Robust Object Detection Based on a Comparative Learning Perspective.
2023 IEEE Intl Conf on Dependable, Autonomic and Secure Computing, Intl Conf on Pervasive Intelligence and Computing, Intl Conf on Cloud and Big Data Computing, Intl Conf on Cyber Science and Technology Congress (DASC/PiCom/CBDCom/CyberSciTech)(2023)
摘要
With the advent of adversarial samples, the safety of Artificial Intelligence is of particular concern, as only a small amount of perturbation can be added to mislead a model's judgment. Therefore there is an urgent need for research on models that can resist adversarial perturbations. To alleviate this problem, we first analyze the vulnerability of adversarial samples and propose a uniform perspective robust model for object detection that accurately identifies both clean and ad-versarial samples. We propose a robust object detection based on the contrastive learning perspective (RCP), which can learn features from both clean and adversarial samples from a fine-grained perspective, and can recognize adversarial samples more accurately. Extensive experiments on PASCAL VOC and MS COCO show that our proposed method only degrades the clean sample detection performance by a small amount in exchange for a large robustness improvement against adversarial attacks, achieving state of the art results.
更多查看译文
关键词
Artificial intelligence security,Object detection,Adversarial attacks,Adversarial defense
AI 理解论文
溯源树
样例
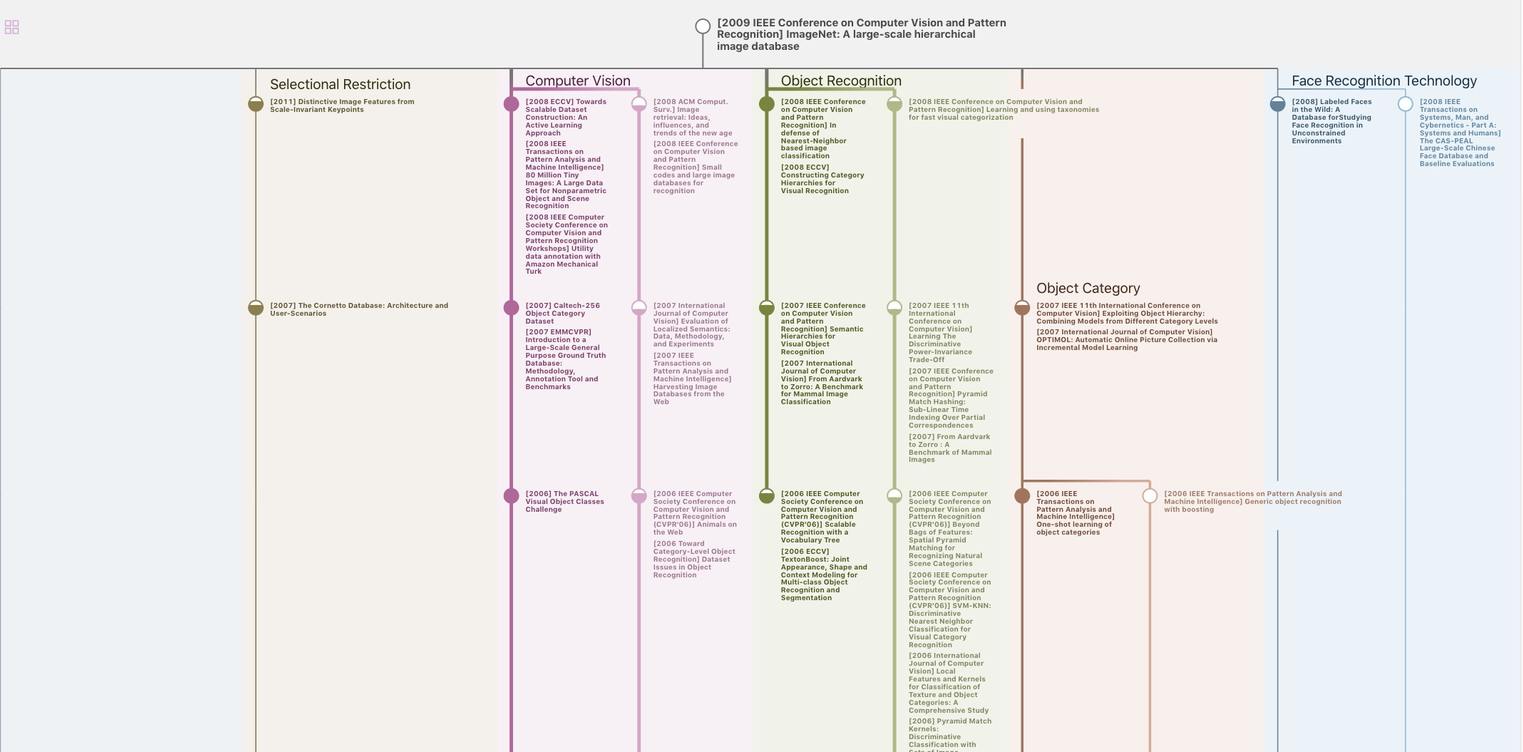
生成溯源树,研究论文发展脉络
Chat Paper
正在生成论文摘要