Boundary-aware small object detection with attention and interaction
The Visual Computer(2023)
摘要
Object detection is a critical technology for the intelligent analytical processing of images captured by drones. The objects usually come in various scales and can be extremely small. Existing detection methods are inherently based on pyramid hierarchy architectures to extract multi-scale features and provide better feature representation for small objects. Nevertheless, they inevitably dilute the representation of details in low-level features during top-down feature fusion and are totally unconcerned with whether the fused feature fits the objects of specific scales within a layer. Moreover, the pyramid can only implicitly fuse the spatial context, which makes the fused features cannot receive fine spatial location information for object localization. In this work, we propose an effective boundary-aware network with attention refinement and spatial interaction to tackle the above challenges. Specifically, we first present a highly effective yet simple boundary-aware detection head (BAH), which directly guides representation learning of object structure semantics in the prediction layer to preserve object-related boundary semantics. Additionally, the attentional feature parallel fusion (AFPF) module offers multi-scale feature encoding capability in a parallel triple fusion fashion and adaptively selects features appropriate for objects of certain scales. Furthermore, we design a spatial interactive module (SIM) to preserve fine spatial detail through cross-spatial feature association. Extensive experiments prove that the proposed network significantly outperforms the state-of-the-art methods, in which we achieve 33.1 mAP and 56.5 AP50 on the VisDrone benchmark, 63.4 mAP and 94 AP50 on the NWPU VHR-10 benchmark. The source code will be released.
更多查看译文
关键词
Boundary-aware network,Spatial interaction,Attentional feature parallel fusion,Small object detection
AI 理解论文
溯源树
样例
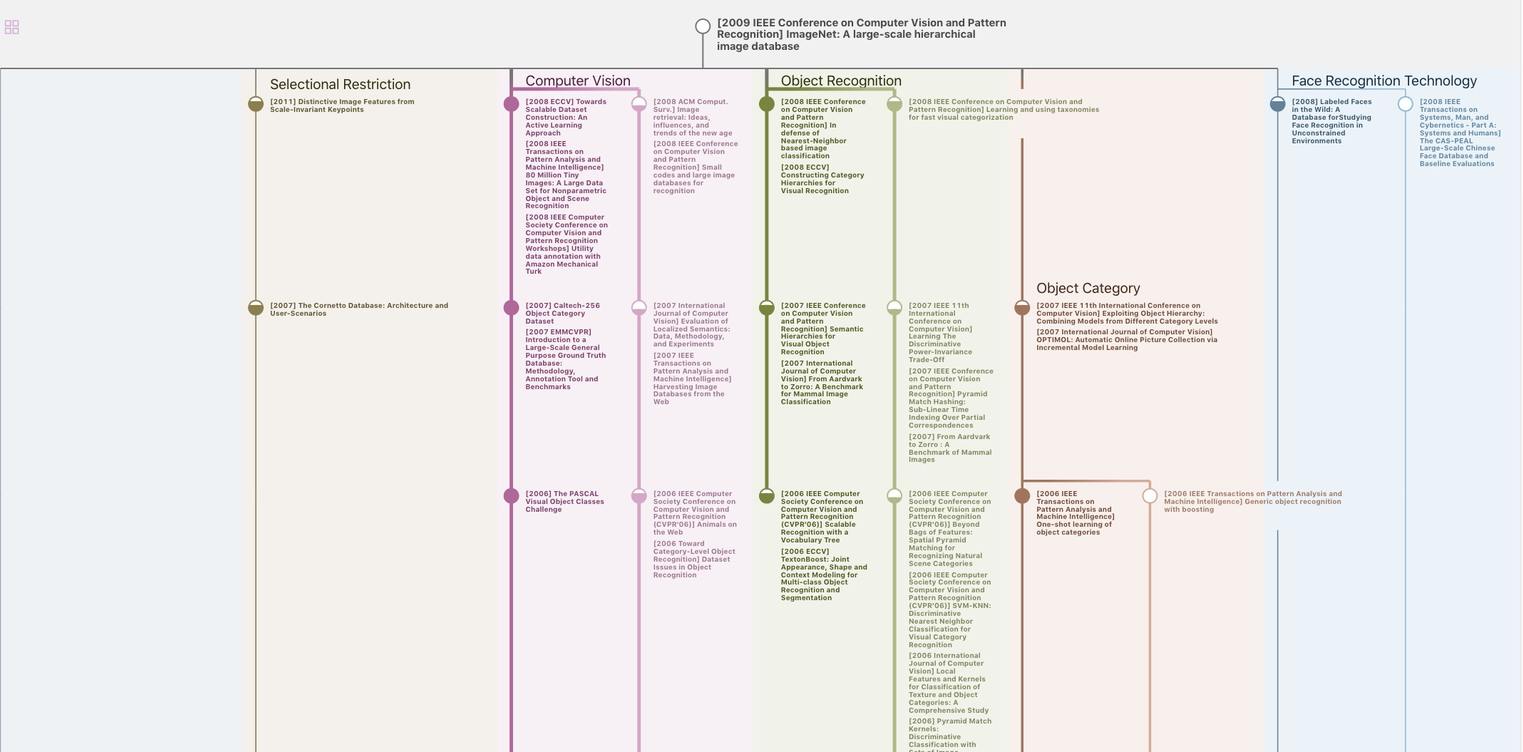
生成溯源树,研究论文发展脉络
Chat Paper
正在生成论文摘要