A Compressed and Accurate Sparse Deep Learning-based Workload-Aware Timing Error Model
2023 IEEE 41ST INTERNATIONAL CONFERENCE ON COMPUTER DESIGN, ICCD(2023)
Abstract
This paper showcases the novel application of Deep-Learning (DL) in the development of accurate microarchitecture and workload-aware timing error models and investigates methods such as sparsification for reducing their complexity, while maintaining high accuracy. Our study shows that DL can help increase the accuracy and true positive rate (TPR) of workload-aware models for a pipelined floating-point core compared to existing models. In addition, we demonstrate that removing up to 40% of the total neurons has minimal impact on the accuracy and overall predictive performance (up to 2.2%) of our DL-based timing error models, while significantly reducing the computational complexity. In fact, the complexity of the sparse model is approximately 2x smaller than the dense one.
MoreTranslated text
AI Read Science
Must-Reading Tree
Example
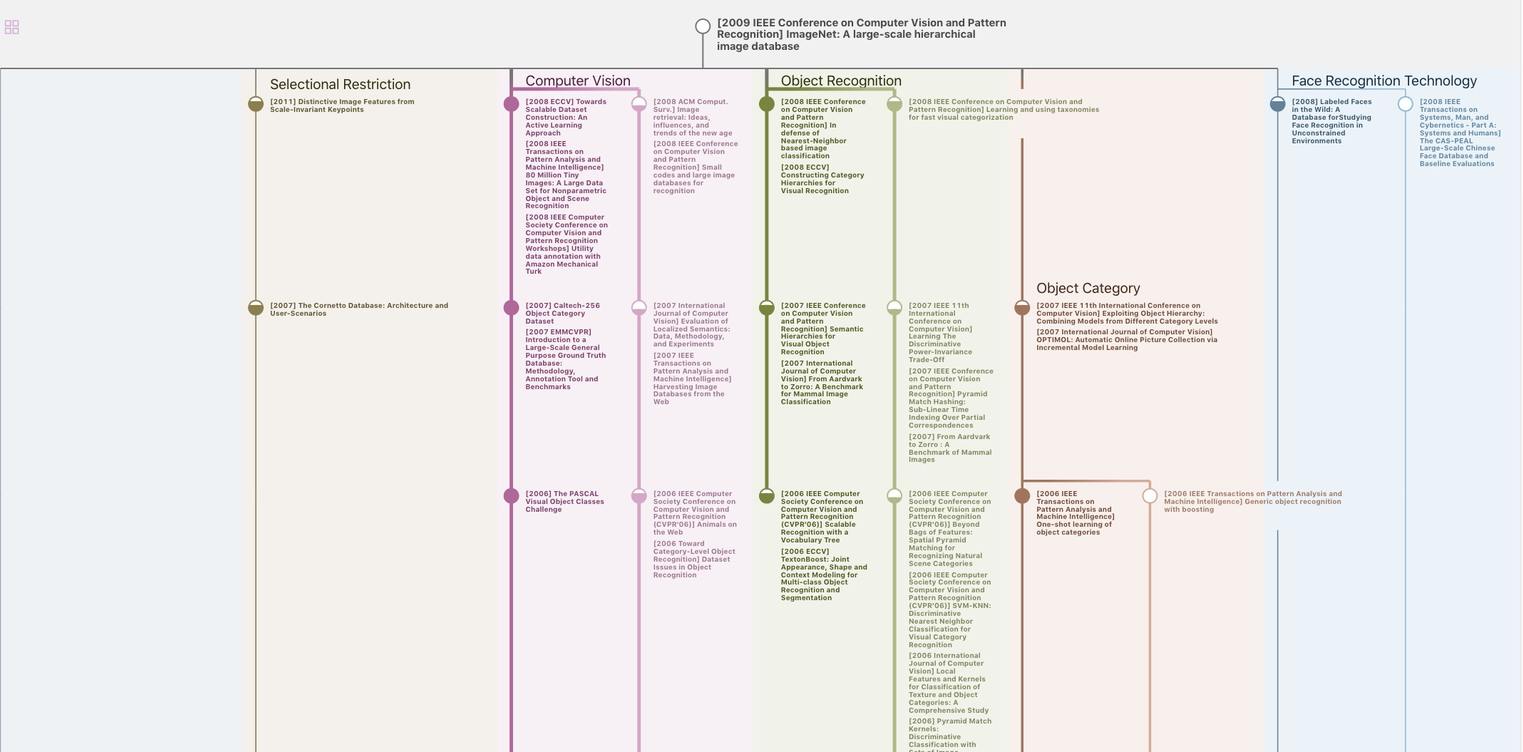
Generate MRT to find the research sequence of this paper
Chat Paper
Summary is being generated by the instructions you defined