Wildforest Fire Detection with ShRe-Xception Network on Aerial Optical and Infrared Images.
2023 IEEE International Conference on Imaging Systems and Techniques (IST)(2023)
摘要
It is commonly accepted that wildforest fires are one of the major natural disasters worldwide. This destruction has a crucial impact to fire fighters and civilian lives, animal extinction, and forest degradation. This work focuses on preventing potentially extensive disasters by developing a detection system that can classify images of forests in "Fire" and "non-Fire" cases with input from both RGB and Infrared (IR) cameras. Our design uses both an existing small – architecture of Xception network (small-arch Xception) and the proposed Short Recursive (ShRe)-Xception. We first use transfer learning on the small-arch Xception network with input from either RGB or IR images. We also train the proposed ShRe-Xception network by using all images from the very beginning. Datasets used for training and testing purposes contain "Fire" and "non-Fire" images of forests that are captured from UAVs and uploaded in IEEE portal. When testing the above models, the estimated accuracy was RGB: 77.72% / IR: 96.83% after transfer learning on small-arch Xception, RGB: 84.86% / IR: 29.18% when initially training the small-arch Xception, and RGB: 90.10% / IR: 99.31% after initially training the ShRe-Xception model. This makes the latter model the most accurate one for images of both spectrums in our experiments. The ShRe-Xception can potentially play a vital and efficient role on the real-time fire detection during aerial surveillance on wild-forests.
更多查看译文
关键词
Wildforest fires,remote sensing,neural networks,ShRe-Xception network
AI 理解论文
溯源树
样例
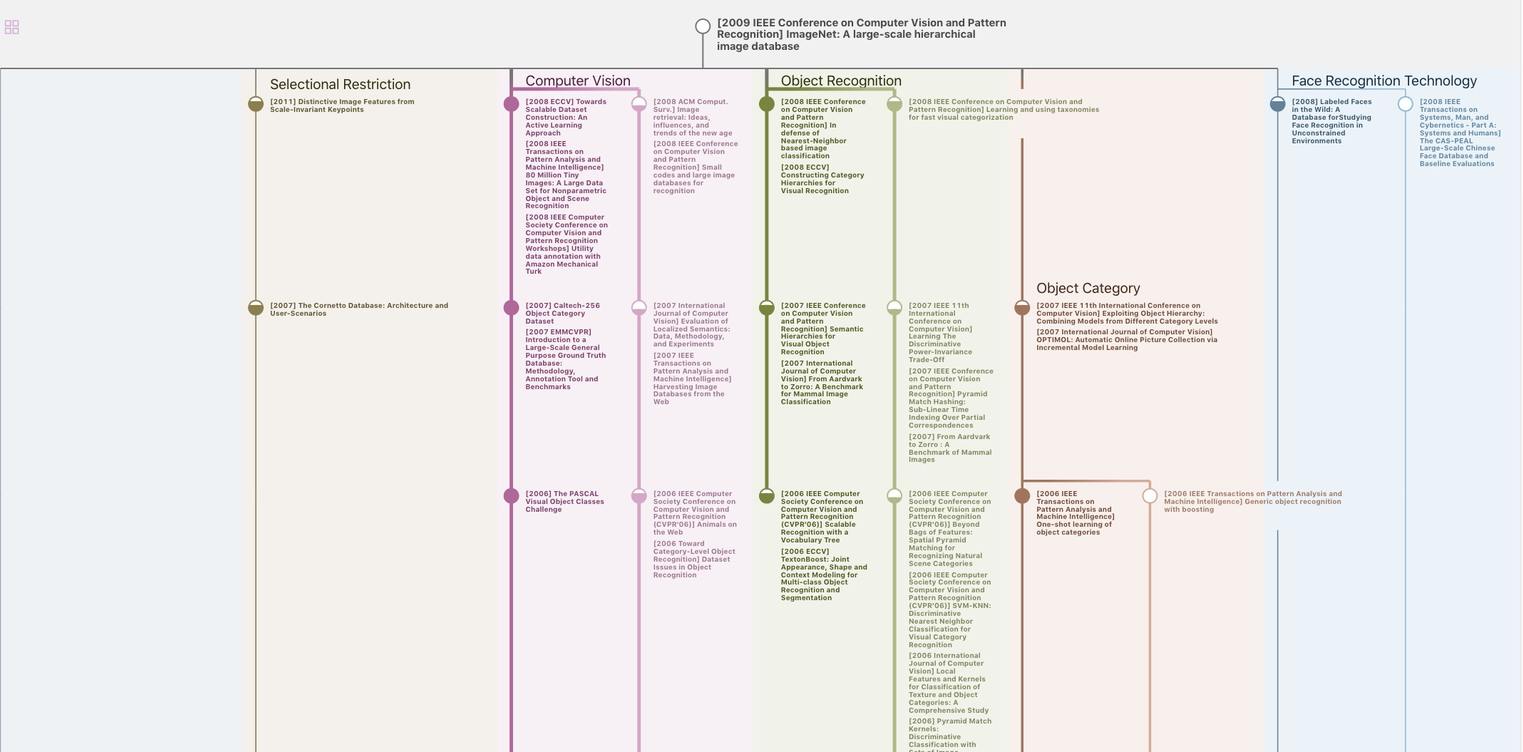
生成溯源树,研究论文发展脉络
Chat Paper
正在生成论文摘要