A Regression Analysis Approach for Feature Selection in Student Achievement Data.
2023 15th International Conference on Innovations in Information Technology (IIT)(2023)
摘要
TIMSS is a global standardized assessment of mathematics and science knowledge, skills, and achievement of students in grades four and eight. The data generated from the 64 countries and 8 benchmarking entities participated in TIMSS 2019 is enormous and worth exploring and analyzing. This work aims to identify the significant factors that affect students’ scores in mathematics in Grade 8 in the United Arab Emirates (UAE), using the data exported from the TIMSS 2019 dataset. Towards this, we leverage Linear Regression modeling, together with backward elimination techniques with the objective of: 1) maximizing the accuracy of predicting student performance, and 2) minimizing the number of identified significant features that contribute to student performance. Out of the hundreds of features (i.e., variables) collected in the TIMSS dataset, our experimental evaluation identified 17 features as the most significant in affecting student performance. Those identified features span multiple categories, including variables related to the categories of: student (9 features), home (3 features), teacher (2 features), classroom (1 feature), and use of technology (2 features).
更多查看译文
关键词
TIMSS,Regression,Feature Selection
AI 理解论文
溯源树
样例
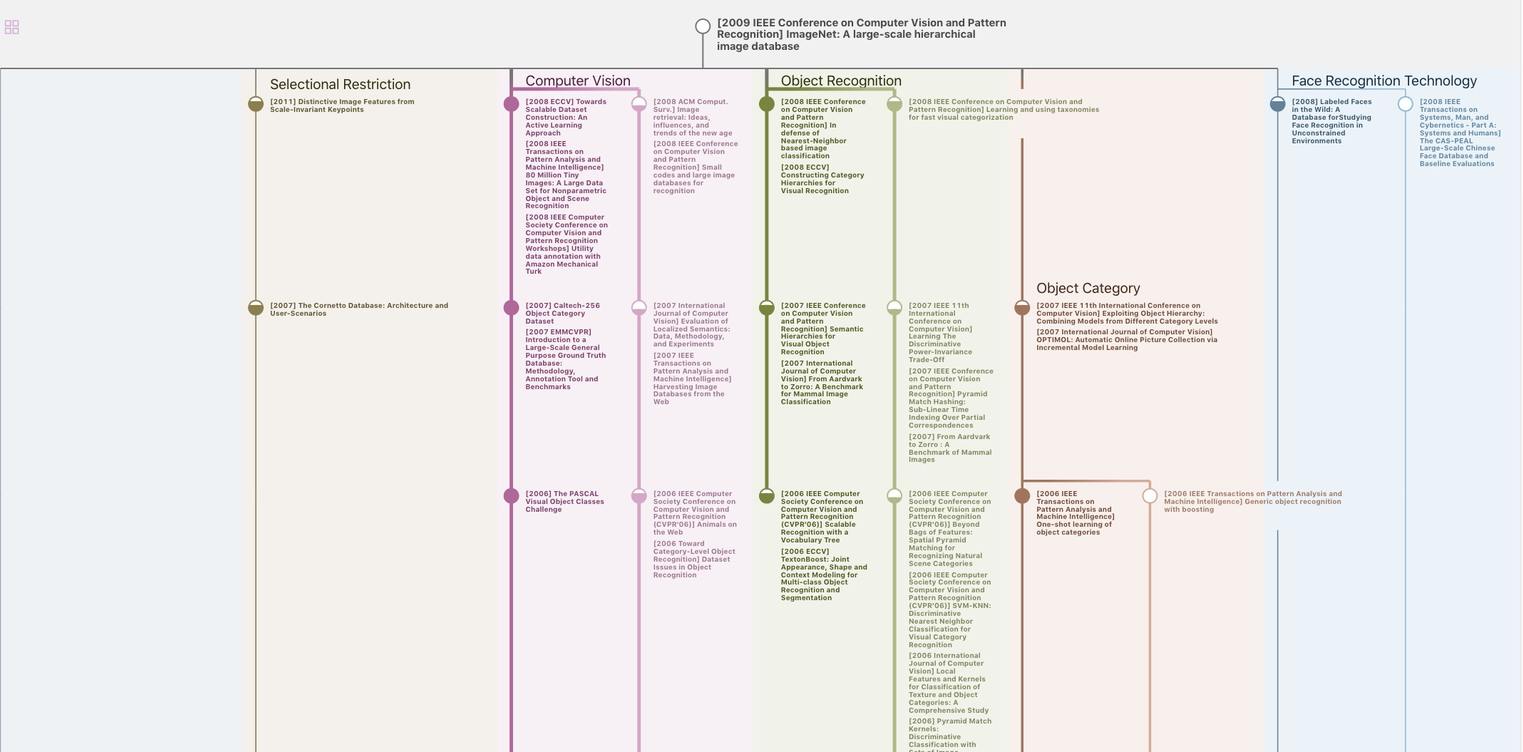
生成溯源树,研究论文发展脉络
Chat Paper
正在生成论文摘要