Graph neural networks for visual question answering: a systematic review
Multimedia Tools and Applications(2023)
摘要
Recently, visual question answering (VQA) has gained considerable interest within the computer vision and natural language processing (NLP) research areas. The VQA task involves answering a question about an image, which requires both language and vision understanding. Effectively extracting visual representations from images, textual embedding from questions, and bridging the semantic disparity between image and question representations pose fundamental challenges in VQA. Lately, an increasing number of studies are focusing on utilizing graph neural networks (GNNs) to enhance the performance of VQA tasks. The ability to handle graph-structured data is a major advantage of GNNs for VQA tasks, which allows better representation of relationships between objects and regions in an image. These relationships include both spatial and semantic relationships. This paper systematically reviews various graph neural networks based studies for image-based VQA. Fifty-four related publications written between 2018—Jan. 2023 were carefully synthesized for this review. The review is structured into three perspectives: the various graph neural network techniques and models that have been applied for VQA, a comparison of the model's performance and existing challenges. After analyzing these papers, 45 different models were identified, grouped into four different GNN techniques. These are Graph Convolution Network (GCN), Graph Attention Network (GAT), Graph Isomorphism Network (GIN) and Graph Neural Network (GNN). Also, the performance of these models is compared based on accuracy, datasets, subtasks, feature representation and fusion techniques. Lastly, the study provided some possible suggestions to mitigate still existing challenges for future research in visual question answering.
更多查看译文
关键词
Graph neural networks,Visual question answering,Computer vision,Natural language processing
AI 理解论文
溯源树
样例
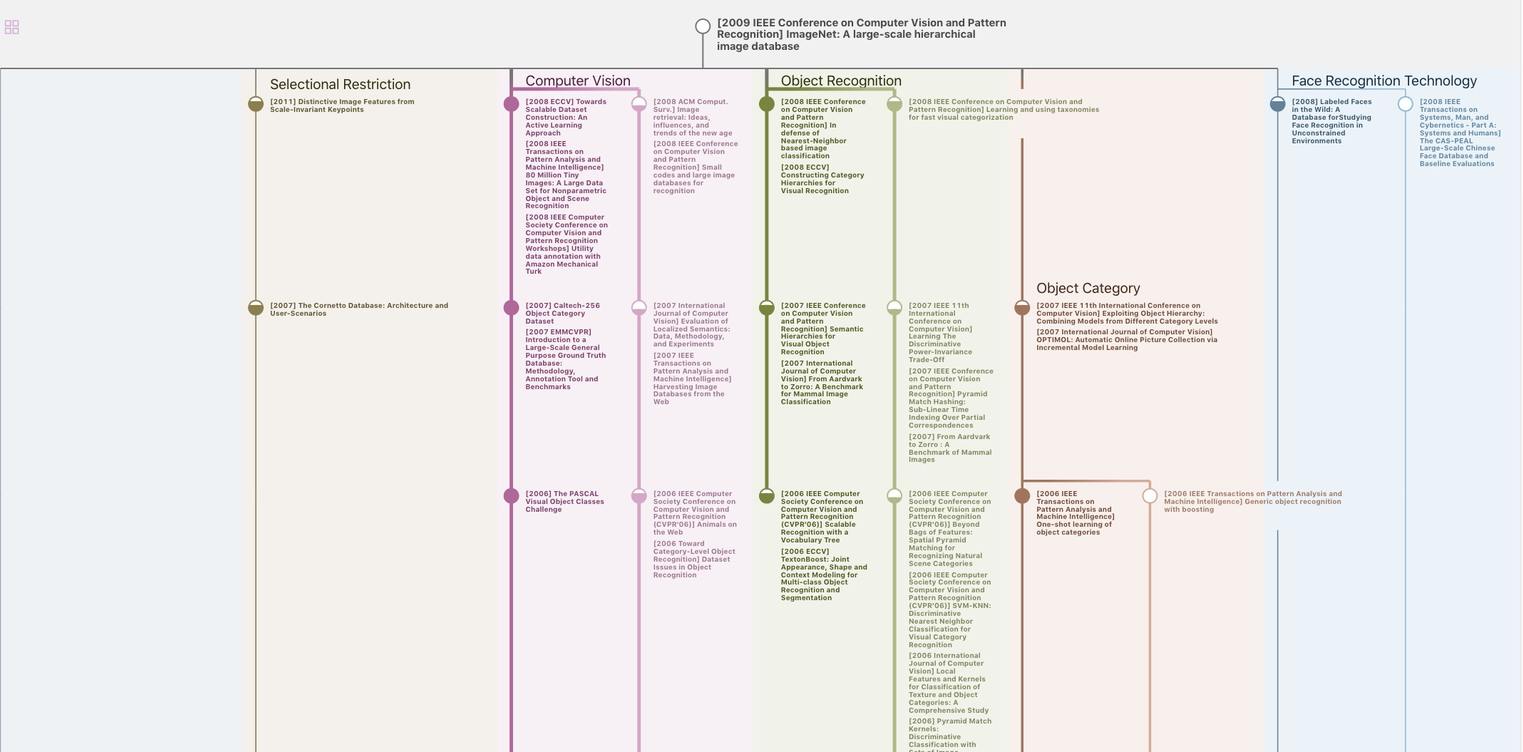
生成溯源树,研究论文发展脉络
Chat Paper
正在生成论文摘要