Distilling Autoregressive Models to Obtain High-Performance Non-autoregressive Solvers for Vehicle Routing Problems with Faster Inference Speed
AAAI 2024(2024)
摘要
Neural construction models have shown promising performance for Vehicle Routing Problems (VRPs) by adopting either the Autoregressive (AR) or Non-Autoregressive (NAR) learning approach. While AR models produce high-quality solutions, they generally have a high inference latency due to their sequential generation nature. Conversely, NAR models generate solutions in parallel with a low inference latency but generally exhibit inferior performance. In this paper, we propose a generic Guided Non-Autoregressive Knowledge Distillation (GNARKD) method to obtain high-performance NAR models having a low inference latency. GNARKD removes the constraint of sequential generation in AR models while preserving the learned pivotal components in the network architecture to obtain the corresponding NAR models through knowledge distillation. We evaluate GNARKD by applying it to three widely adopted AR models to obtain NAR VRP solvers for both synthesized and real-world instances. The experimental results demonstrate that GNARKD significantly reduces the inference time (4-5 times faster) with acceptable performance drop (2-3%). To the best of our knowledge, this study is first-of-its-kind to obtain NAR VRP solvers from AR ones through knowledge distillation.
更多查看译文
关键词
PRS: Learning for Planning and Scheduling
AI 理解论文
溯源树
样例
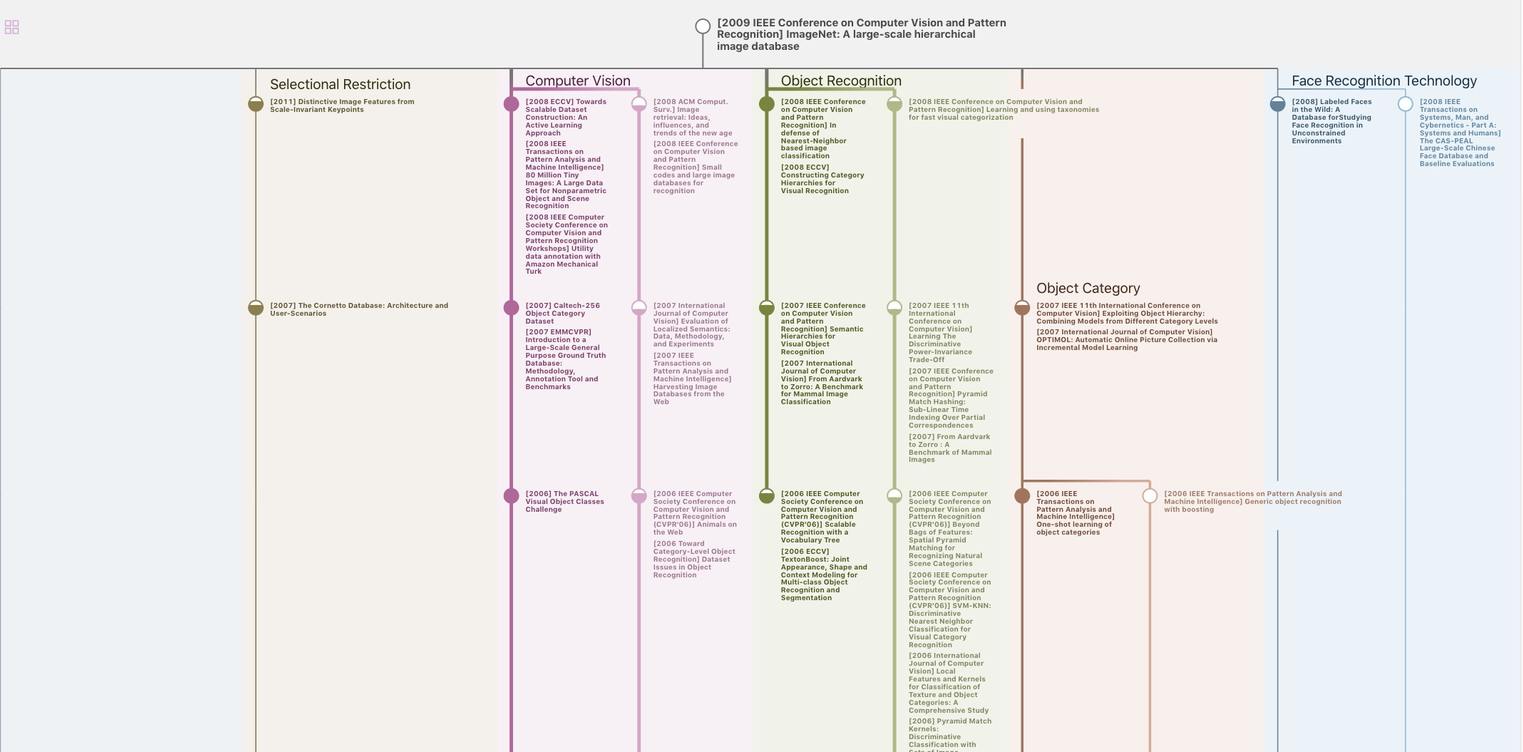
生成溯源树,研究论文发展脉络
Chat Paper
正在生成论文摘要