A multi-scale hierarchical node graph neural network for few-shot learning
Multimedia Tools and Applications(2023)
摘要
The graph neural network model has shown strong classification performance in few-shot learning (FSL) tasks in recent years. However, the existing methods have mainly participated in model training by extracting the single features of the samples as nodes. This method of constructing nodes ignores the multi-scale information contained in the samples to a certain extent and limits the expressiveness of node features. Therefore, we propose a multi-scale hierarchical node graph neural network (MsHN-GNN), which constructs hierarchical nodes bearing multi-scale information by deeply extracting structural features, global features, and local features of samples and enhances the representation ability of node features. At the same time, MsHN-GNN sorts the hierarchical relationship among sub-nodes through the block alignment module so that sub-nodes correspond one-to-one according to their own content attributes, which lays a foundation for updating multi-scale hierarchical nodes. In addition, we design a unique graph network update method according to the characteristics of hierarchical nodes. Through the multi-scale hierarchical node aggregation and edge aggregation modules, we realize the hierarchical information interaction among multiple sub-nodes so that the updated nodes and edge features have stronger information expression ability. Finally, to verify the effectiveness of the model, we conduct extensive experiments on miniImageNet, TiredImageNet, and CUB-200-2011 datasets. The experimental results show that MsHN-GNN outperforms other advanced few-shot learning methods with a graph structure in classification performance. In the above three datasets, the 5-way 1-shot experimental results achieved 68.32%, 72.56%, and 76.21%, respectively.
更多查看译文
关键词
Few-shot learning,Graph neural network,Multi-scale hierarchical node,Block alignment,Aggregation module
AI 理解论文
溯源树
样例
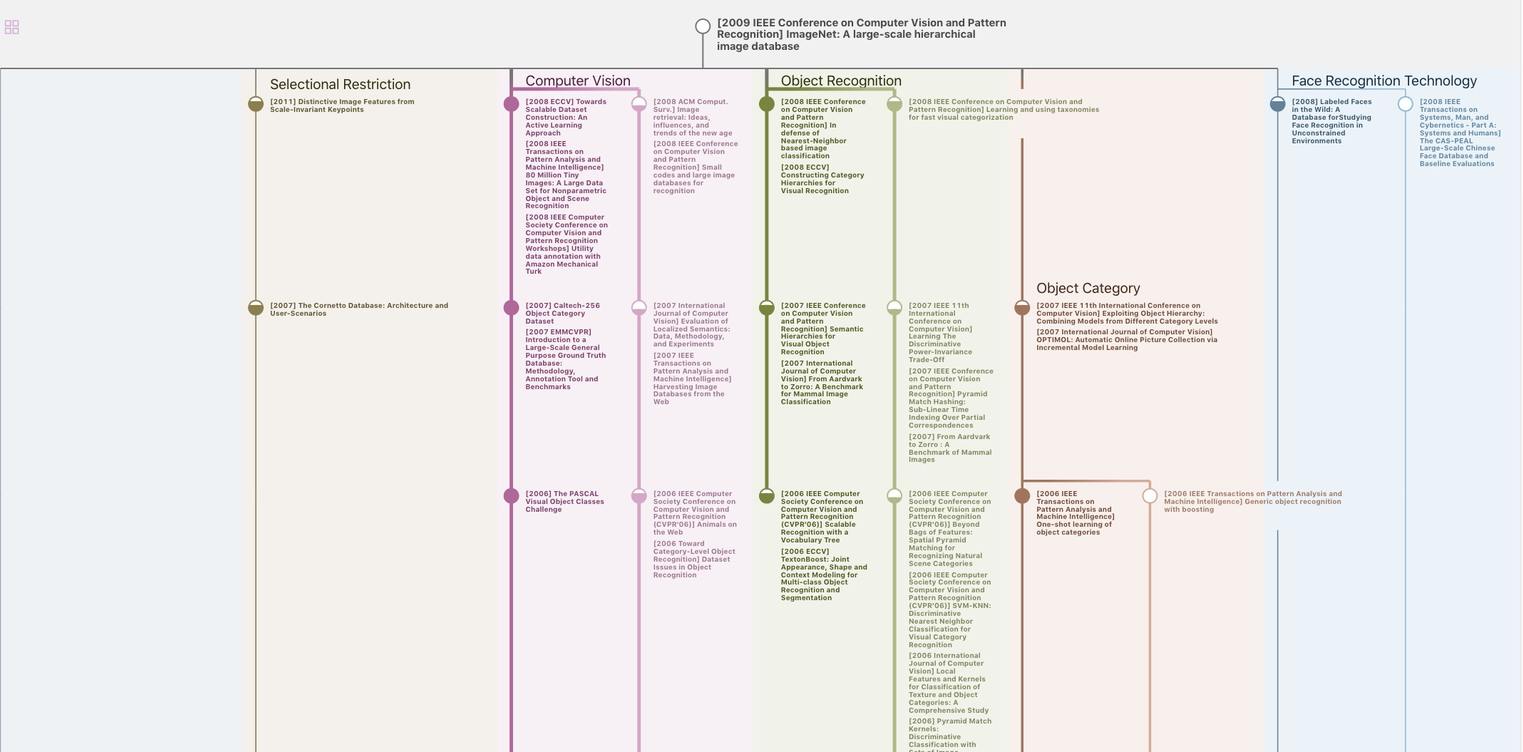
生成溯源树,研究论文发展脉络
Chat Paper
正在生成论文摘要