Federated-Edge Computing Based Cyber-Physical Systems Framework for Enhanced Diabetes Management.
2023 15th International Conference on Innovations in Information Technology (IIT)(2023)
摘要
This paper presents an intelligent cyber-physical system framework for detecting and managing type 2 diabetes. The framework leverages the benefits of cloud and federated-edge computing while addressing concerns of security, delay, and communication costs. It enables the seamless integration of knowledge extracted from machine learning (ML) models trained at the edges into a CNN model using distillation and ensemble techniques. To identify the effective model for detecting diabetes, we performed a comparative study using four different ML classifiers: Support Vector Machine (SVM), Artificial Neural Network (ANN), Case-Based Reasoning with Fuzzy K-Nearest Neighbor (CBR-FKNN), and K-means with Logistic Regression (K-Means-LR) at a single edge. We employed various preprocessing techniques and feature selection algorithms to identify the optimal features for the four models. An experimental evaluation was conducted using two diabetes datasets, the PIMA dataset and the Diabetes dataset 2019. The experiments were mainly conducted to assess our proposed framework but not the datasets. The results demonstrated that the SVM model outperforms other models in diabetes detection. The results also showed that the Diabetes dataset 2019 provided better accuracy and F1 scores than the PIMA dataset.
更多查看译文
关键词
diabetes,machine learning,federated learning,edge computing,CPS
AI 理解论文
溯源树
样例
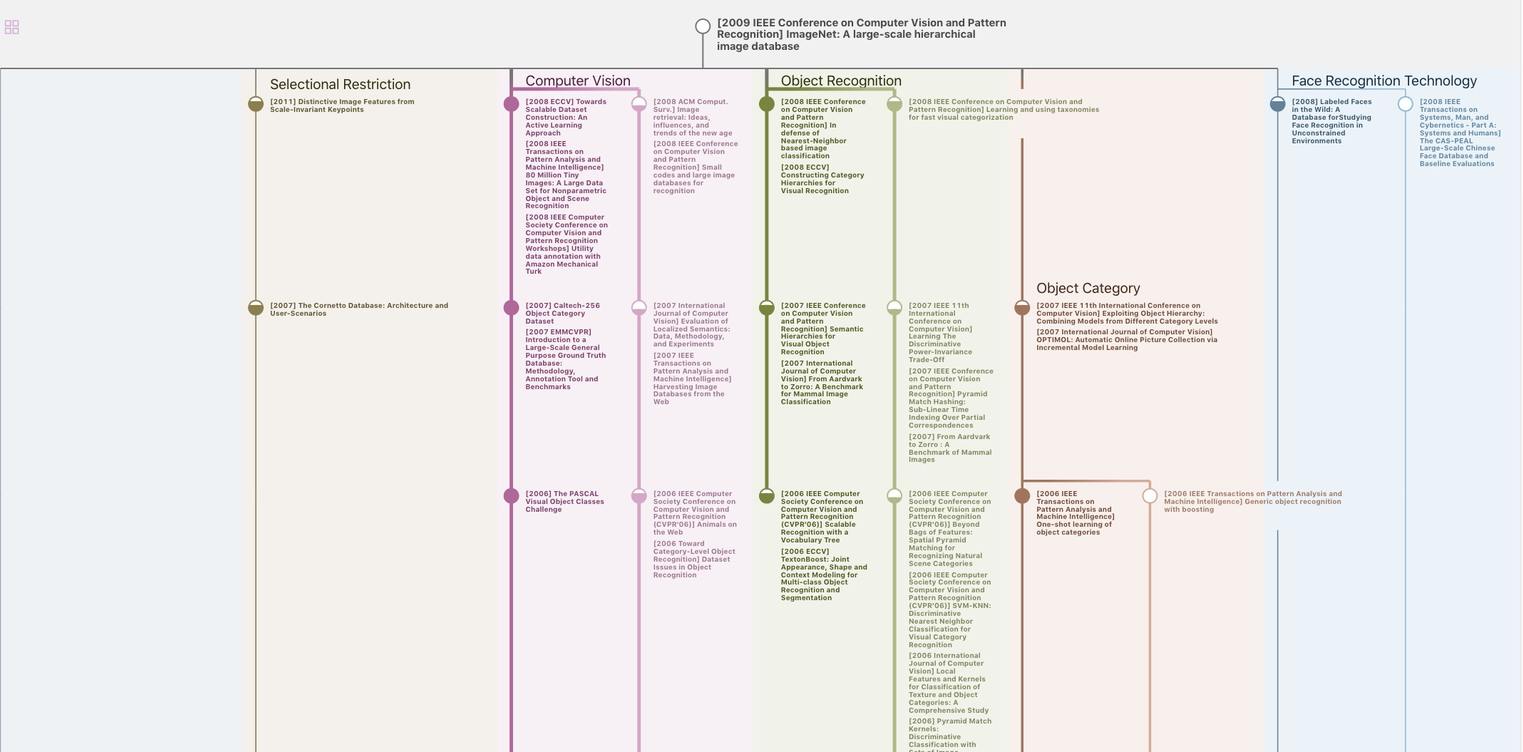
生成溯源树,研究论文发展脉络
Chat Paper
正在生成论文摘要