A Novel Causal Inference-Guided Feature Enhancement Framework for PolSAR Image Classification
IEEE TRANSACTIONS ON GEOSCIENCE AND REMOTE SENSING(2024)
摘要
In recent years, there has been a prominent focus on enhancing the quality of features derived from convolutional neural networks (CNNs) within the field of polarimetric synthetic aperture radar (PolSAR) image classification. Targeting this challenge, this article first visualizes the lack of discriminability and generalizability in CNN features through several empirical observations. Subsequently, we explain why these problems arise from a causal perspective, accomplished by means of a structural causal model (SCM) constructed according to the training and testing process of CNNs. This SCM facilitates the identification of variables that affect the quality of PolSAR image feature learning, as well as an intervention on those variables using backdoor adjustment. Building upon this groundwork, a novel causal inference-guided feature enhancement framework is constructed. It can be seamlessly integrated into any CNN-based PolSAR image classifier in a plug-and-play manner, enabling the enhanced classifier to filter out interference information and prevent model overfitting. These two aspects bring better feature discriminability and generalizability, respectively, leading to improved classification performance. Experimental results on four widely-used PolSAR image datasets demonstrate the effectiveness of our proposed framework. We integrate it into several mainstream methods in the field and show that the accuracy of the enhanced classifier is improved compared to the original model.
更多查看译文
关键词
Feature extraction,Training,Image classification,Convolutional neural networks,Remote sensing,Convolution,Synthetic aperture radar,Causal inference,deep learning,feature enhancement,image classification,polarimetric synthetic aperture radar (PolSAR)
AI 理解论文
溯源树
样例
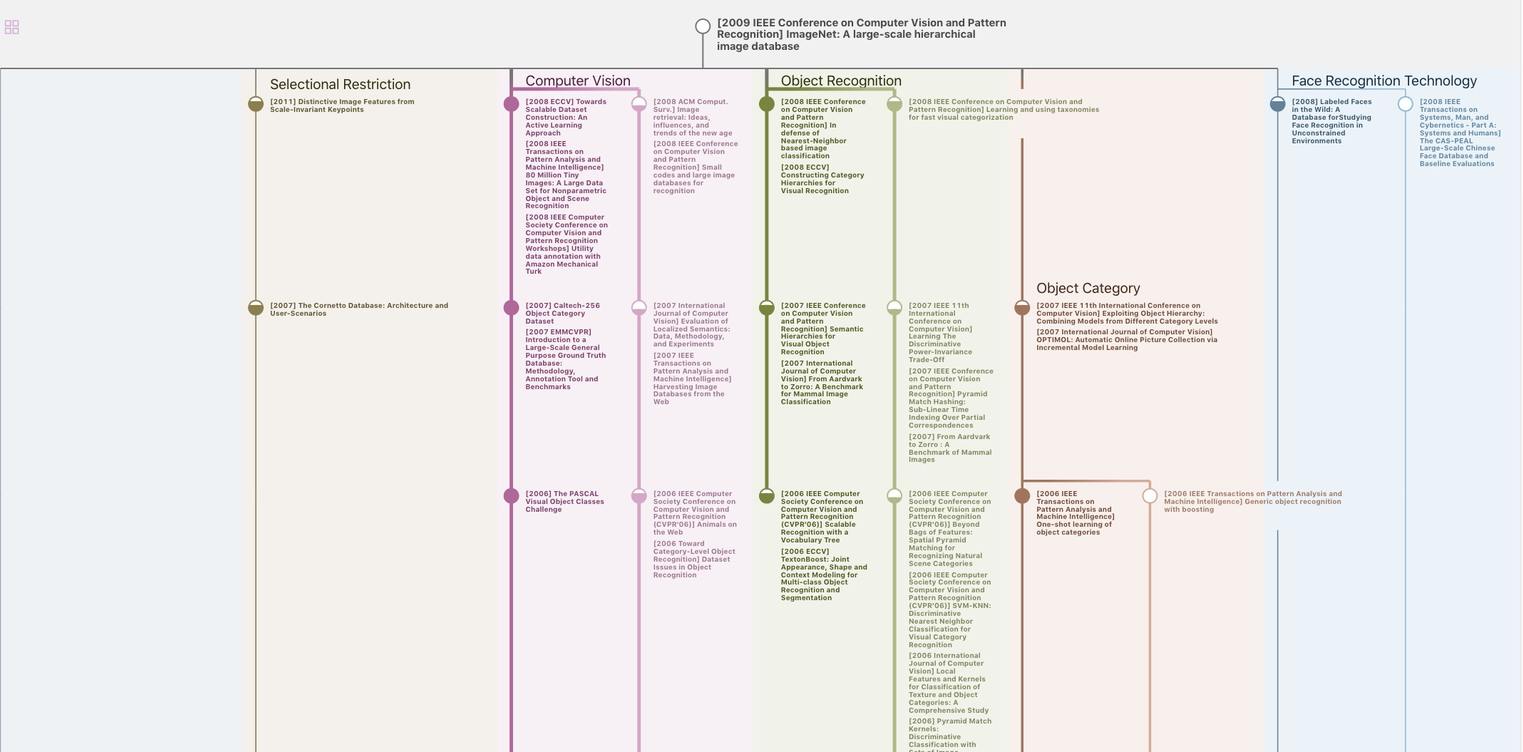
生成溯源树,研究论文发展脉络
Chat Paper
正在生成论文摘要