Parameter-Adaptive Paired Offspring Generation for Constrained Large-Scale Multiobjective Optimization Algorithm.
2023 IEEE Symposium Series on Computational Intelligence (SSCI)(2023)
摘要
There are significant challenges in designing optimization algorithms for constrained large-scale multiobjective optimization problems due to numerous decision variables and constraints. For example, the decision space size exponentially grows with the number of decision variables, and constraints restrict the feasible range, increasing the complexity of the search space. To solve these problems, this paper presents a constrained large-scale multiobjective optimization algorithm based on adaptive paired offspring generation (aPOCEA). Specifically, an adaptive parameter adjustment strategy is proposed to determine the number of solutions in each subpopulation and balance the exploration and exploitation ability of the algorithm, enhancing the convergence speed of aPOCEA. Meanwhile, we propose a parent selection strategy to select high-quality parent solutions, increasing the probability of generating high-quality offspring solutions. Experimental results on ten benchmarks, each with two to three objectives, multiple constraints, and hundreds of decision variables, demonstrate that aPOCEA outperforms other representative optimization algorithms.
更多查看译文
关键词
Constrained large-scale multiobjective optimization,adaptive parameter adjustment strategy,parent selection strategy
AI 理解论文
溯源树
样例
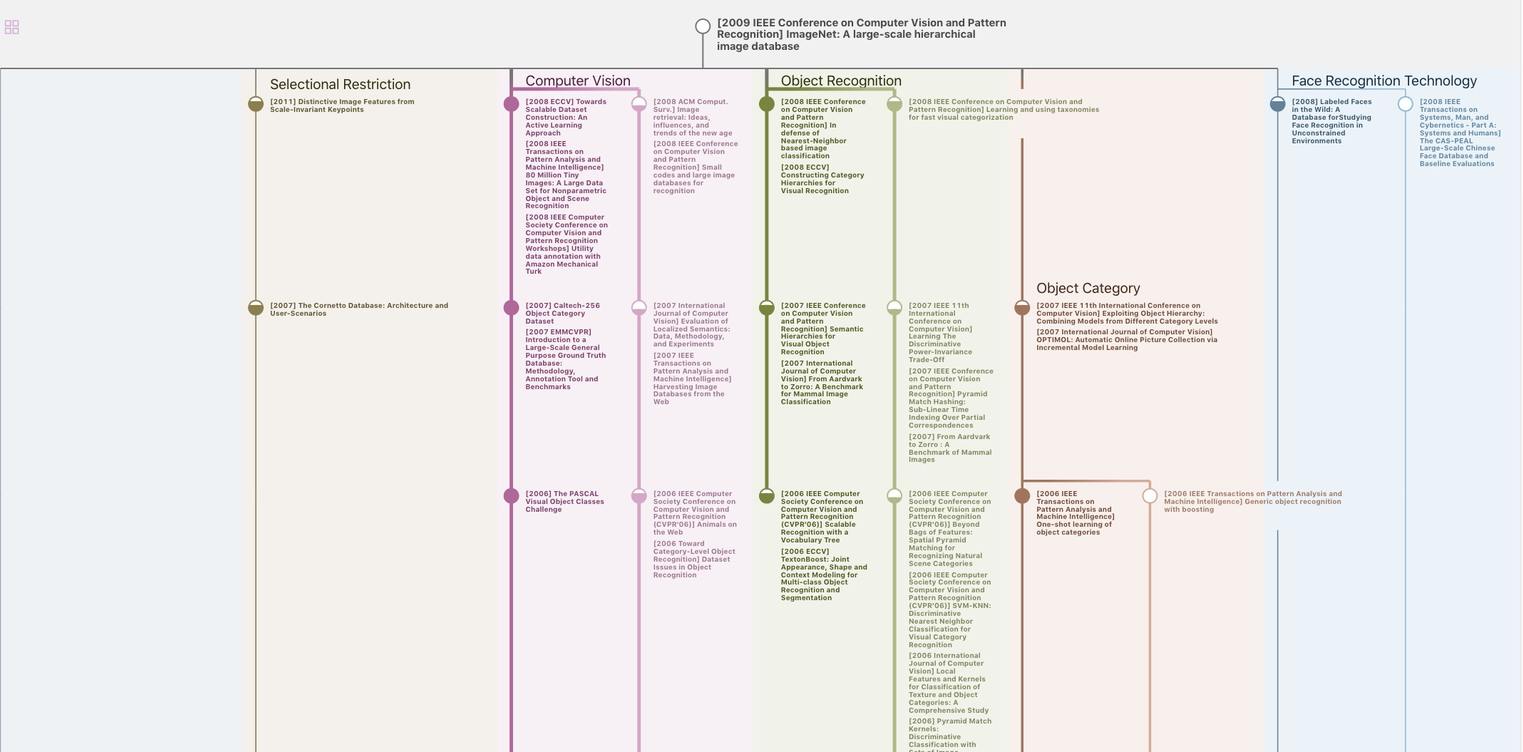
生成溯源树,研究论文发展脉络
Chat Paper
正在生成论文摘要