Lightweight image super-resolution via multi-branch aware CNN and efficient transformer
Neural Computing and Applications(2023)
摘要
A hybrid architecture model of multi-branch aware CNN and efficient transformer (MAET) is proposed and implemented for lightweight image super-resolution (SR). In the model, the multi-branch aware block (MAB) removes the redundant branches when their local spatial features are captured by other branches, while the efficient transformer block (ETB) applies the scaled cosine attention (SCA) to scale up the model capacity by generating mild attentional values from the pixel pairs. By removing the redundant branches and applying SCA to scale up the model capacity, we believe that the model is able to improve the performance while maintaining a low computation complexity. Specifically, MAET consists of a multi-branch aware CNN module (MACM) and an efficient transformer module (ETM). MACM is a lightweight CNN module composed of a serious of MABs to extract hierarchical local features. ETM is composed of ETBs to fully exploit global information by modeling long-term image dependencies to refine the texture details. ETB adopts the feature split strategy, residual post-normalization, and SCA for efficient multi-head attention. Extensive experiments demonstrate that the proposed MAET achieves better accuracy and visual improvements against the state-of-the-art lightweight image SR methods in terms of quantitative and qualitative evaluations.
更多查看译文
关键词
Lightweight image super-resolution,Multi-branch aware,Transformer,Deep learning
AI 理解论文
溯源树
样例
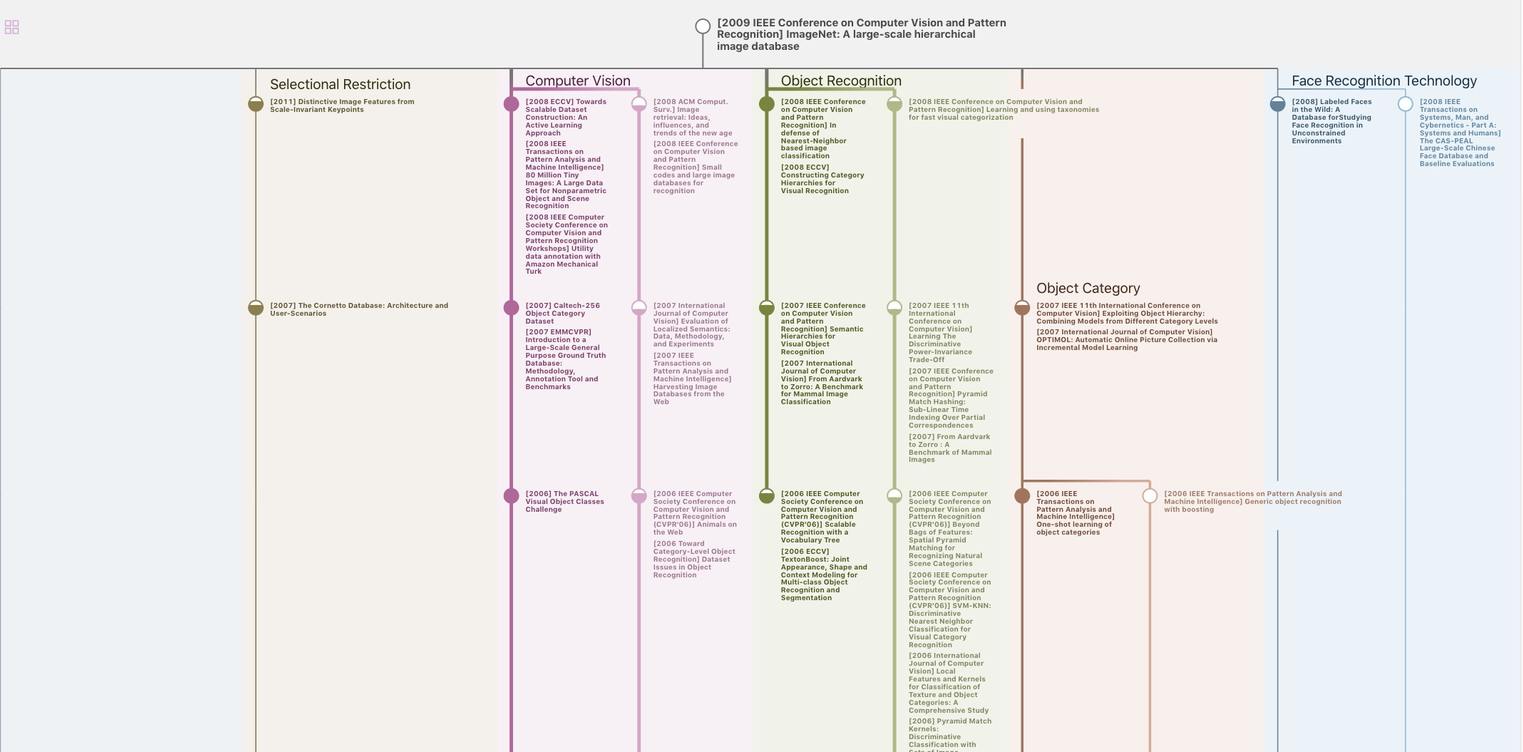
生成溯源树,研究论文发展脉络
Chat Paper
正在生成论文摘要