Transformer Network with Time Prior for Predicting Clinical Outcome from EEG of Cardiac Arrest Patients.
2023 Computing in Cardiology (CinC)(2023)
摘要
The prognosis of neurologic outcome for patients after cardiopulmonary resuscitation is usually made based on morphologic patterns in the electroencephalogram (EEG). However, the specificity of these patterns is comparably low and the dependency on the investigator is high. Hence, features that are instead learned by neural networks are a promising tool to overcome both problems. As part of the George B. Moody PhysioNet Challenge, we propose the convolutional embedding transformer (CET) that learns input features with a feature encoder and applies a time prior in the cost function to enhance the training process and enable the model to learn more generalized internal feature representations. The input of CET is the raw, downsampled data. For comparison we implement a support vector machine (SVM) model that uses the features based on morphologic patterns. In a 5 fold cross-validation on the training data, CET achieves a mean challenge score of 0.41 and AUPRC of 0.84. The model did not achieve a score on the test set during the official phase (team “IWill-Survive”).
更多查看译文
AI 理解论文
溯源树
样例
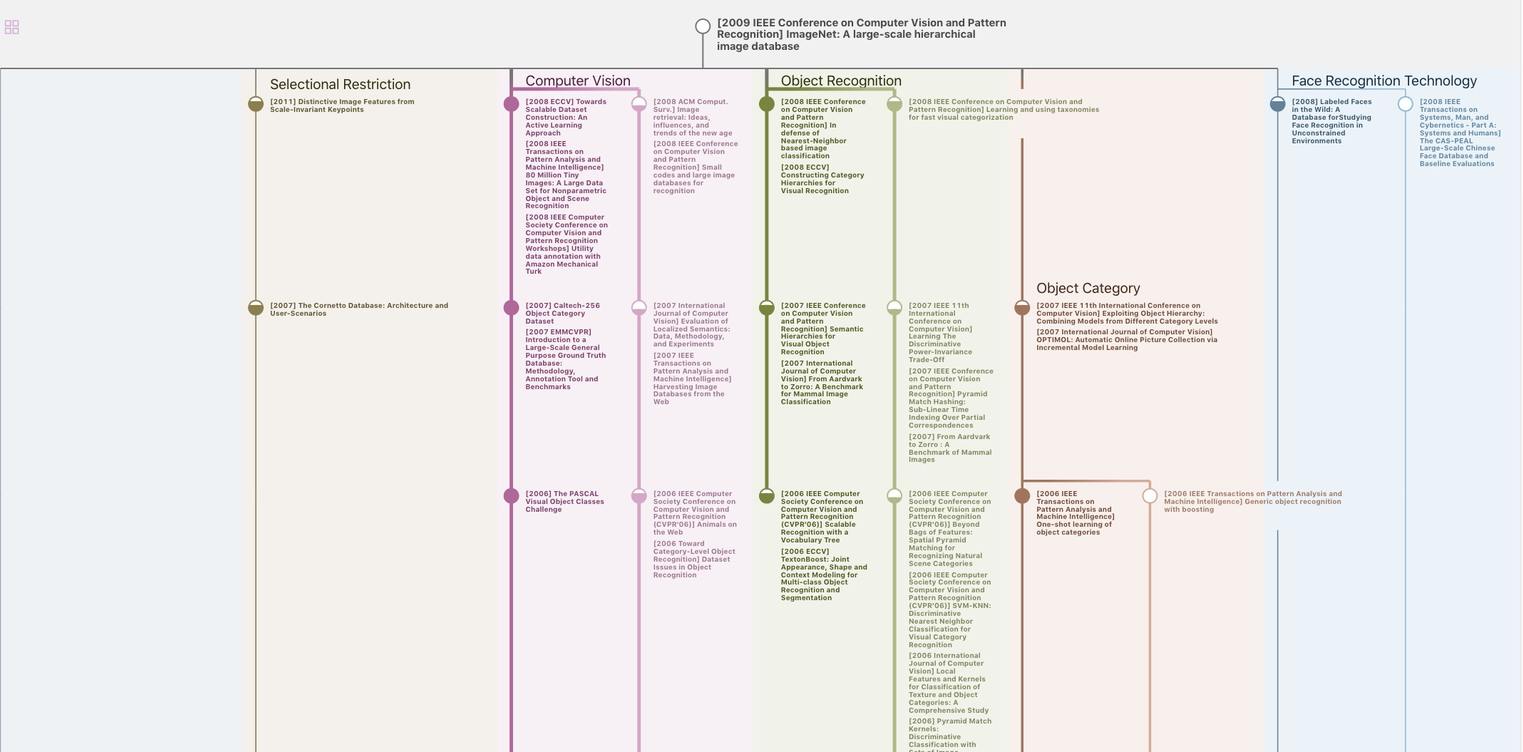
生成溯源树,研究论文发展脉络
Chat Paper
正在生成论文摘要