A Meta-Heuristic Sustainable Intelligent Internet of Things Framework for Bearing Fault Diagnosis of Electric Motor under Variable Load Conditions
SUSTAINABILITY(2023)
摘要
The study introduces an Intelligent Diagnosis Framework (IDF) optimized using the Grasshopper Optimization Algorithm (GOA), an advanced swarm intelligence method, to enhance the precision of bearing defect diagnosis in electrical machinery. This area is vital for the energy sector and IoT manufacturing, but the evolving designs of electric motors add complexity to fault identification. Machine learning offers potential solutions but faces challenges due to computational intensity and the need for fine-tuning hyperparameters. The optimized framework, named GOA-IDF, is rigorously tested using experimental bearing fault data from the CWRU database, focusing on the 12,000 drive end and fan end datasets. Compared to existing machine learning algorithms, GOA-IDF shows superior diagnostic capabilities, especially in processing high-frequency data that are susceptible to noise interference. This research confirms that GOA-IDF excels in accurately categorizing faults and operates with increased computational efficiency. This advancement is a significant contribution to fault diagnosis in electrical motors. It suggests that integrating intelligent frameworks with meta-heuristic optimization techniques can greatly improve the standards of health monitoring and maintenance in the electrical machinery domain.
更多查看译文
关键词
machine learning,bearing fault,intelligent framework,electric motor,variable load conditions
AI 理解论文
溯源树
样例
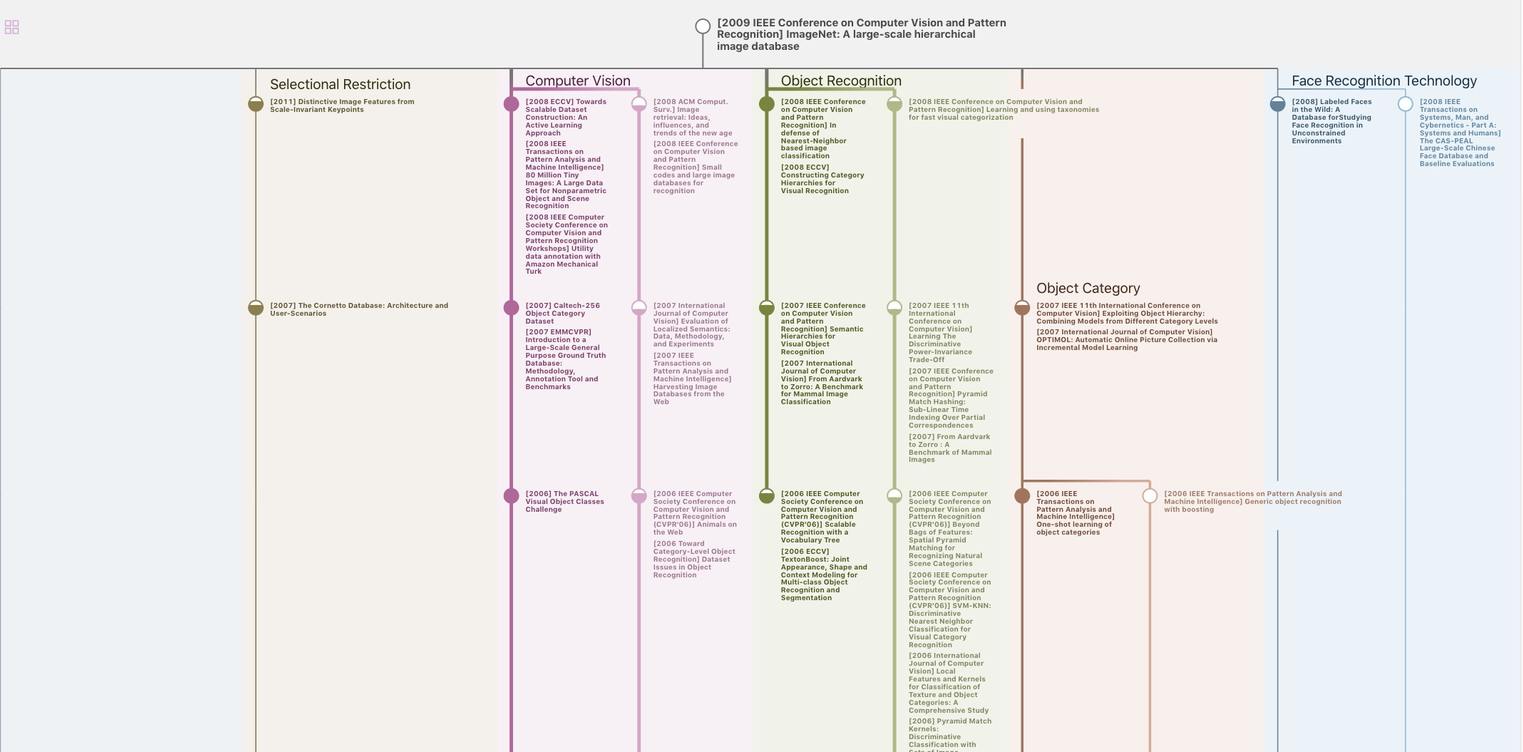
生成溯源树,研究论文发展脉络
Chat Paper
正在生成论文摘要