Comparative Analysis of ResNet101, InceptionV3, and InceptionResnetV2 Architectures for Cervical Vertebrae Maturation Stage Classification.
2023 International Conference on Electrical Engineering and Informatics (ICEEI)(2023)
摘要
The classification of cervical vertebrae maturity (CVM) plays a crucial role in orthodontic diagnosis and treatment planning. This study aims to evaluate the performances of bone maturity classification using three convolutional neural network architectures. The dataset used in this study utilizes the CVM-900 dataset which contains labeled cervical vertebrae x-ray images in 6 levels of bone maturation. Preprocessing was conducted by ROI cropping which results in three datasets: Crop C2-C4, Crop C2-C6, and uncrop. Then, to decrease the possibility of overfitting, the datasets are augmented with random translation and rotation. This work compares three pre-trained networks: ResNet-101, InceptionResNetV2, and InceptionV3. Each network has unique characteristics to improve performance in deep learning. Visual explanations of CVM stage classification from each model are conducted through Grad-CAM which leverages the gradient information to the target classes. We found that InceptionResNetV2 yields the highest accuracy across models and ROIs. It is shown that ROI affects model's performance. However, due to overfitting and the inability of our model to infer multiscale features, the increase in accuracy is not as significant.
更多查看译文
关键词
Resnet101,InceptionV3,InceptionResnetV2,Cervical Vertebrae Maturity (CVM)
AI 理解论文
溯源树
样例
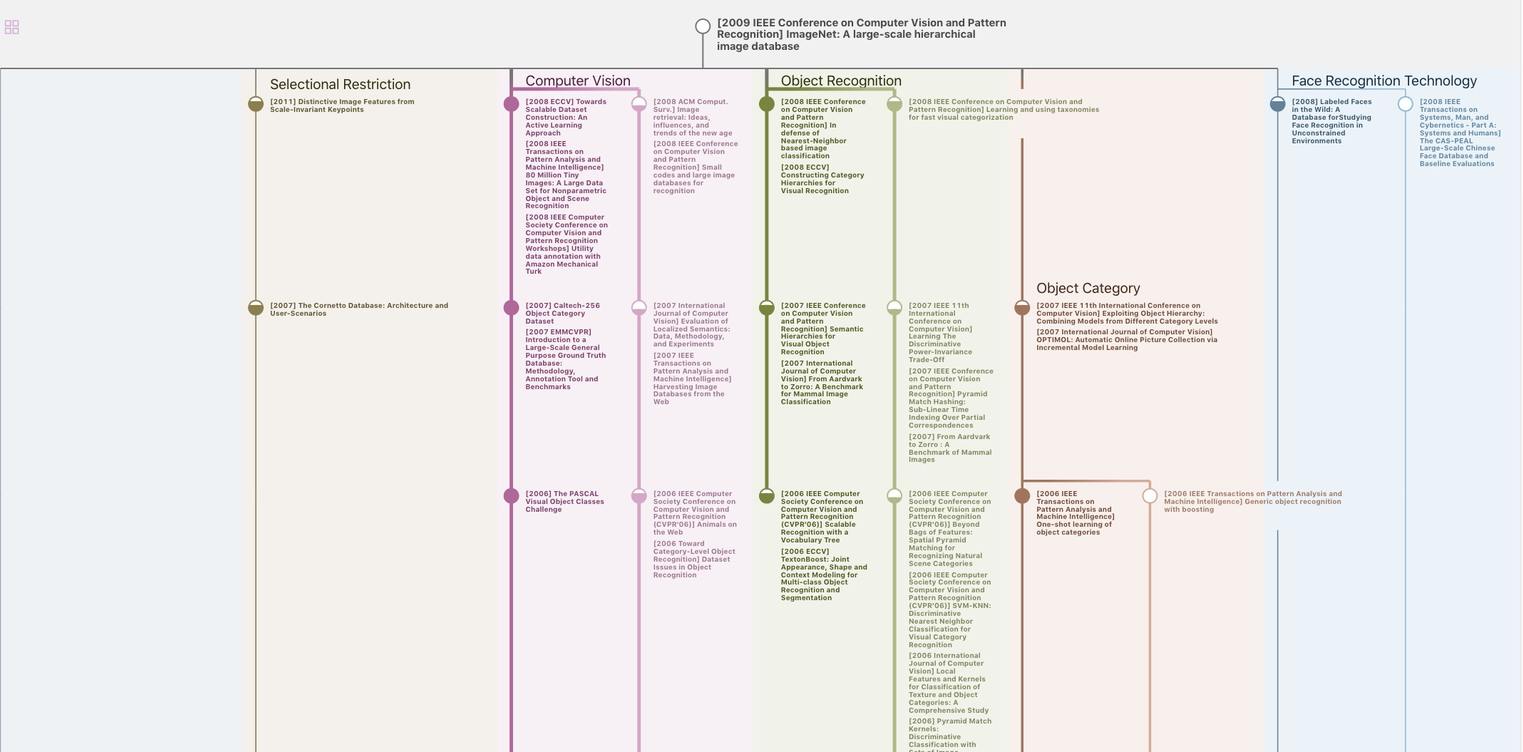
生成溯源树,研究论文发展脉络
Chat Paper
正在生成论文摘要