Multi-View Multi-Label Personalized Classification via Generalized Exclusive Sparse Tensor Factorization
crossref(2024)
摘要
Abstract Multi-Label Classification (MLC) assigns multiple relevant labels to each sample simultaneously,while multi-view MLC aims to apply MLC to handle heterogeneous data represented by multiple feature subsets.In recent years, a variety of methods have been proposed to handle these problems and have achieved great success in a wide range of applications. MLC saves global label correlation by building a single model shared by all samples but ignores sample-specific local structures, while Personalized Learning (PL) is able to preserve sample-specific information by learning local models but ignores the global structure. Integrating PL with MLC is a straightforward way to overcome the limitations, but it still faces three key challenges. 1) capture both local and global structures in a unified model; 2) efficiently preserve full-order interactions between labels, samples and features or multi-view features in heterogeneous data; 3) learn a concise and interpretable model where only a fraction of interactions are associated with multiple labels. In this paper, we propose a novel Multi-Label Personalized Classification (MLPC) method and its multi-view extension to handle these challenges. For 1), it integrates local and global components to preserve sample-specific information and global structure shared across samples, respectively. For 2), a multilinear model is developed to capture full-order label-feature-sample interactions, and over-parameterization is avoided by tensor factorization. For 3), exclusive sparsity regularization penalizes factorization by promoting intra-group competition, thereby eliminating irrelevant and redundant interactions during Exclusive Sparse Tensor Factorization (ESTF). Moreover, theoretical analysis generalizes the proposed ESTF and reveals the equivalence between MLPC and a family of jointly regularized counterparts. We develop an alternating algorithm to solve the optimization problem, and demonstrate its effectiveness based on comprehensive experiments on both synthetic and real-world benchmark datasets.
更多查看译文
AI 理解论文
溯源树
样例
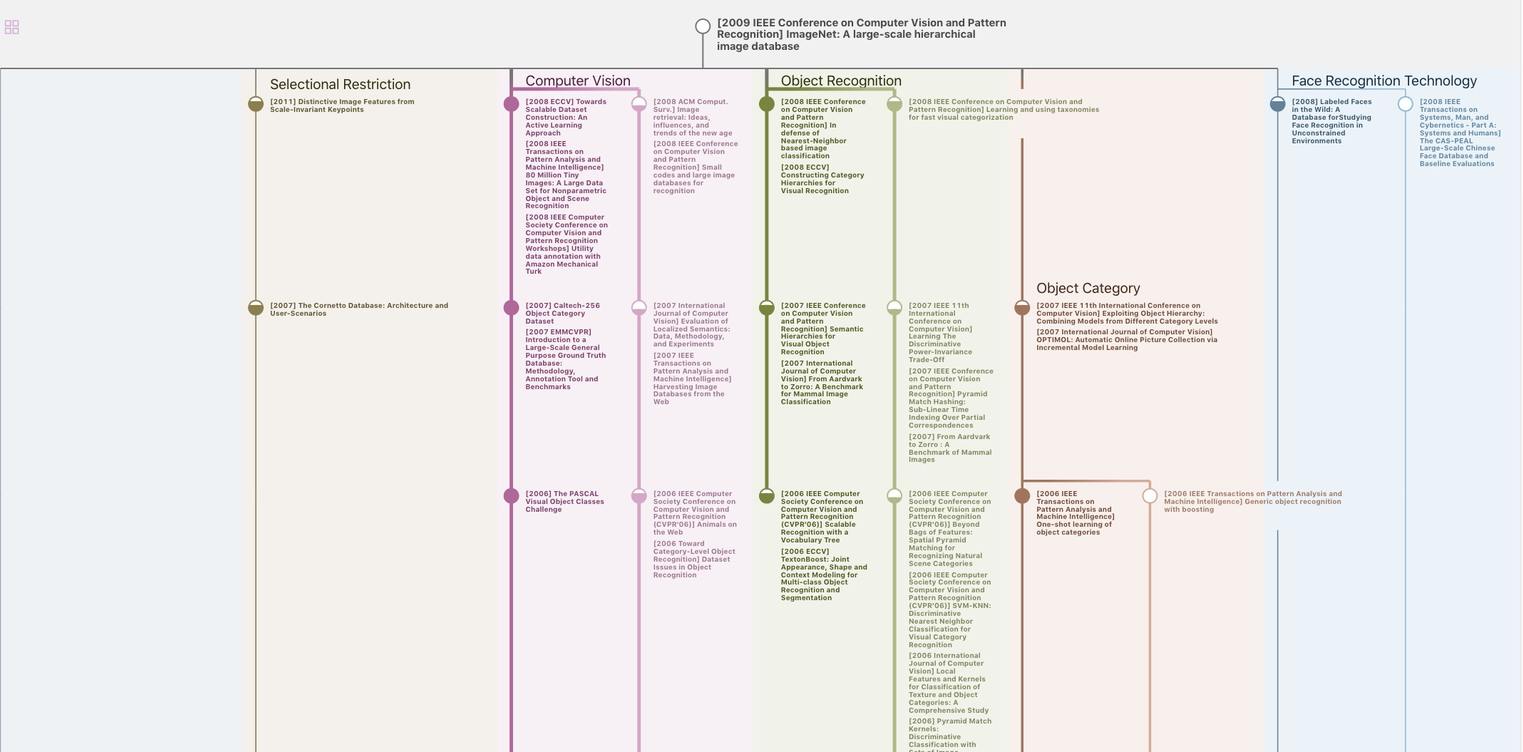
生成溯源树,研究论文发展脉络
Chat Paper
正在生成论文摘要