Local motion feature extraction and spatiotemporal attention mechanism for action recognition
The Visual Computer(2023)
摘要
Video action recognition faces the important and challenging problem of spatiotemporal relationship modeling. In order to solve this issue, current methods typically rely on 2D or 3D CNN operations to model local spatiotemporal dependencies at fixed scales. However, most of these models fail to emphasize the keyframes and action-sensitive regions of the input video, resulting in poor performance. In this paper, an action recognition network with local motion feature extraction and spatiotemporal attention mechanism is proposed. The proposed network consists of a motion capture (MC) module and a temporal attention (TA) and spatiotemporal attention (STA) module, which capture detailed motion features, and learns the contribution of each frame and each region to the action at the feature level, respectively. To evaluate our network, we construct a concrete water addition violation dataset (CWAVD), which can be used to identify water addition violations by construction site workers and improve construction management efficiency and quality. The proposed network achieves the state-of-the-art performance on three of the most challenging datasets, UCF101 (97.6%), HMDB51 (77.3%) and SSV2 (67.8%).
更多查看译文
关键词
Action recognition,Spatiotemporal attention,Convolution neural network,Abnormal behavior
AI 理解论文
溯源树
样例
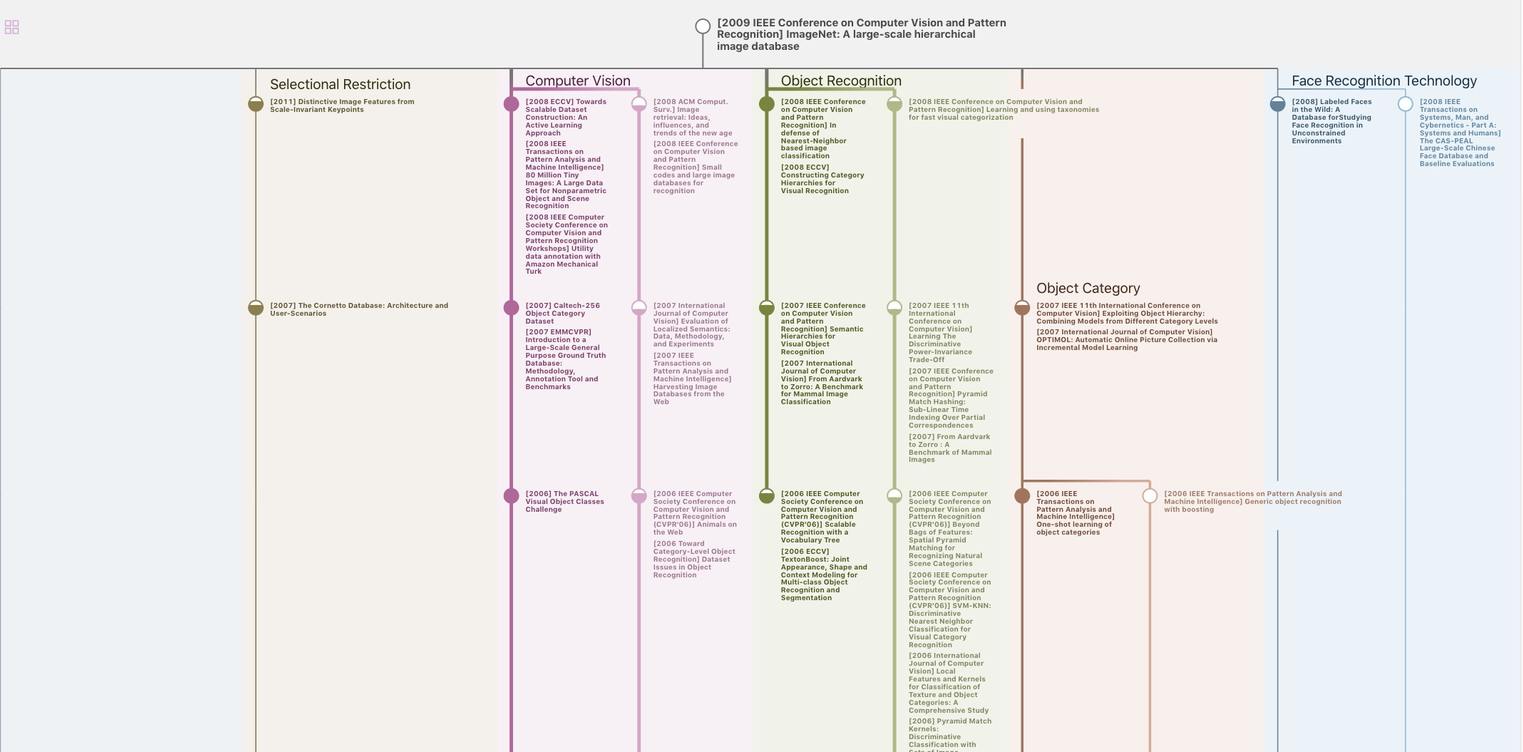
生成溯源树,研究论文发展脉络
Chat Paper
正在生成论文摘要