Machine Learning Based Model and Solution for Network Flow Attack Detection
2023 4th IEEE Global Conference for Advancement in Technology (GCAT)(2023)
摘要
Network attacks pose major risks to computer system and data security and integrity. The LuFlow22 dataset was used in this study to detect flow-based network threats. We use machine learning classification approaches, specifically the set of machine learning algorithms comprises Decision Trees, Random Forests, Support Vector Machines (SVM), and KNearest Neighbors (KNN) are all examples of machine learning algorithms, to assess their effectiveness in detecting and mitigating network assaults. The Decision Tree algorithm emerges as the greatest performer in terms of precision, regularly achieving greater accuracy in producing positive predictions. The results highlight the Decision Tree algorithm’s dependability and effectiveness in detecting flow-based network attacks. Furthermore, we address possible future uses of this method, such as its integration with real-time network intrusion detection systems to strengthen network security and protect against emerging threats. The study illustrates the importance of using machine learning in network security and provides the groundwork for future more powerful and adaptive intrusion detection systems.
更多查看译文
关键词
Flow Based Network Attack,Decision Tree,Malicious,K-Nearest Neighbors,Security,Machine Learning,Network Treats
AI 理解论文
溯源树
样例
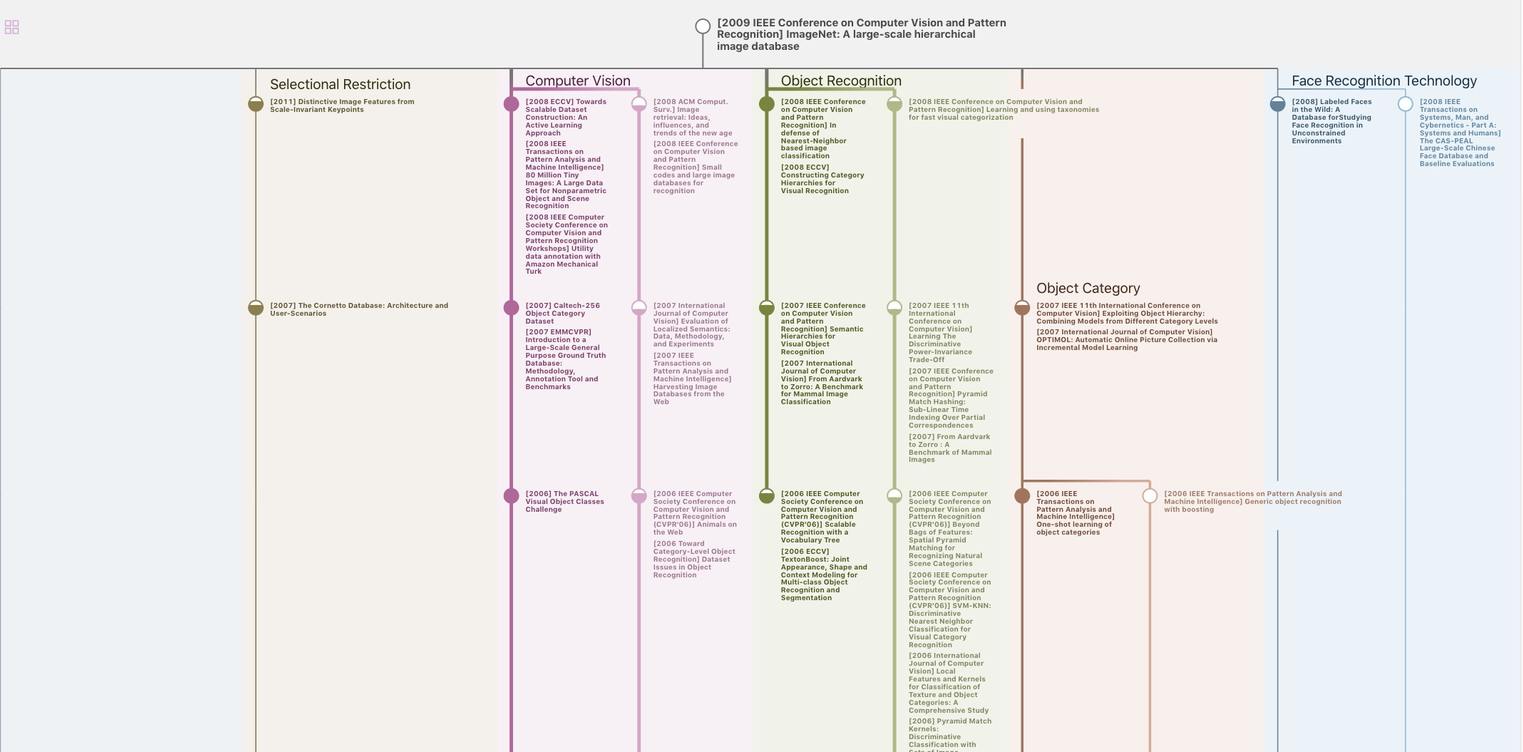
生成溯源树,研究论文发展脉络
Chat Paper
正在生成论文摘要