GDP: Group-Based Differential Privacy Framework for Secure Process Mining in the Internet of Medical Things.
2023 International Conference on Electrical Engineering and Informatics (ICEEI)(2023)
摘要
With the widespread adoption of Internet of Things (IoTs) technology in the healthcare domain, the Internet of Medical Things (IoMTs) has emerged as a promising paradigm for improving patient care and healthcare outcomes. IoMT generates vast amounts of data, including process logs. Therefore, Process Mining (PM) is a potential approach that can provide valuable insights into healthcare processes for several optimizations. The analysis of such sensitive data through PM raises privacy and security concerns. However, using PM for specific data allows IoMTs to secure the sensitive data. Therefore, this paper aims to evaluate existing PM algorithms, for behavior after adding noise in data without affecting PM results. Differential Privacy (DP) is a widely recognized privacy-preserving technique that provides strong privacy guarantees by injecting random noise into data. Therefore, this research presents a framework, called Group-based DP (GDP) for PM to protect the privacy of healthcare data in specific columns which are neither activity nor class ID. This study includes evaluation of prominent PM algorithms, including, Alpha algorithm, Heuristics algorithm, inductive algorithm, correlation mining algorithm and footprints algorithm. Furthermore, we suggested pertinent advanced research directions concerning real-world requirements.
更多查看译文
关键词
IoMTs,Differential Privacy,Security,Process Mining,Healthcare
AI 理解论文
溯源树
样例
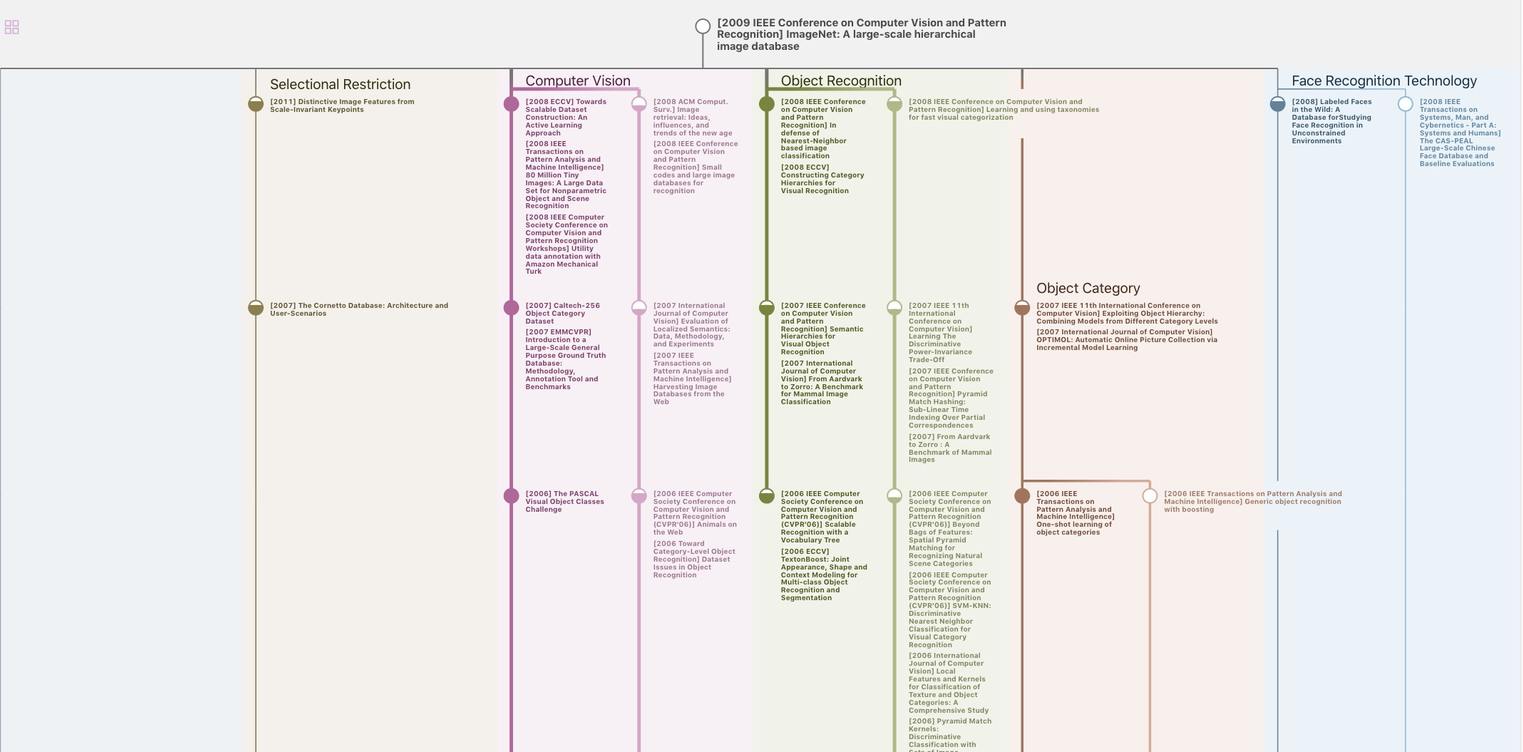
生成溯源树,研究论文发展脉络
Chat Paper
正在生成论文摘要