Regenerating Networked Systems’ Monitoring Traces Using Neural Networks
Journal of Network and Systems Management(2024)
摘要
Monitoring main entities in distributed systems is important for research, development, and innovation activities involving those systems (offline analysis, simulated evaluation, etc.). In many of them, monitoring can only be done via periodic and indirect sampling of the entities online (e.g., obtaining peer lists from trackers in BitTorrent). A problem with such approach is that the monitoring system may fail to see one or more online entities when samples are captured. Avoiding such failures by increasing monitoring resources (i.e., using fault tolerance techniques) may be challenging due to restrictions imposed by observed entities (e.g., a minimum interval between monitoring requests), if not impossible (e.g., monitoring again the behavior of a system during the 2023 Women’s World Cup). To cope with such failures after the monitoring has occurred, previous investigations have applied statistical methods to identify and correct failures. In this paper, we move in that direction by investigating artificial neural networks as a means to regenerate monitoring traces collected via sampling. We propose a deep learning based algorithm and three neural network topologies for correcting traces. We provide evidence that precision, accuracy, and recall can be substantially improved compared to existing statistical methods. The proposed method has potential to pave the road for improving the quality of monitoring traces of large distributed systems using neural networks, for increasing the quality of previously taken monitoring traces, and for delivering more resource-efficient distributed system monitoring.
更多查看译文
关键词
Monitoring of distributed systems,Snapshot of online users,Snapshot failures,Data imputation,Deep learning
AI 理解论文
溯源树
样例
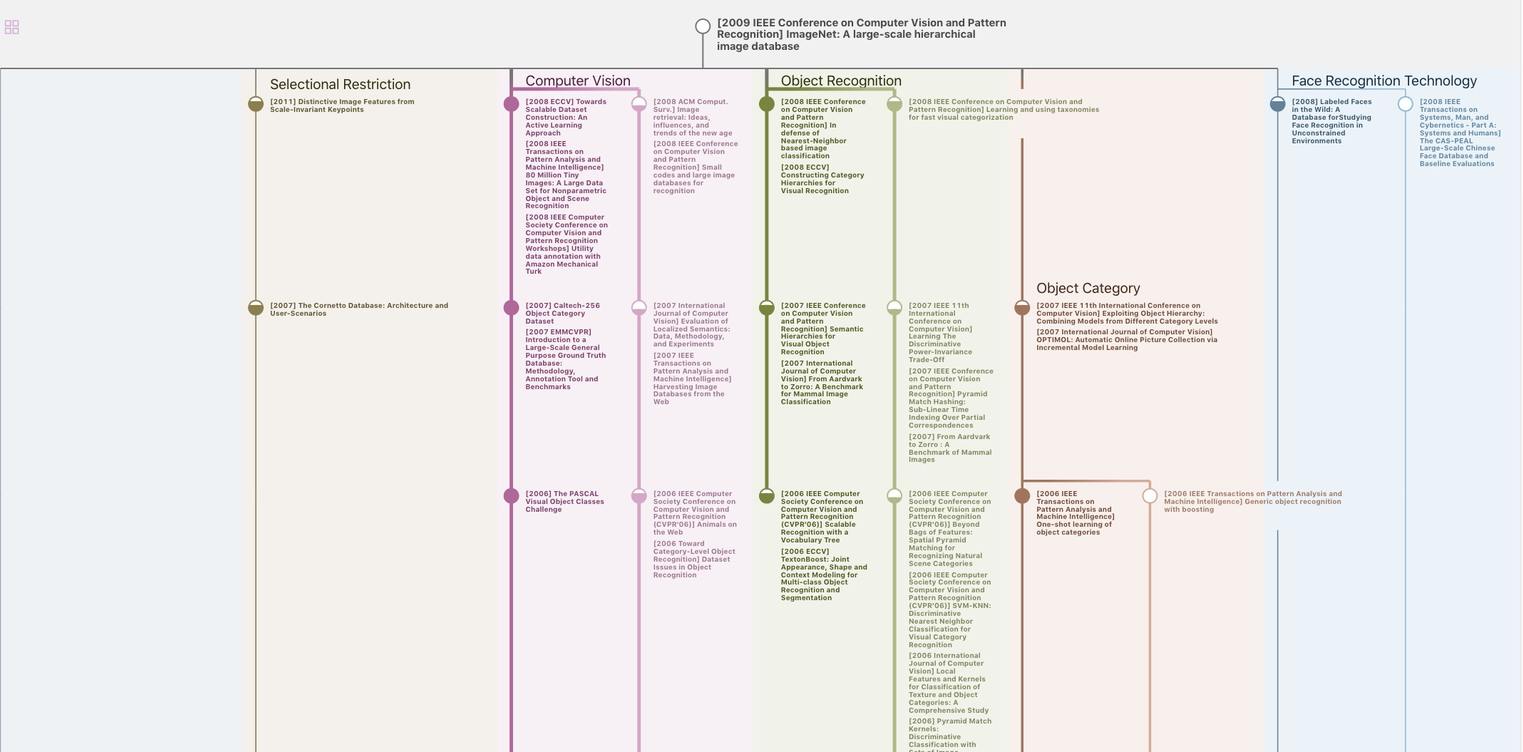
生成溯源树,研究论文发展脉络
Chat Paper
正在生成论文摘要