A Medical Image Fusion Method Based on Detail Enhancement Decomposition.
2023 IEEE Intl Conf on Dependable, Autonomic and Secure Computing, Intl Conf on Pervasive Intelligence and Computing, Intl Conf on Cloud and Big Data Computing, Intl Conf on Cyber Science and Technology Congress (DASC/PiCom/CBDCom/CyberSciTech)(2023)
摘要
The fusion technology of multimodal medical images is an important branch of image fusion, which involves merging a set of biomedical images from two or more different image modalities into another biomedical image using a specific approach. The resulting fused medical images offer greater completeness and richness in terms of information, texture structure, and lesion features compared to traditional single-modal fused images, thus significantly aiding clinicians in improving clinical diagnosis and disease analysis. However, existing medical image fusion algorithms have some issues, such as contrast reduction, blurring of edge details and textures, and color distortion. To address these problems, we propose an image decomposition method based on a detail enhancement model. This method decomposes the medical image into detail and base layers, enhancing texture details by introducing a first-order derivative filter, and smoothing the decomposed layers using a second-order Laplace filter to emphasize image details and resolve blurriness and unclear edges. Furthermore, the authors present a medical image fusion algorithm based on an improved detail-enhanced decomposition model. Targeted fusion rules are proposed for the decomposed layers, incorporating local norms and maximum singular values. By fusing the base and detail layers, the algorithm successfully addresses typical issues such as contrast reduction, detail blurring, and color distortion. Experimental results demonstrate that the algorithm excels in terms of computational speed and visual evaluation compared to traditional algorithms.
更多查看译文
关键词
Image Decomposition,Filters,Local Paradigm,Maximum Singular Value
AI 理解论文
溯源树
样例
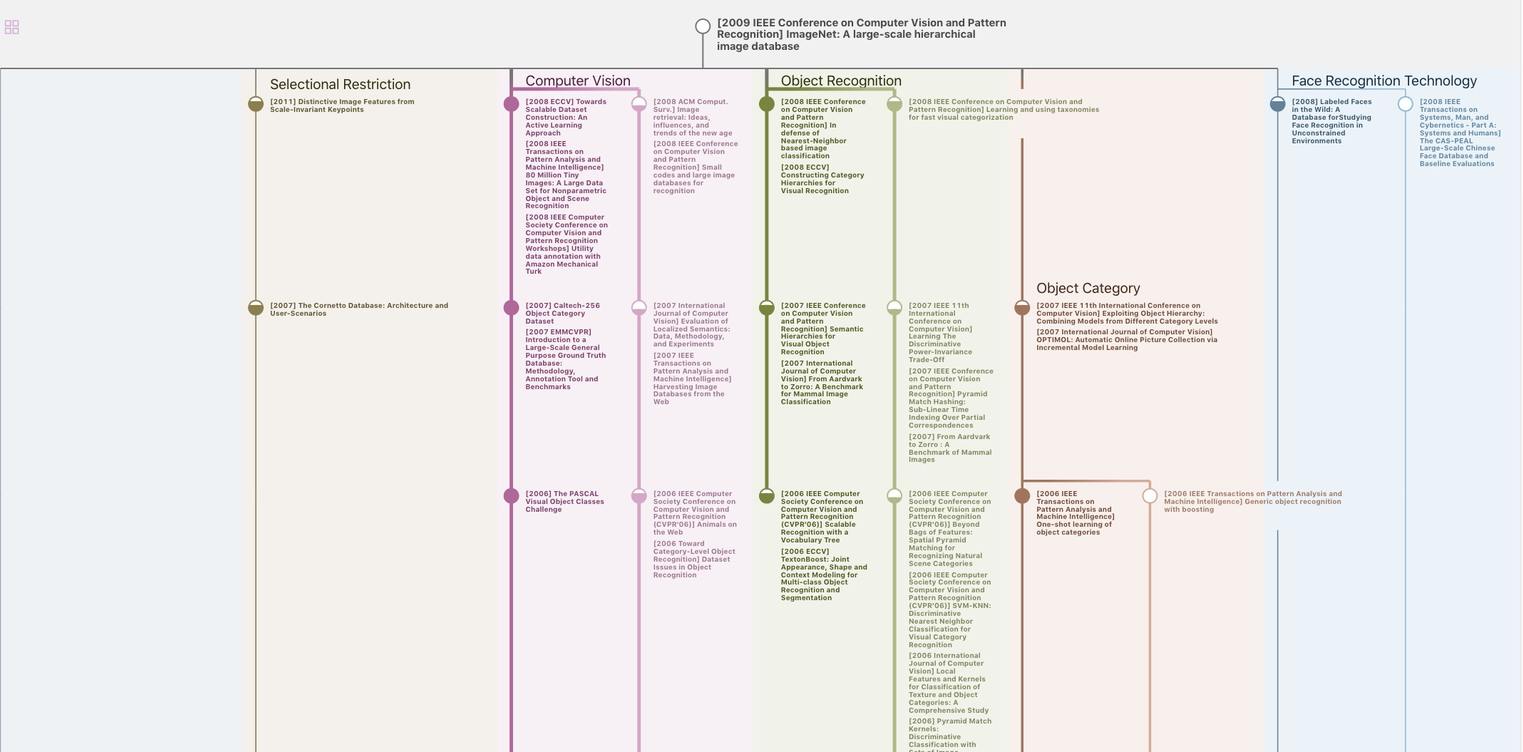
生成溯源树,研究论文发展脉络
Chat Paper
正在生成论文摘要