Response of an Euler–Bernoulli beam subject to a stochastic disturbance
Engineering with Computers(2023)
摘要
This work concerns the stochastic analysis of the bending of a slender cantilever beam subject to an external force with the inclusion of a stochastic effect characterised by white noise. The beam deflection is governed by the classic dynamic Euler–Bernoulli equation. Its response to the stochastic external load is investigated by learning pattern from the simulation data which are collected from numerical computations of ten thousand numerical experiments, which are achieved using a finite difference method coupled with a Monte Carlo method for the uncertainty quantification. Insightful results are presented with visualisation techniques and discussed in detail. Of note, by performing regression analysis to the data, the solution is shown to follow a centred Gaussian process with a strong numerical evidence. The associated autocovariance matrix is computed using the sample data. Then, a mild solution in the probability sense for the deflection at a fixed position and a fixed time is written explicitly in a simple form. The results obtained by the finite difference scheme were also compared to the finite-element scheme and were found to be in good agreement. Unsurprisingly, the finite-element scheme was found to be much more computationally expensive compared to finite difference scheme. Hence, for such a simple structure, the stochastic analysis using the finite difference scheme is preferred. Analysis of the results also showed that some of the regression parameters converge when the number of simulations reaches five hundred and only vary subject to numerical errors of order 10^-6 if the number of simulations is further increased. While others converge when the number of simulations exceeds two thousand showing that depending on the level of precision required fewer than ten thousand simulations might be required.
更多查看译文
关键词
Euler-Bernoulli beam,Stochastic partial differential equation,Finite difference,Monte Carlo simulation,Stochastic process,Regression,Machine learning,Visualisation
AI 理解论文
溯源树
样例
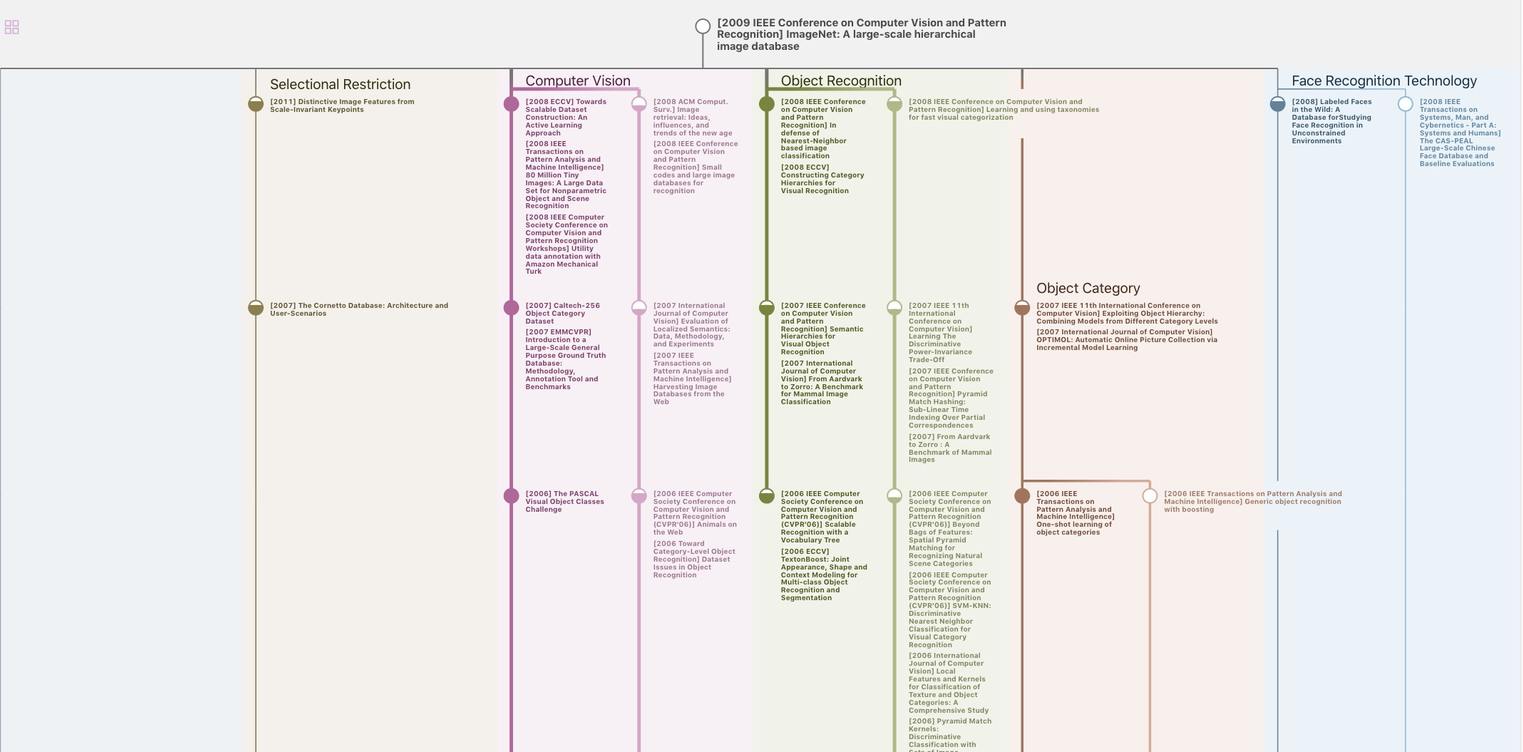
生成溯源树,研究论文发展脉络
Chat Paper
正在生成论文摘要