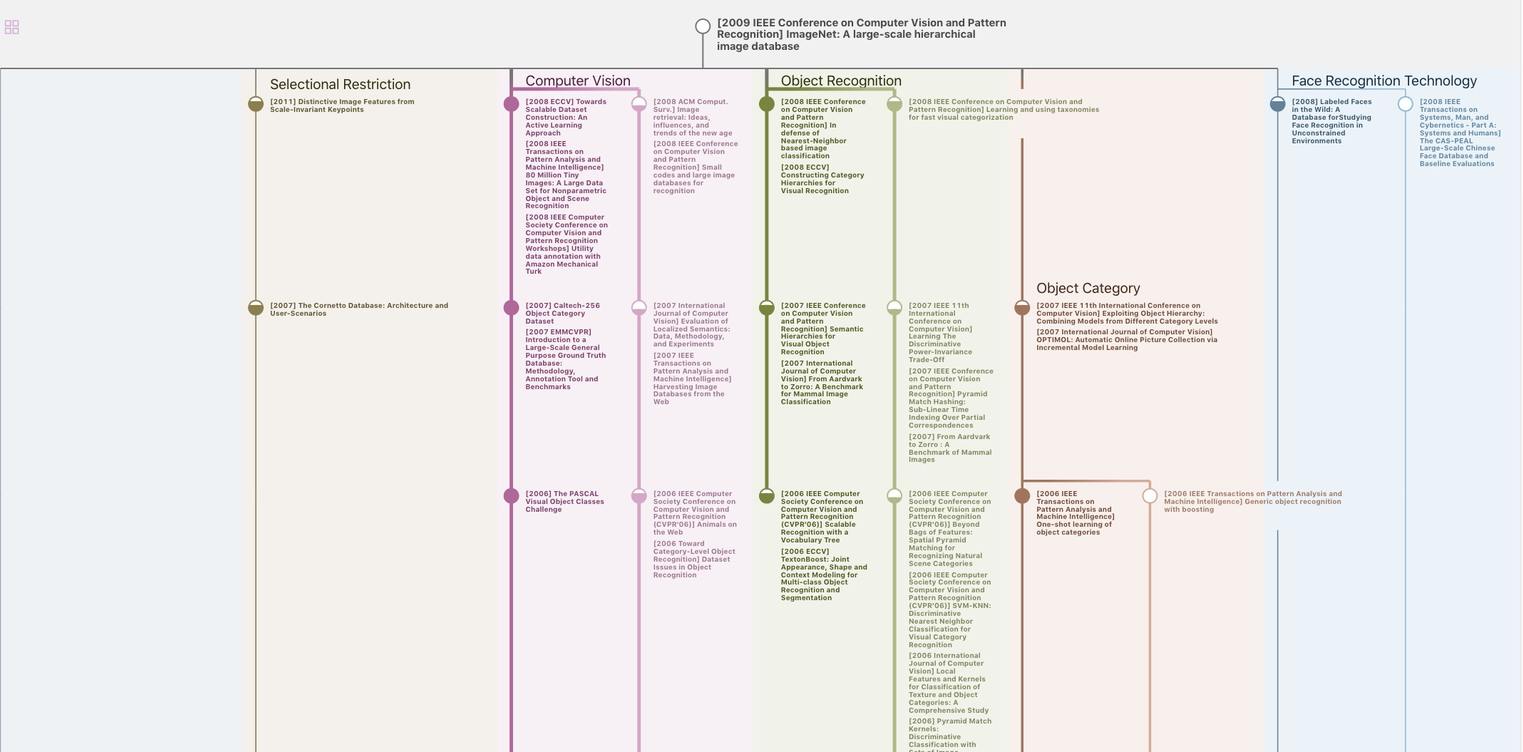
生成溯源树,研究论文发展脉络
Chat Paper
正在生成论文摘要