Saliency-aware regularized graph neural network
ARTIFICIAL INTELLIGENCE(2024)
摘要
The crux of graph classification lies in the effective representation learning for the entire graph. Typical graph neural networks focus on modeling the local dependencies when aggregating features of neighboring nodes, and obtain the representation for the entire graph by aggregating node features. Such methods have two potential limitations: 1) the global node saliency w.r.t. graph classification is not explicitly modeled, which is crucial since different nodes may have different semantic relevance to graph classification; 2) the graph representation directly aggregated from node features may have limited effectiveness to reflect graph -level information. In this work, we propose the Saliency -Aware Regularized Graph Neural Network (SAR-GNN) for graph classification, which consists of two core modules: 1) a traditional graph neural network serving as the backbone for learning node features and 2) the Graph Neural Memory designed to distill a compact graph representation from node features of the backbone. We first estimate the global node saliency by measuring the semantic similarity between the compact graph representation and node features. Then the learned saliency distribution is leveraged to regularize the neighborhood aggregation of the backbone, which facilitates the message passing of features for salient nodes and suppresses the less relevant nodes. Thus, our model can learn more effective graph representation. We demonstrate the merits of SAR-GNN by extensive experiments on seven datasets across various types of graph data.
更多查看译文
关键词
Graph neural network,Graph classification
AI 理解论文
溯源树
样例
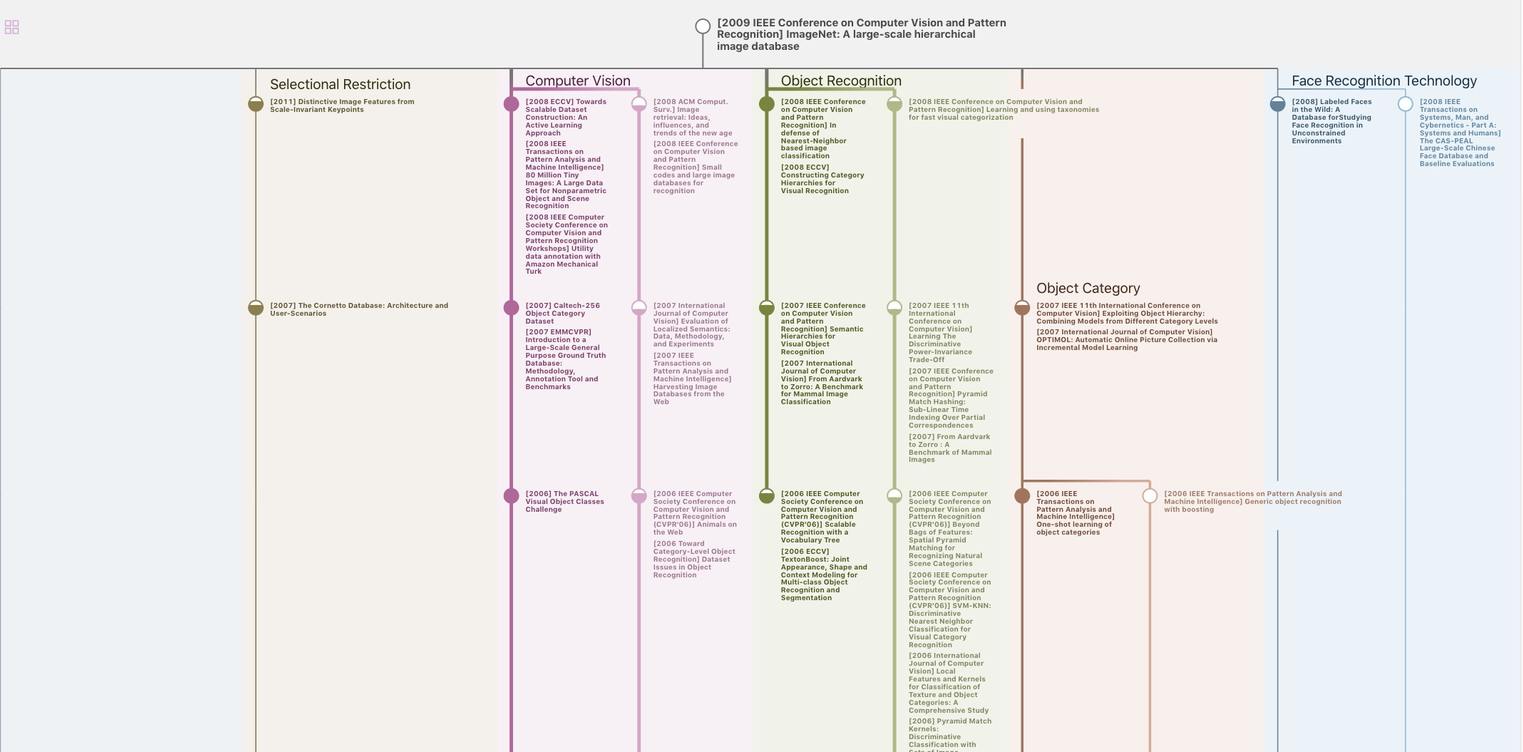
生成溯源树,研究论文发展脉络
Chat Paper
正在生成论文摘要