Modeling different effects of user and product attributes on review sentiment classification
Applied Intelligence(2024)
摘要
As a fundamental task, review sentiment classification aims to predict a user’s overall sentiment in a review about a product. Recent studies have proven critical effects of user and product attributes on this task. However, they usually adopt the same way to incorporate these two attributes, which does not fully consider their different effects and thus is not capable of leveraging them effectively. To address this issue, we propose a simple and effective review sentiment classification model based on hierarchical attention network, where different effects of user and product attributes are respectively captured via fusion modules and attention mechanisms. We further propose a training framework based on mutual distillation to fully capture the individual effects of user and product attributes. Specifically, two auxiliary models with only the user or product attribute are introduced to benefit our model. During the joint training process, our model and the auxiliary models boost each other via mutual knowledge distillation in an iterative manner. On the benchmark IMDB and Yelp datasets, our model significantly outperforms competitive baselines with a 1.6% improvement in average accuracy. When BERT embeddings are used as inputs, our model still performs much better than recent BERT-enhanced baselines.
更多查看译文
关键词
Sentiment classification,User and product attributes,Knowledge distillation
AI 理解论文
溯源树
样例
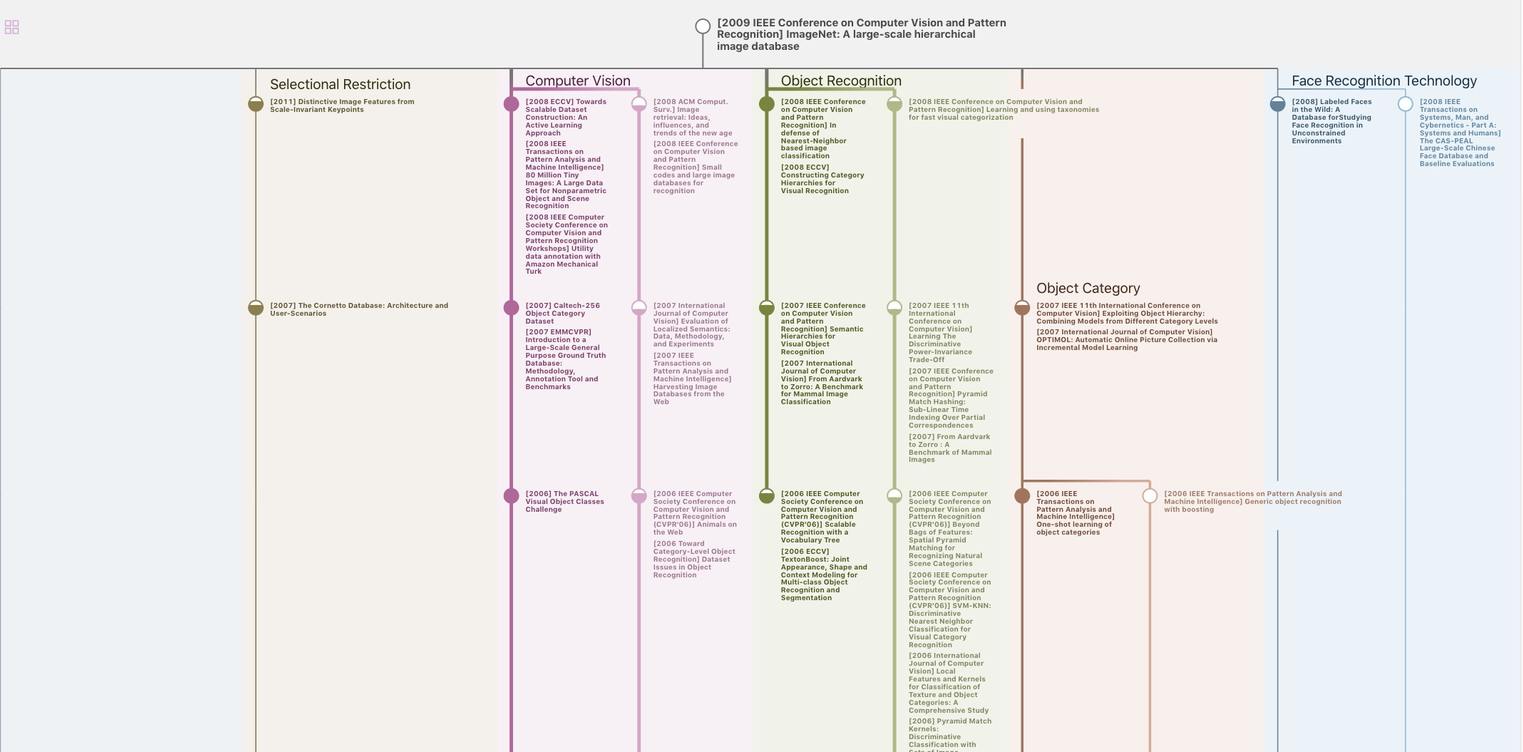
生成溯源树,研究论文发展脉络
Chat Paper
正在生成论文摘要