A Two-Phase ResNet for Object Detection in Aerial Images.
2023 IEEE International Conference on Imaging Systems and Techniques (IST)(2023)
摘要
Unmanned Aerial Vehicles (UAVs) have experienced great growth in real-world applications (surveillance, smart farming, etc.). One of the most popular features of UAVs in combination with optical sensors is real-time object detection. In this regard, Deep Learning algorithms have been proven to be the best solution to such kinds of problems. In this work, a modified Retina Network (ResNet) is proposed in which a larger number of Convolutional Blocks is introduced and features are combined from different levels of the Neural Network. The extra convolution block is a mirror of the FPN network; therefore, the new model is called Two-Phase ResNet. Since the goal is also to provide a sensitivity study, the classical ResNet and the Two-Phase ResNet are trained and tested using the Stanford Drone Dataset. The Two-Phase ResNet model achieves an accuracy score 6% higher than the baseline model (ResNet), and it seems to outperform the original model for each metric (Accuracy, Precision, Recall, and F1 score). Finally, both the original and the modified ResNet, are compared with other well-known object detectors. The proposed architecture seems to achieve similar or better performance compared to almost each object detector from the literature in terms of mean Average Precision. The Two-Phase ResNet can potentially be used to detect tiny objects in applications where accuracy is a critical factor.
更多查看译文
关键词
aerial images,object detection,ResNet,deep learning,remote sensing
AI 理解论文
溯源树
样例
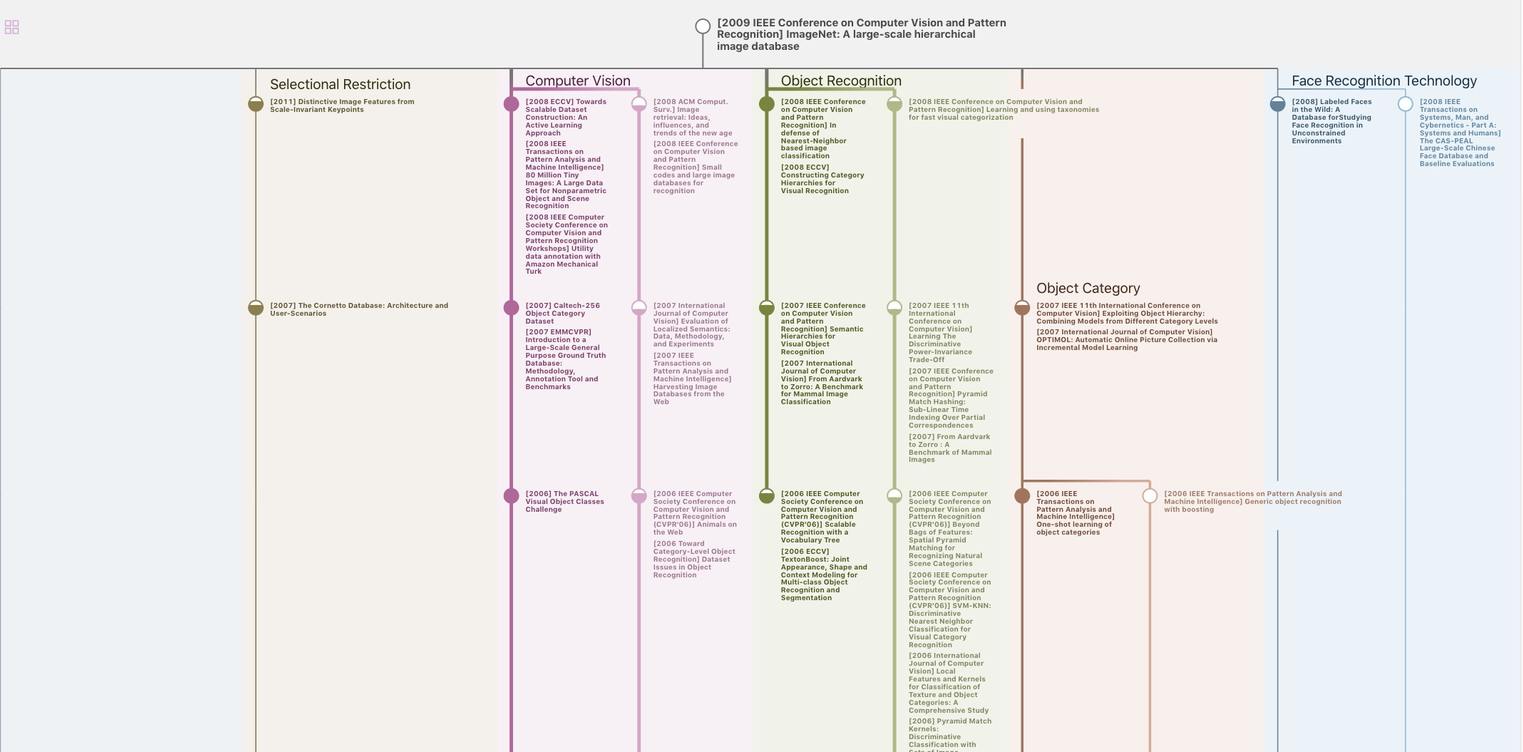
生成溯源树,研究论文发展脉络
Chat Paper
正在生成论文摘要