A data-efficient visual analytics method for human-centered diagnostic systems to endoscopic ultrasonography
Applied Intelligence(2023)
摘要
Endoscopic ultrasound (EUS) has emerged as a pivotal tool for the screening and diagnosis of submucosal tumors (SMTs). However, the inherently low-quality and highly variable image content presents substantial obstacles to the automation of SMT diagnosis. Deep learning, with its adaptive feature extraction capabilities, offers a potential solution, yet its implementation often requires a vast quantity of high-quality data - a challenging prerequisite in clinical settings. To address this conundrum, this paper proposes a novel data-efficient visual analytics method that integrates human feedback into the model lifecycle, thereby augmenting the practical utility of data. The methodology leverages a two-stage deep learning algorithm, which encompasses self-supervised pre-training and an attention-based network. Comprehensive experimental validation reveals that the proposed approach facilitates the model in deciphering the hierarchical structure information within high-noise EUS images. Moreover, when allied with human-machine interaction, it enhances data utilization, thereby elevating the accuracy and reliability of diagnostic outcomes. The code is available at https://github.com/Zehebi29/LA-RANet.git .
更多查看译文
关键词
Visual analytics,Human-centered,Endoscopic ultrasound,Self-supervised,Attention mechanism
AI 理解论文
溯源树
样例
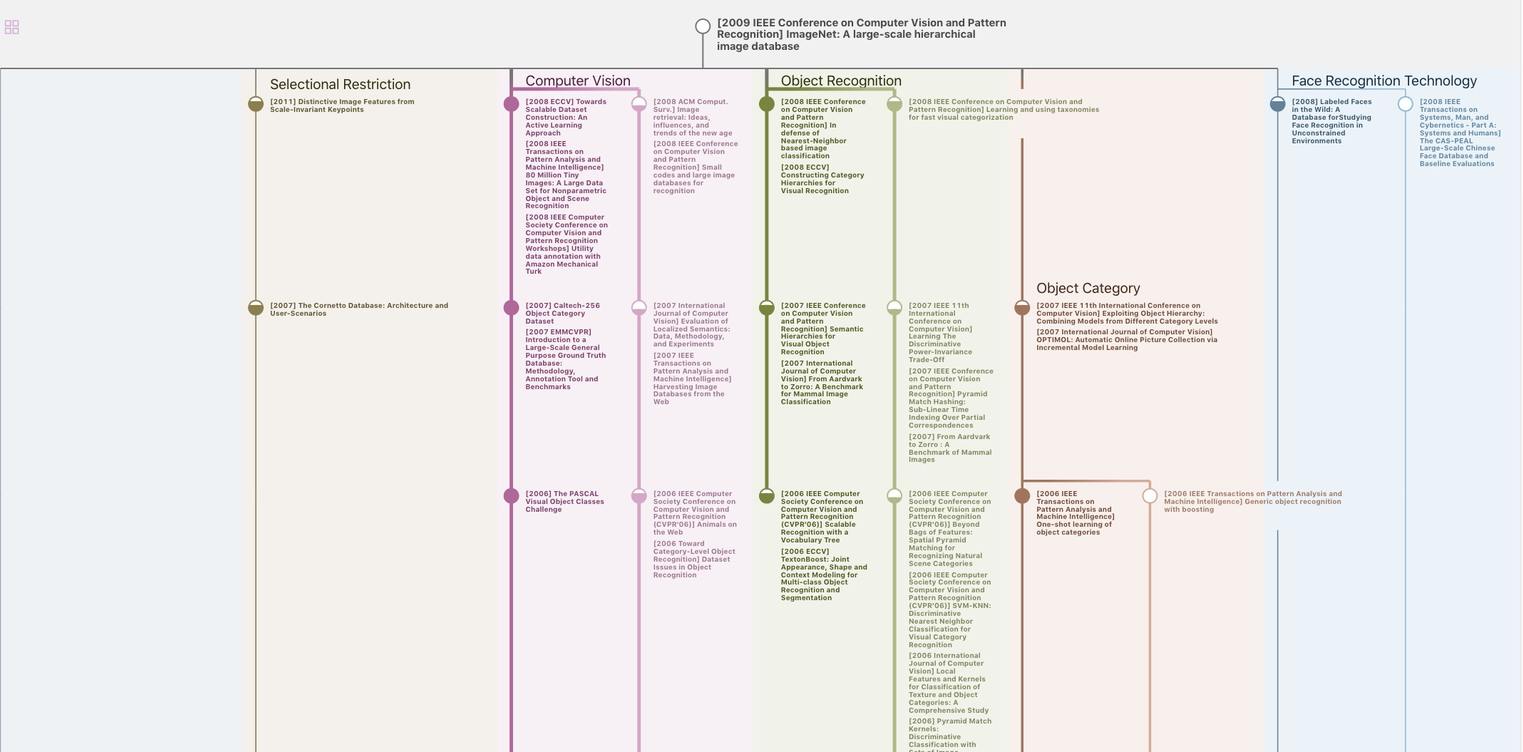
生成溯源树,研究论文发展脉络
Chat Paper
正在生成论文摘要