A Model for Cognitively Valid Lifelong Learning.
2023 IEEE International Conference on Robotics and Biomimetics (ROBIO)(2023)
摘要
In continual learning, usually a sequence of tasks are given to a learning agent and the performance of the agent after learning is measured in terms of resistance to catastrophic forgetting, efficacy of knowledge transfer and overall performance on the individual tasks. On the other hand, in multi-task learning, the system is designed to simultaneously acquire knowledge in multiple tasks, often through offline batch learning. A more cognitively valid scenario for lifelong robot learning would be to have a robotic agent to autonomously decide which task to engage and disengage while leveraging many-to-many knowledge transfer ability among tasks during online learning. In this study, we propose a novel lifelong robot learning architecture to fulfill the aforementioned desiderata, and show its validity in an environment where a robot learns the effects of its actions in different task settings. To realize the proposed model, we adopt learning progress measure for task selection, and have the tasks learn by independent neural networks with special structure that allows access to the neural layers of the non-selected tasks. The experiments conducted with a simulated robot arm in an object interaction scenario show that the proposed architecture yields better knowledge transfer and facilitates faster learning compared to baselines of fixed sequence task learning and isolated task learners with no knowledge transfer.
更多查看译文
AI 理解论文
溯源树
样例
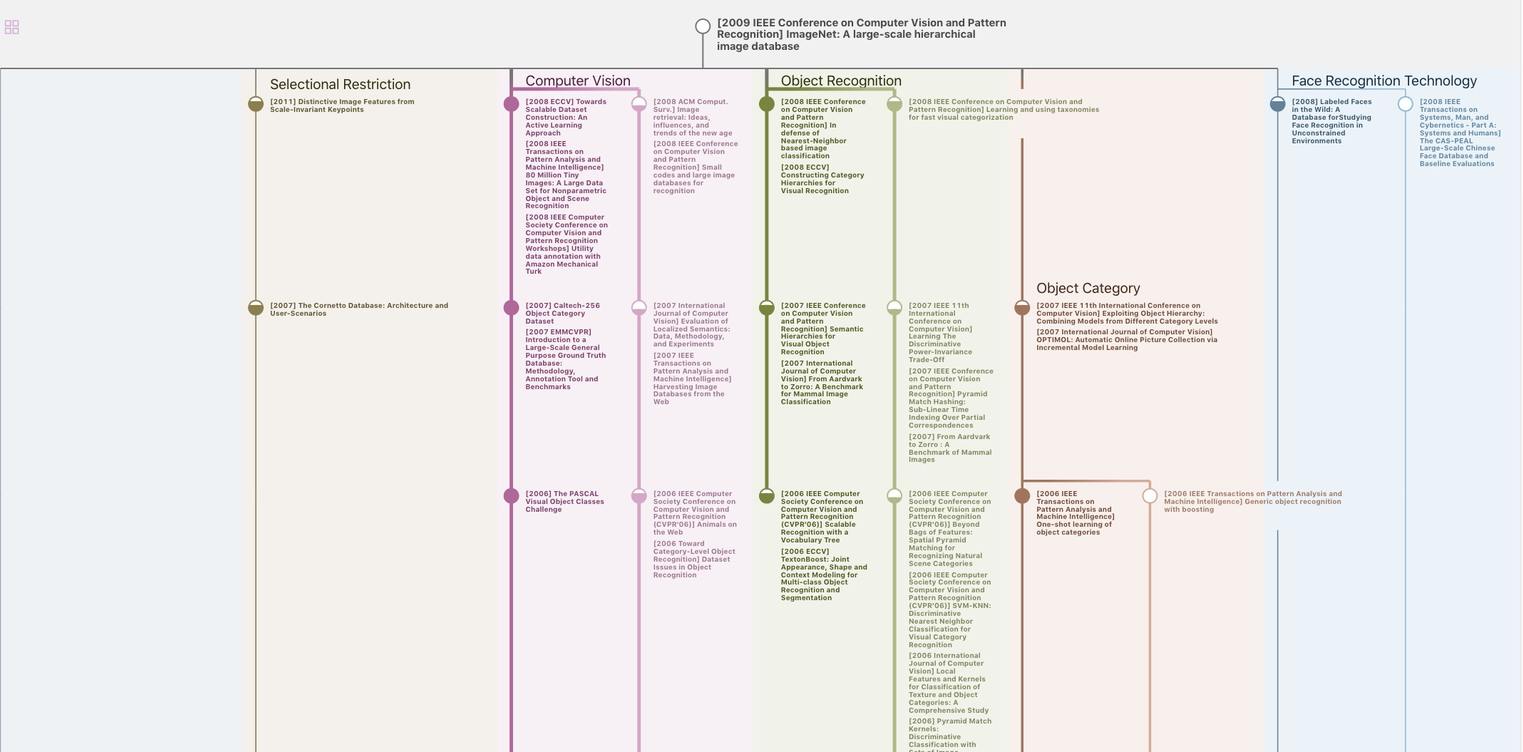
生成溯源树,研究论文发展脉络
Chat Paper
正在生成论文摘要