Threshold-Free Network-Oriented Statistics in Neuroscience
biorxiv(2024)
摘要
Network neuroscience has emerged as a powerful tool for studying brain structure and function. Currently, the network-based statistic (NBS) method is widely used to control the type I error rate for mass univariate tests on brain network edges. However, the NBS requires the selection of a cluster-forming threshold, which lacks objective guidelines. There is also an urgent need for a powerful and flexible statistical framework for analyzing brain networks in diverse contexts. Here, we introduce a permutation-based framework called “threshold-free network-oriented statistics” (TFNOS). It integrates two “threshold-free” pathways: traversing all cluster-forming thresholds (TT) and using predefined clusters (PC). The TT procedure, building upon the threshold-free network-based statistics, requires the setting of additional parameters. The PC procedures have six variants according to the way of pooling data, the form of the null distribution, and the controlled error rate. Using numerical simulations, we comprehensively evaluated the performance of the TT procedure under 600 parameter combinations, benchmarked TFNOS procedures and baselines across different topologies of effects, sample sizes, and effect sizes, and provided examples of application on real data. We offer recommended parameter values that can make the TT procedure stably maintain leading power while empirically controlling the false discovery rate (FDR) at an appropriate level. Analyses reveal that the recommended parameters available in the field are overly liberal. Furthermore, for the PC procedures, FDR-controlling variants showed improved power compared to FWER-controlling variants, some of which are simple but do not compromise power. The nonparametric PC procedures allow for the selection of any test statistics considered appropriate. Overall, we provide empirical and principled criteria for selecting statistical procedures and the TFNOS is a generalized framework for inference in various contexts on edges or nodes of undirected or directed brain networks.
### Competing Interest Statement
The authors have declared no competing interest.
更多查看译文
AI 理解论文
溯源树
样例
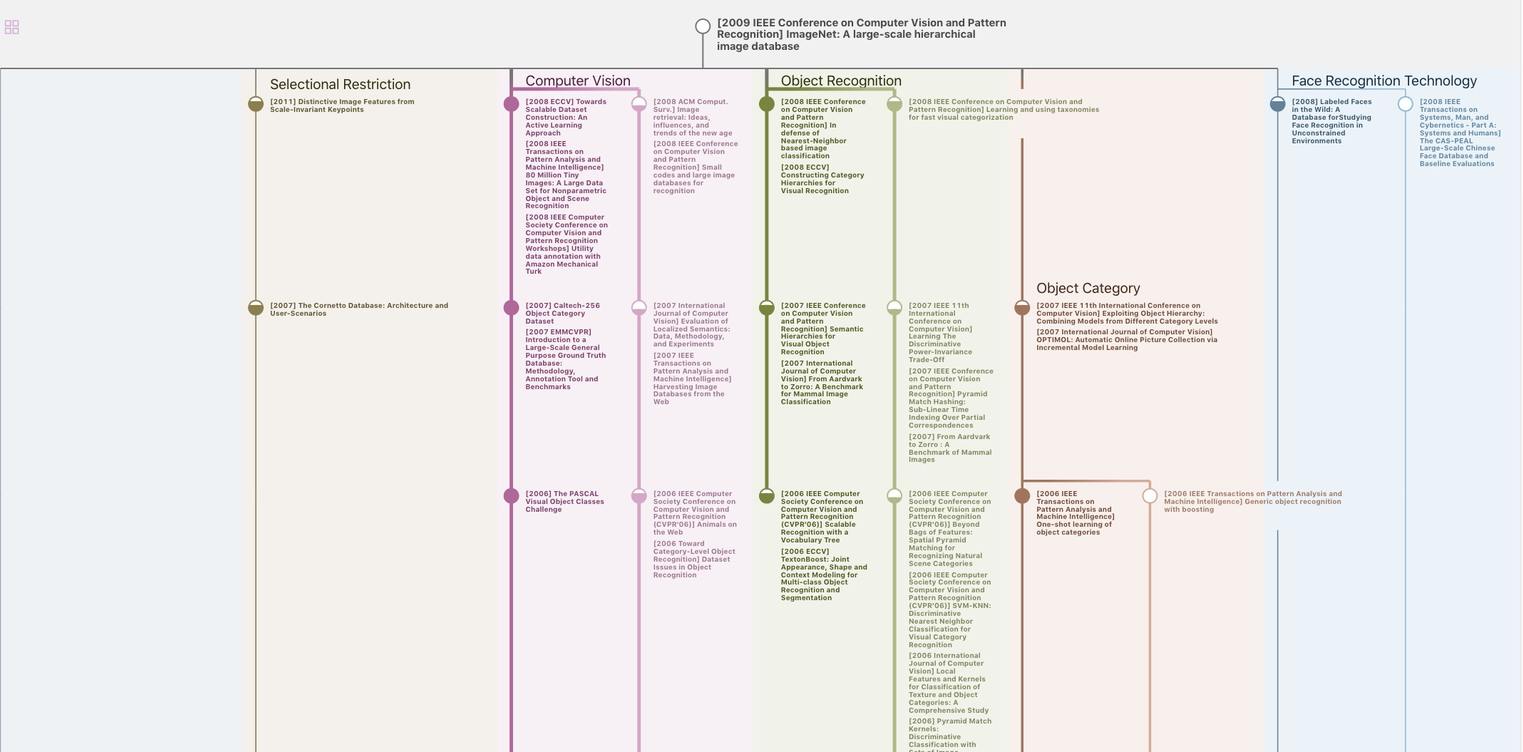
生成溯源树,研究论文发展脉络
Chat Paper
正在生成论文摘要