CNN-based retrieval of x-ray phase-contrast images from experimental data
2023 IEEE Nuclear Science Symposium, Medical Imaging Conference and International Symposium on Room-Temperature Semiconductor Detectors (NSS MIC RTSD)(2023)
摘要
Speckle-based x-ray phase-contrast imaging (XPCI) offers enhanced sensitivity towards weakly-attenuating materials – such as human tissue – and overcomes limitations of typical phase-contrast imaging systems due to less stringent requirements for setup geometry, coherence, and propagation distances. However, obtaining high-quality phase-contrast images requires accurate tracking of the near-field speckles generated by a random diffuser. A recently proposed speckle tracking method, convolutional-neural-network-based analysis (CADE), offers superior tracking performance, reduced computational times, and robustness to noise when compared to previous state-of-the-art methods but has only been validated on simulated displacements. In this study, we validated CADE on a simulated sine wave sample and experimental XPCI images of a plastic ball. CADE achieved good accuracy in displacement estimation with a simulated sample and further successfully extracted phase contrast signals from experimental imaging data. This brings the implementation of CADE in XPCI one step closer, broadening the potential of XPCI by introducing a novel, improved speckle tracking method.
更多查看译文
AI 理解论文
溯源树
样例
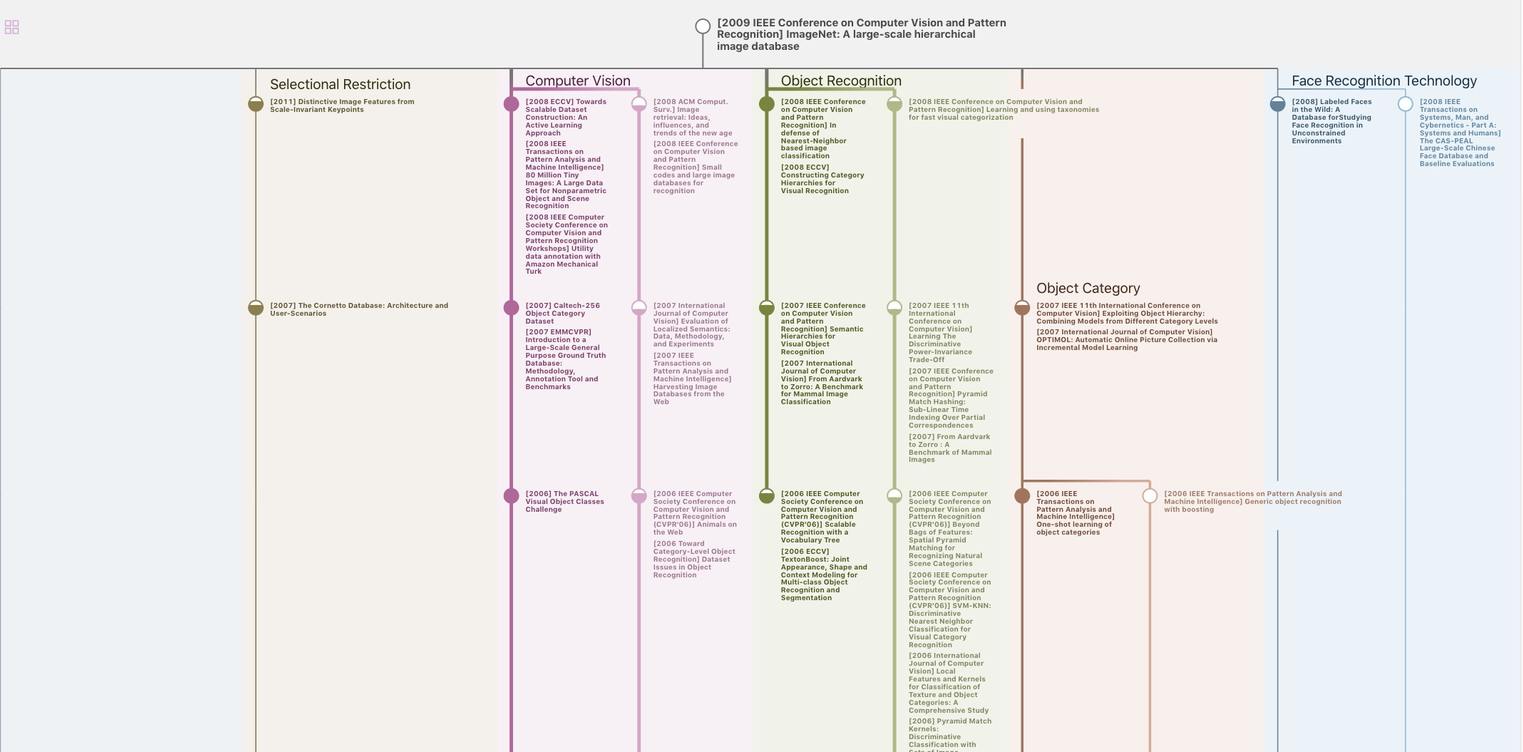
生成溯源树,研究论文发展脉络
Chat Paper
正在生成论文摘要