Privacy-Preserving Genomic Analysis via PSO-Driven Federated Learning on Blockchain
2023 3rd Intelligent Cybersecurity Conference (ICSC)(2023)
摘要
Federated learning, a distributed machine learning method, trains statistical models over remote devices or servers with local data, without exchanging data samples. It preserves patient data securely behind hospital firewalls, sharing only anonymous model parameters, enhancing privacy and security. This innovative approach exchanges only untraceable learned feature representations. This study introduces a new federated learning framework an innovative aggregation methods based on Particle Swarm Optimization (PSO) algorithm for breast cancer prognosis. To safeguard patient data privacy, a privacy mechanism is implemented at the user's end. By utilizing the federated learning model, they've addressed the data scarcity problem, leading to improved accuracy in breast cancer progno-sis. Federated learning, as mentioned earlier, protects personal data by sending model parameters to agents instead of raw data to a central node, keeping data localized. However, the risk of malicious nodes injecting fake data into the global model is a concern. To address this, we need a verification mechanism to authenticate senders and their training data while preserving data privacy. We use zero-knowledge proof (ZKP) for verification without exposing raw data for this part. At the outset of the federated learning process, each client computes the Merkle tree root hash of their local training data and submits it to the federated learning contract for participation. In subsequent training rounds, the client's compiler checks the integrity of their data by comparing the Merkle tree root hash to the initial one. If they match, a trace file is generated and sent for proof generation, verification, fact creation, and registration. Leveraging the TCGAbiolinks package, we conducted experiments demonstrating the effectiveness of our approach with real data. Learner clients enhance their local models using a PSO algorithm, achieving remarkable results in binary classification tasks with 400 features while preserving patient data privacy via ZKP integration. Our framework reaches 97% accuracy with eight clients, compared to a comparable system's 82%, highlighting its practical applicability and efficiency in real-world scenarios, particularly when dealing with larger numbers of participants.
更多查看译文
关键词
Federated learning,blockchain,privacy,security,zero-knowledge proof,genomic data,decentralized learning,deep learning,machine learning
AI 理解论文
溯源树
样例
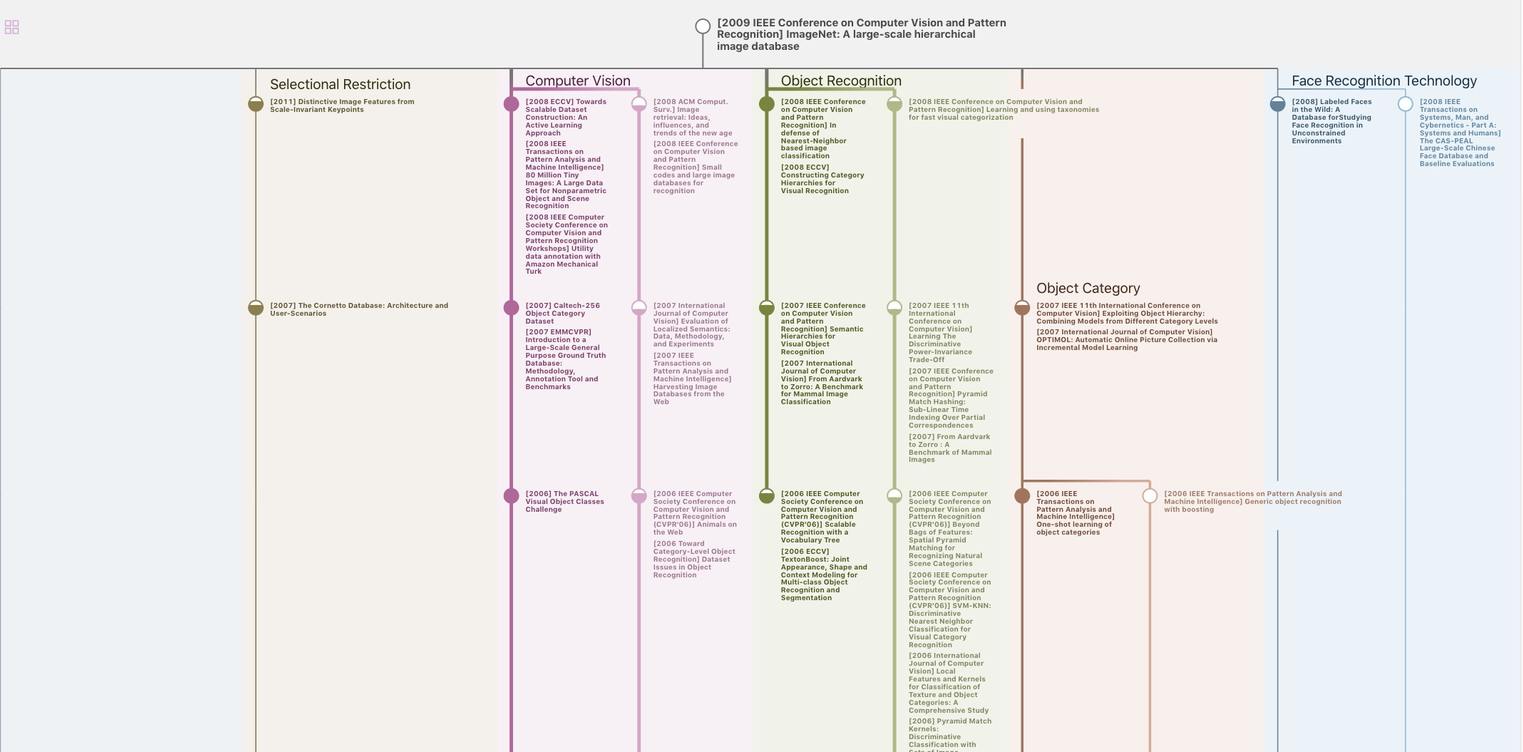
生成溯源树,研究论文发展脉络
Chat Paper
正在生成论文摘要