Protecting Vulnerable Road Users: Semantic Video Analysis for Accident Prediction.
2023 IEEE Symposium Series on Computational Intelligence (SSCI)(2023)
摘要
Pedestrians and cyclists are some of the most vulnerable, but also least predictable traffic participants. Due to their ability to move in urban environments with high degrees of freedom and sudden changes of direction, their movement is still challenging to predict. We present a driver assistance system that tackles some of these challenges. Our system consists of a world model made of a variational autoencoder and a long short-term memory network. The world model takes vision and action data from the perspective of the vulnerable traffic participant and generates a visual prediction (image) of their environment up to one second in advance. The second part of our system is a transformer-based description system that takes the predicted perceptions and here, as a showcase, abstracts them down to a textual warning if a collision between car and vulnerable traffic participant seems imminent. Our description system helps contextualize the dangerous situation for the driver and could be extended to other driver assistance systems, such as blind spot detection. We evaluate our system on a dataset generated in simulations using CARLA.
更多查看译文
关键词
autonomous driving,machine learning,video description,world models
AI 理解论文
溯源树
样例
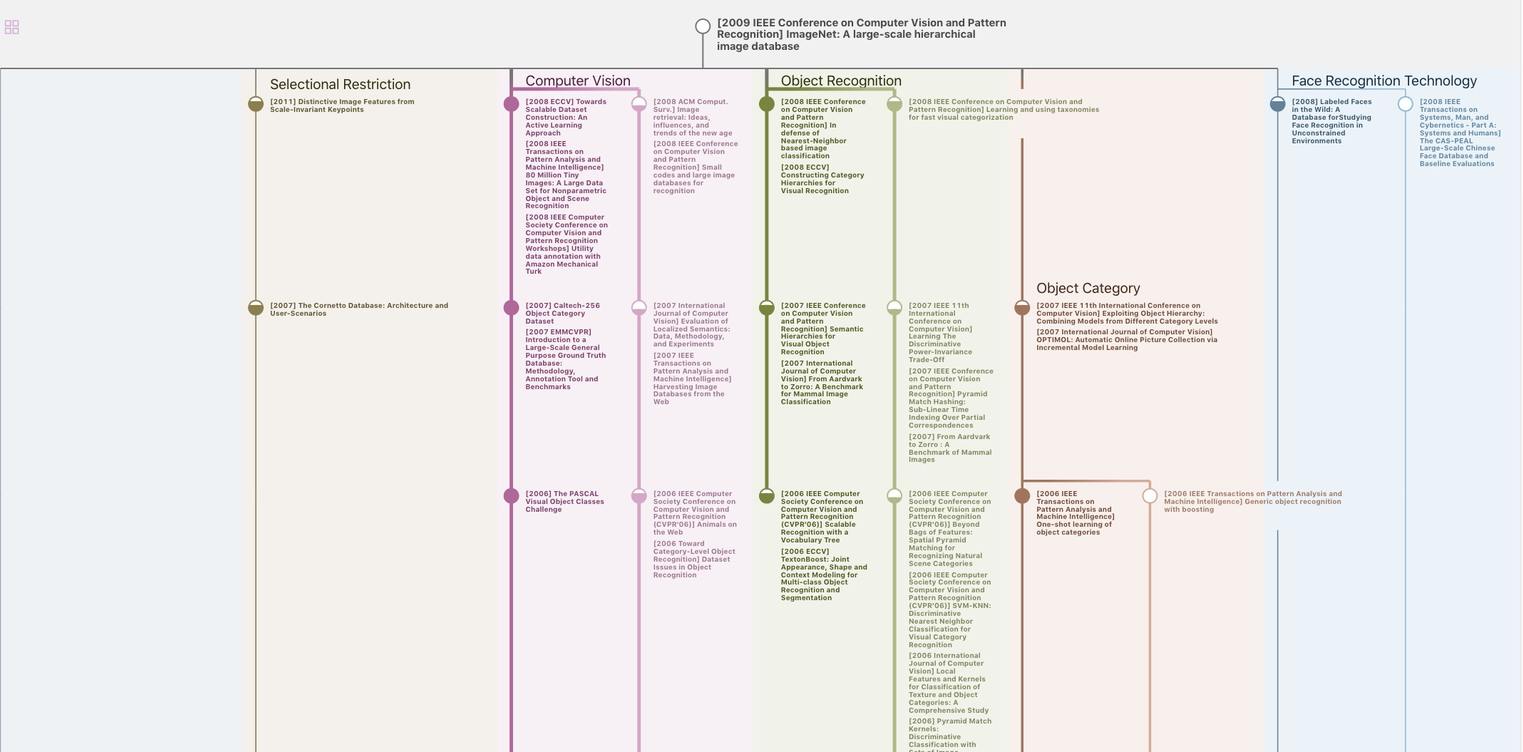
生成溯源树,研究论文发展脉络
Chat Paper
正在生成论文摘要