An extended asymmetric sigmoid with Perceptron (SIGTRON) for imbalanced linear classification
CoRR(2023)
摘要
This article presents a new polynomial parameterized sigmoid called SIGTRON,
which is an extended asymmetric sigmoid with Perceptron, and its companion
convex model called SIGTRON-imbalanced classification (SIC) model that employs
a virtual SIGTRON-induced convex loss function. In contrast to the conventional
π-weighted cost-sensitive learning model, the SIC model does not have an
external π-weight on the loss function but has internal parameters in the
virtual SIGTRON-induced loss function. As a consequence, when the given
training dataset is close to the well-balanced condition, we show that the
proposed SIC model is more adaptive to variations of the dataset, such as the
inconsistency of the scale-class-imbalance ratio between the training and test
datasets. This adaptation is achieved by creating a skewed hyperplane equation.
Additionally, we present a quasi-Newton optimization(L-BFGS) framework for the
virtual convex loss by developing an interval-based bisection line search.
Empirically, we have observed that the proposed approach outperforms
π-weighted convex focal loss and balanced classifier LIBLINEAR(logistic
regression, SVM, and L2SVM) in terms of test classification accuracy with 51
two-class and 67 multi-class datasets. In binary classification problems,
where the scale-class-imbalance ratio of the training dataset is not
significant but the inconsistency exists, a group of SIC models with the best
test accuracy for each dataset (TOP1) outperforms LIBSVM(C-SVC with RBF
kernel), a well-known kernel-based classifier.
更多查看译文
AI 理解论文
溯源树
样例
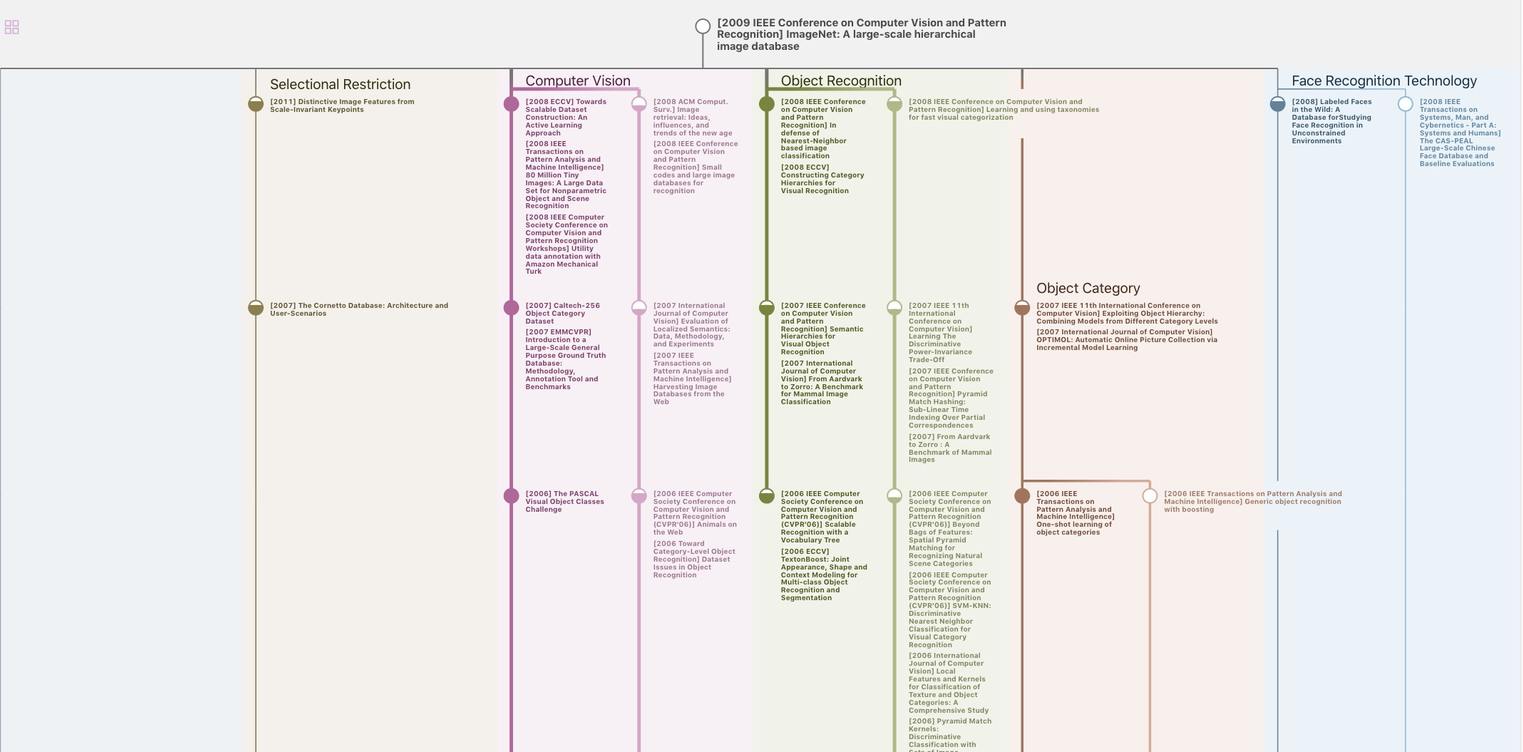
生成溯源树,研究论文发展脉络
Chat Paper
正在生成论文摘要