Representation learning: serial-autoencoder for personalized recommendation
Frontiers of Computer Science(2023)
摘要
Nowadays, the personalized recommendation has become a research hotspot for addressing information overload. Despite this, generating effective recommendations from sparse data remains a challenge. Recently, auxiliary information has been widely used to address data sparsity, but most models using auxiliary information are linear and have limited expressiveness. Due to the advantages of feature extraction and no-label requirements, autoencoder-based methods have become quite popular. However, most existing autoencoder-based methods discard the reconstruction of auxiliary information, which poses huge challenges for better representation learning and model scalability. To address these problems, we propose Serial-Autoencoder for Personalized Recommendation (SAPR), which aims to reduce the loss of critical information and enhance the learning of feature representations. Specifically, we first combine the original rating matrix and item attribute features and feed them into the first autoencoder for generating a higher-level representation of the input. Second, we use a second autoencoder to enhance the reconstruction of the data representation of the prediciton rating matrix. The output rating information is used for recommendation prediction. Extensive experiments on the MovieTweetings and MovieLens datasets have verified the effectiveness of SAPR compared to state-of-the-art models.
更多查看译文
关键词
personalized recommendation,autoencoder,representation learning,collaborative filtering
AI 理解论文
溯源树
样例
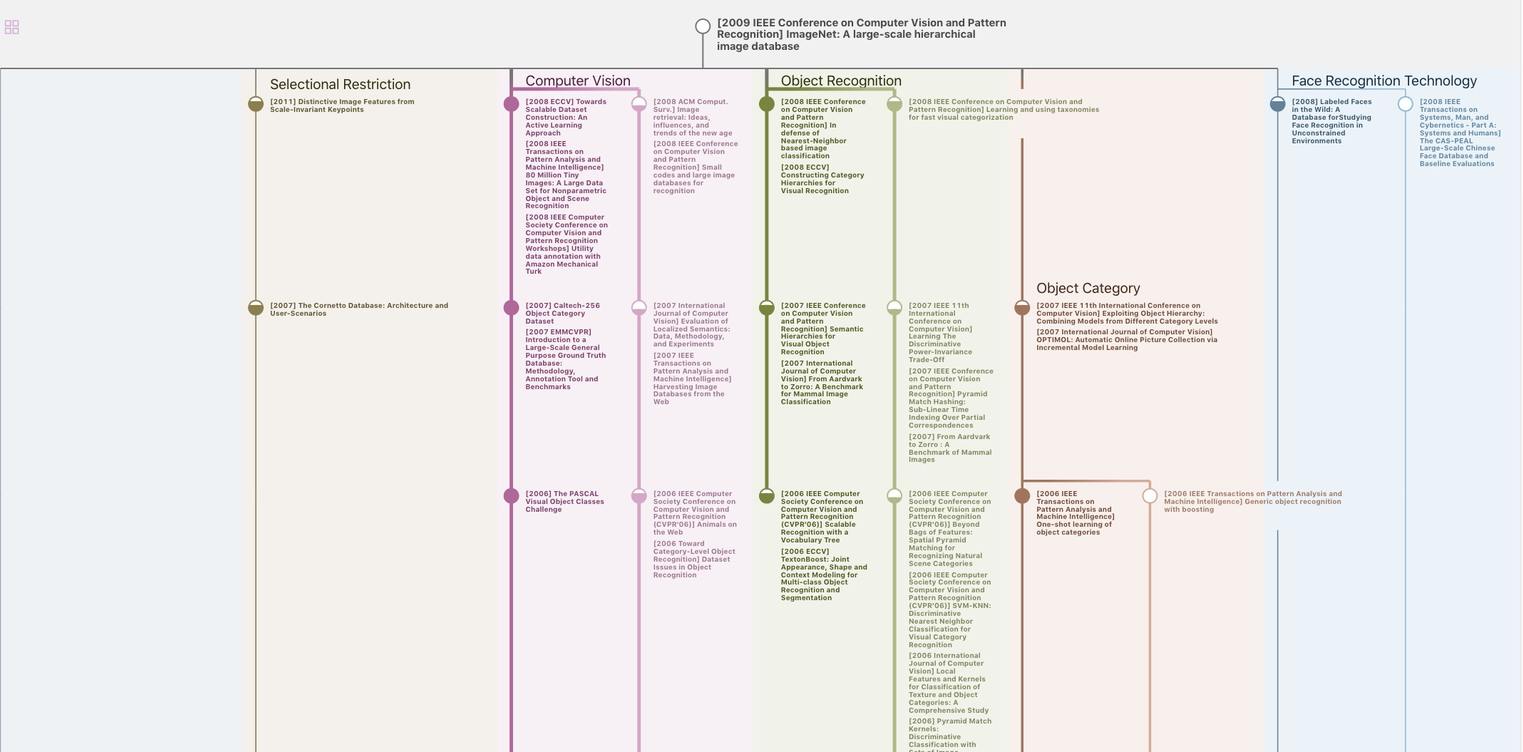
生成溯源树,研究论文发展脉络
Chat Paper
正在生成论文摘要