TEILP: Time Prediction over Knowledge Graphs via Logical Reasoning
AAAI 2024(2024)
摘要
Conventional embedding-based models approach event time prediction in temporal knowledge graphs (TKGs) as a ranking problem. However, they often fall short in capturing essential temporal relationships such as order and distance. In this paper, we propose TEILP, a logical reasoning framework that naturaly integrates such temporal elements into knowledge graph predictions. We first convert TKGs into a temporal event knowledge graph (TEKG) which has a more explicit representation of time in term of nodes of the graph. The TEKG equips us to develop a differentiable random walk approach to time prediction. Finally, we introduce conditional probability density functions, associated with the logical rules involving the query interval, using which we arrive at the time prediction. We compare TEILP with state-of-the-art methods on five benchmark datasets. We show that our model achieves a significant improvement over baselines while providing interpretable explanations. In particular, we consider several scenarios where training samples are limited, event types are imbalanced, and forecasting the time of future events based on only past events is desired. In all these cases, TEILP outperforms state-of-the-art methods in terms of robustness.
更多查看译文
关键词
ML: Neuro-Symbolic Learning,ML: Statistical Relational/Logic Learning,DMKM: Rule Mining & Pattern Mining,KRR: Logic Programming
AI 理解论文
溯源树
样例
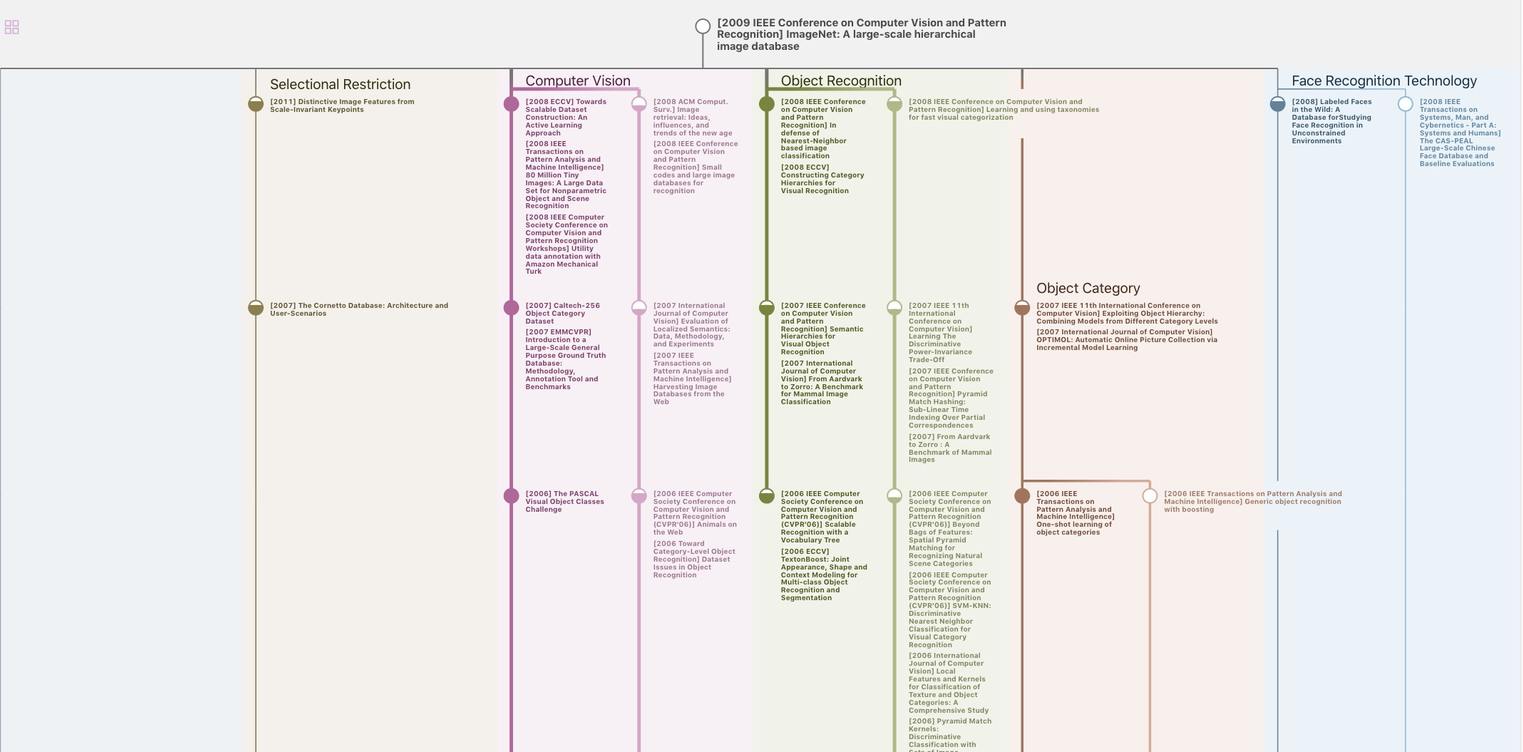
生成溯源树,研究论文发展脉络
Chat Paper
正在生成论文摘要