Adaptive trajectory-constrained exploration strategy for deep reinforcement learning
KNOWLEDGE-BASED SYSTEMS(2024)
摘要
Deep reinforcement learning (DRL) faces significant challenges in addressing hard -exploration tasks with sparse or deceptive rewards and large state spaces. These challenges severely limit the practical application of DRL. Most previous exploration methods relied on complex architectures to estimate state novelty or introduced sensitive hyperparameters, resulting in instability. To mitigate these issues, we propose an efficient adaptive trajectory -constrained exploration strategy for DRL. The proposed method guides the agent's policy away from suboptimal solutions by regarding previous offline demonstrations as references. Specifically, this approach gradually expands the exploration scope of the agent and strives for optimality in a constrained optimization manner. Additionally, we introduce a novel policy -gradient -based optimization algorithm that utilizes adaptive clipped trajectory -distance rewards for both singleand multi -agent reinforcement learning. We provide a theoretical analysis of our method, including a deduction of the worst -case approximation error bounds, highlighting the validity of our approach for enhancing exploration. To evaluate the effectiveness of the proposed method, we conducted experiments on two large 2D grid world mazes and several MuJoCo tasks. The extensive experimental results demonstrated the significant advantages of our method in achieving temporally extended exploration and avoiding myopic and suboptimal behaviors in both singleand multi -agent settings. Notably, the specific metrics and quantifiable results further support these findings. The code used in the study is available at https://github.com/buaawgj/TACE.
更多查看译文
关键词
Deep reinforcement learning,Hard-exploration problem,Policy gradient,Offline suboptimal demonstrations
AI 理解论文
溯源树
样例
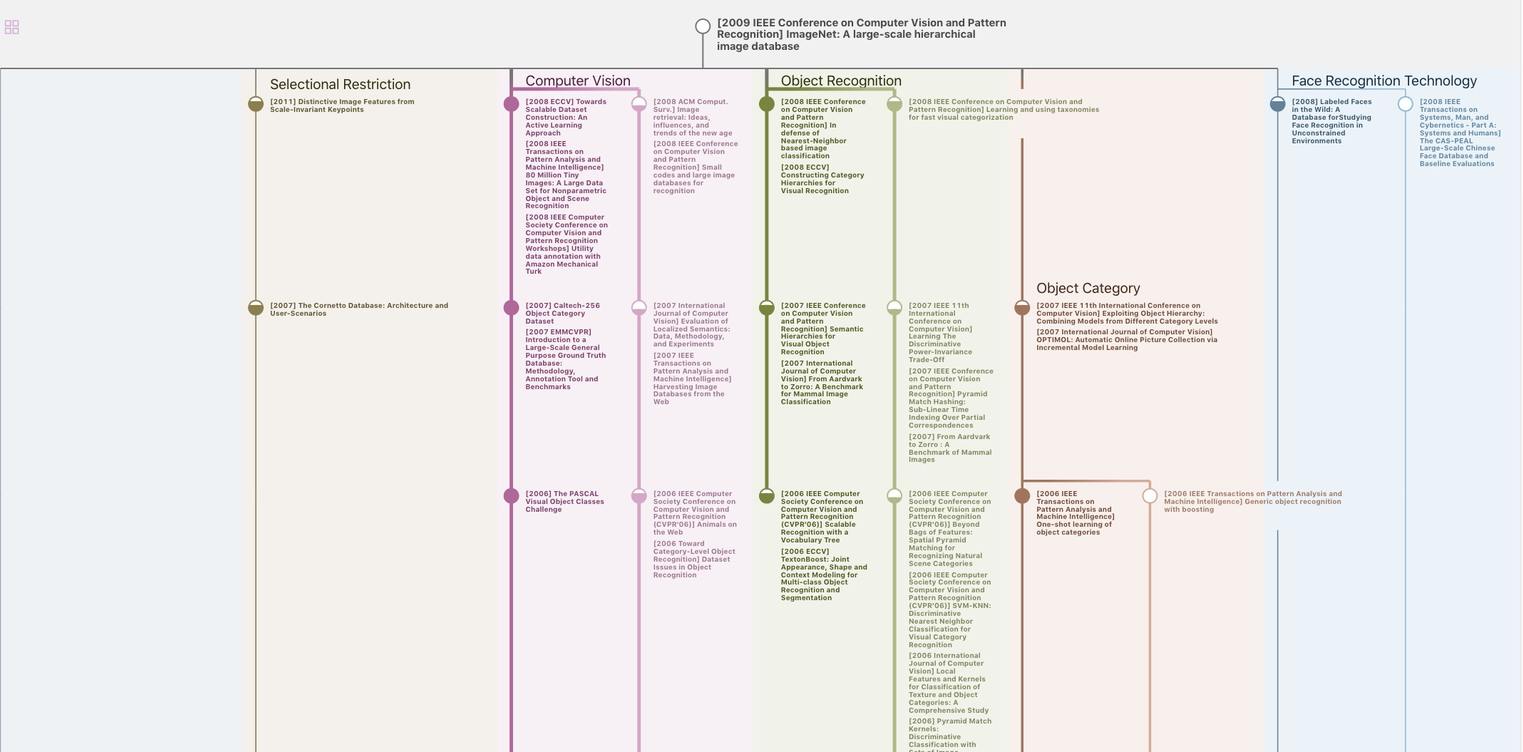
生成溯源树,研究论文发展脉络
Chat Paper
正在生成论文摘要